Image Colorization vs
AI Image Generation Comparing Free Tools in 2024
Image Colorization vs
AI Image Generation Comparing Free Tools in 2024 - Free AI Image Colorization Tools in 2024
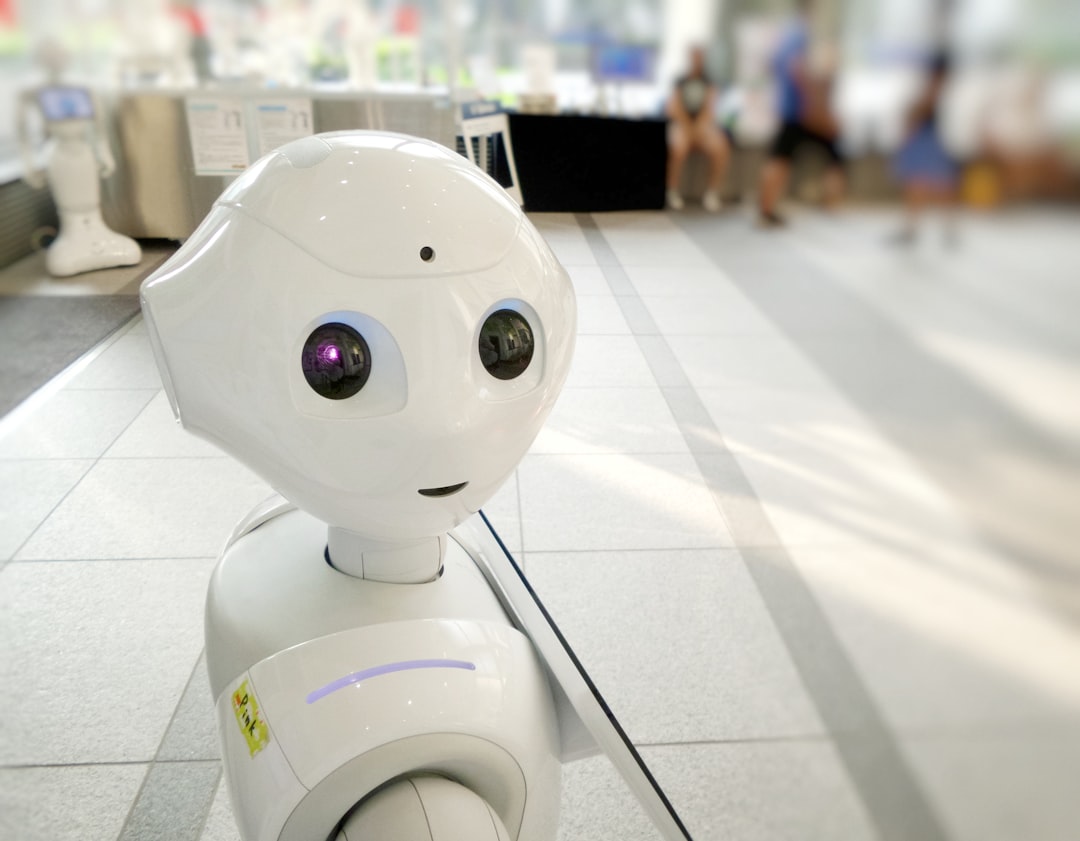
The availability of free AI image colorization tools has expanded significantly in 2024, offering a range of options for anyone wanting to breathe life into old black and white photos. CapCut Photo Colorizer has gained popularity for its straightforward approach and the high quality of its colorized outputs. ColouriseSG offers a unique appeal with its ability to inject vibrant colors into old photos, making them appear revitalized. YouCam AI, a mobile-friendly option, stands out for its colorization and broader image enhancement features. Palettefm has earned recognition for its strong performance, suggesting that the underlying algorithms are becoming increasingly adept at understanding the nuances of shades, patterns and textures within a picture. While the quality of these tools continues to improve, their emergence highlights a growing interest in utilizing AI not just to generate entirely new images, but also to restore and enhance existing ones in meaningful ways.
In 2024, a variety of free AI image colorization tools have emerged, many relying on sophisticated neural network architectures like convolutional neural networks (CNNs). These networks excel at recognizing patterns within grayscale images, enabling them to predict color in a manner that mirrors human color perception.
Some tools incorporate Generative Adversarial Networks (GANs) for even more realistic outputs. GANs utilize a two-part system: one network generates the colorized image, and another evaluates its authenticity against a set of criteria. This feedback loop contributes to more contextually sound and natural-looking results.
It's interesting to observe that the colorization style can vary widely depending on the training datasets used by these AI models. This implies that a single grayscale image can be interpreted and colorized in many distinct ways, reflecting the artistic leanings embedded within the model's training data.
Several free colorization platforms have introduced adjustable settings, allowing users to fine-tune elements like color saturation and hue. This gives a greater degree of creative control to the user, whether it's an engineer looking for a specific outcome or a hobbyist aiming for a certain aesthetic.
Recent advances in machine learning have resulted in significantly faster image processing times. Some tools can now colorize an image within seconds, a stark contrast to the longer processing times of earlier models.
The training datasets for these newer AI models are far more extensive than their predecessors, often comprised of millions of images. This increased dataset diversity has a clear impact on the quality and versatility of the resulting colorizations.
Open-source platforms are gaining ground within the AI image colorization community. This trend provides not only access to free tools but also opportunities for engineers to improve and adapt algorithms collaboratively, fostering innovation within the field.
Some of these tools integrate edge detection techniques to identify key features in a grayscale image before applying color. This targeted approach allows colors to better align with object boundaries, leading to outputs that are more visually coherent.
Despite improvements, considerable quality differences persist among the free tools. Some lack advanced preprocessing techniques, leading to inconsistent results even for similar input images. It's important for users to be mindful of this variation in quality when choosing a tool.
The user experience surrounding AI colorization tools has also significantly advanced. Many free tools feature intuitive interfaces and integrated tutorials, allowing users of various technical backgrounds to comfortably experiment with the technology. This accessibility caters to both novice and more experienced users, furthering the potential of this technology.
Image Colorization vs
AI Image Generation Comparing Free Tools in 2024 - Popular Free AI Image Generation Platforms
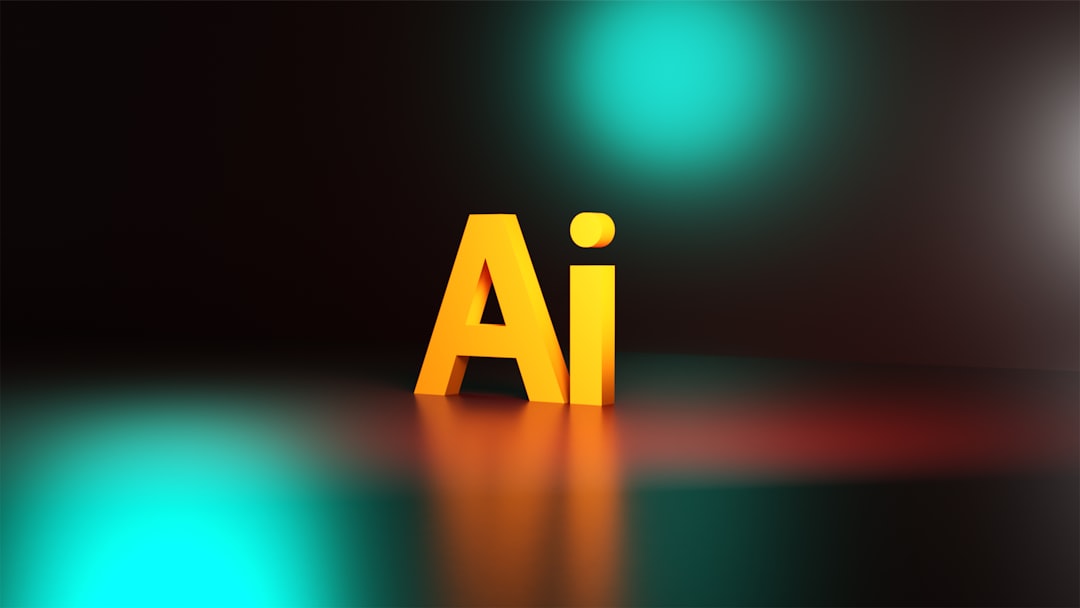
The realm of AI image generation has seen a surge in free platforms throughout 2024, offering a range of options for those interested in exploring this technology. Microsoft Designer's Image Creator has earned a reputation for being user-friendly and well-rounded, while OpenAI's DALL-E 3 is a favorite amongst casual users due to its ease of use and flexibility. Midjourney has established itself with its community-driven approach and intuitive web interface, but it's important to note the limited free trial it offers. Craiyon, formerly known as DALL-E Mini, remains a fully free option, though the output may not always meet the highest quality standards. Platforms like Canva and Piktochart have also incorporated AI image generation features into their free tiers, providing accessible entry points for casual users to experiment with the technology.
While these platforms democratize access to AI image creation, it's important to be aware of the varying levels of image quality and the specific strengths and weaknesses each one possesses. Some platforms produce impressively photorealistic images, while others struggle to generate consistent results. Users should weigh their needs and expectations before choosing a platform, ensuring a match between desired outcome and the platform's capabilities. This landscape is constantly changing, so it's worth staying informed about ongoing developments and the evolution of features within these AI-powered tools.
1. **Data's Impact on Output**: The way free AI image generation platforms are trained involves a complex relationship between dataset size and output quality. While larger datasets generally improve image quality, it's not a simple linear relationship. Increasing the data beyond a certain point provides diminishing returns in terms of performance gains.
2. **Gaming Techniques in AI Art**: Interestingly, some platforms borrow rendering techniques from the gaming industry to improve the realism of their generated images. These techniques, initially designed for creating immersive environments in video games, are now being repurposed to create more visually compelling AI images, adding depth and perspective.
3. **User Influence on AI Development**: A notable trend in free AI image generation is the rise of user-driven feedback mechanisms. These platforms are gathering data on which outputs users prefer, using this data to refine the algorithms. Essentially, users are directly influencing the development of these tools, pushing them in directions the community finds appealing.
4. **Copyright Concerns in Training Data**: The ethics of the images used to train these models is a complex topic. Some free platforms have encountered criticism for inadvertently incorporating copyrighted materials into their training data, raising issues about the legality and ethical implications of using AI to generate images based on these potentially unauthorized sources.
5. **Challenges of Subtle Color Replication**: One area where many AI image generation tools struggle is in accurately restoring the original colors in a photo. Limitations in the algorithmic sensitivity to shades often lead to color inaccuracies. These inaccuracies result in images that either exaggerate or subdue the colors, making the outcome differ from a user's intended result.
6. **Texture Replication**: Replicating textures in an image presents a unique challenge for AI image generation. Platforms that employ more advanced convolutional neural network approaches are generally better at capturing and replicating these textures, but simpler models struggle with this task. This distinction between basic and advanced tools is evident in the realism of the generated outputs.
7. **Pre-built Styles**: Many free tools offer preset styles for image generation that often mirror popular styles in digital art. While this can be helpful for experimenting, it also means that images can sometimes take on a dated or overly stylized appearance that might not be ideal for more professional use cases.
8. **Sensitivity to Image Irregularities**: Some free platforms are very sensitive to specific image features, or edge cases. For example, images with strong contrasts or unusual shapes can lead to outputs that are either exceptionally accurate or entirely inaccurate, depending on how those features were represented in the training data.
9. **Real-time Performance Bottlenecks**: Despite advances, free AI image generation platforms can still struggle with real-time processing when many users are active. This can cause delays in image generation due to limitations in server infrastructure, highlighting that there are limitations to how much processing power is available.
10. **Open-Source Collaboration**: Open-source AI platforms frequently inspire collaborations within their communities. These communities not only enhance algorithms but also promote transparency by enabling users to directly contribute to the improvement of tools that they use, a positive impact in the evolution of the field.
Image Colorization vs
AI Image Generation Comparing Free Tools in 2024 - Palette.fm The Leading AI Colorizer of 2024
Palette.fm has become a prominent AI colorization tool in 2024, largely due to its accuracy and ease of use. It swiftly transforms black and white photos into full-color images in a matter of milliseconds, making it attractive for a wide range of users. Its effectiveness stems from the use of sophisticated algorithms, including a unique one developed internally, which enables high-quality colorization results. Whether restoring old family photos or enhancing artwork, Palette.fm can deliver. It also sets itself apart by offering extensive customization possibilities, unlike many simpler tools. This blend of accessible design and advanced capabilities makes Palette.fm a leading option for AI-powered image colorization today.
Palette.fm has gained prominence as a leading AI colorization tool in 2024, largely due to its accuracy and ease of use. It quickly transforms black-and-white images into vibrant color versions, often within a few hundred milliseconds per image. This speed, coupled with its sophisticated algorithms, including a proprietary one, contributes to its efficiency and high-quality results.
Palette.fm's appeal extends beyond casual users, as its deep customization options have attracted professional colorists at large companies. Individuals restoring old family photos and artists adding color to their work can also benefit from its capabilities. The platform's intuitive design allows users to upload images, experiment with various color filters, and download high-resolution outputs—all without requiring an account.
Created by Emil Wallner, a Swedish machine-learning researcher, Palette.fm strikes a balance between simplicity and advanced functionality. Its neural network is specifically tuned for colorization, adept at recognizing subtle textures and contextual elements within images to produce realistic results. It's continuously learning from user inputs, improving its color prediction abilities over time.
Palette.fm's ability to handle high-resolution images makes it useful for projects requiring meticulous detail. It also offers features like batch processing and adjustable color parameters, providing users with greater control. Interestingly, the platform promotes transparency by offering glimpses into its algorithmic decision-making, which builds trust amongst users.
While Palette.fm is a standalone tool, its growing user base has formed a collaborative community, sharing experiences and contributing to the platform's ongoing refinement. This combination of sophisticated technology, ease of access, and user-driven feedback positions Palette.fm as a top contender in the ever-evolving field of AI colorization in 2024.
Image Colorization vs
AI Image Generation Comparing Free Tools in 2024 - DALL-E 3 Balancing Quality and Accessibility
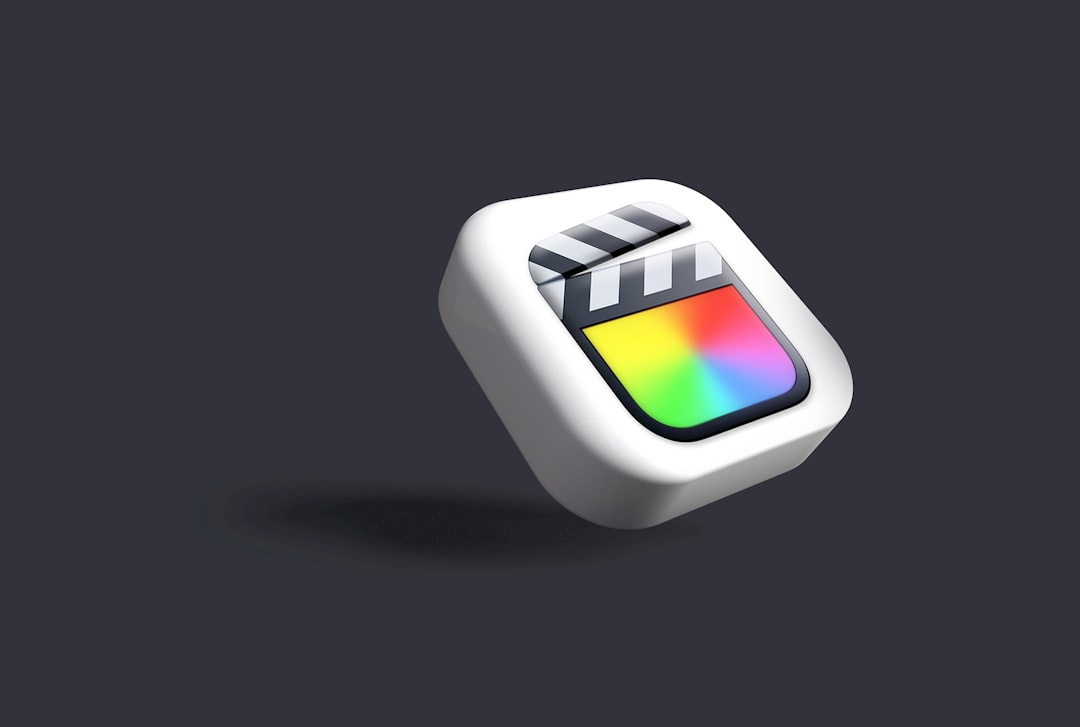
DALL-E 3 has become a prominent AI image generator in 2024, successfully blending high-quality outputs with user-friendly access. Its ability to understand intricate instructions and translate them into visually rich images is a major leap forward. This model stands out by consistently generating images that are not only detailed but also fit within the intended context. To improve user safety, DALL-E 3 incorporates measures aimed at preventing the generation of biased or harmful content, which is a notable step in responsible AI development. Unlike some other freely available tools, DALL-E 3 offers a higher degree of image clarity and adaptability for various creative projects, establishing a standard for others to strive towards. Despite its strengths, it's crucial to acknowledge that quality can still be inconsistent across AI image generators. Users should remain mindful of this variability as not all platforms deliver the same level of visual precision and dependability.
DALL-E 3, the newest version of OpenAI's text-to-image model, has made strides in understanding complex instructions, leading to more nuanced and refined image generation. They've incorporated better safety measures, which were rigorously tested by domain experts, to minimize the risk of producing images of public figures or harmful biases. It's known for creating highly detailed images across many topics, often achieving a high level of contextual accuracy.
It's worth mentioning FLUX1, developed by Black Forest Labs, which is an open-source competitor to DALL-E 3. This highlights that the text-to-image field has become quite competitive. DALL-E 3 has quickly become a popular choice for generating images, following the success of its predecessor, DALL-E 2. When compared to tools like Stable Diffusion and Midjourney, DALL-E 3 stands out for its vibrant images and its ability to closely follow the meaning of user prompts.
Its capabilities have proven useful in a variety of creative fields like art, design, and even engineering and design domains. It's particularly interesting how well it can generate images that adhere to specific technical constraints in those fields. The significant improvements in detail and image quality make DALL-E 3 one of the best options currently available in text-to-image generation.
It appears that AI image generation is going to continue advancing, with tools like DALL-E 3 setting the bar for quality and usability within the field. However, it's important to note that while DALL-E 3 is more advanced than its predecessors, it still has its limitations. The reliance on a feedback loop means that changes are constantly being made based on how users are employing the model. There's also still variability in its ability to generate images in real-time, which highlights a challenge for infrastructure improvements and keeping up with user demand. The constant refining and changes that are a part of using a model such as DALL-E 3 are interesting to study and could likely continue impacting this area for a long time.
Image Colorization vs
AI Image Generation Comparing Free Tools in 2024 - Google ImageFX and Its Imagen 3 Model
Google's Imagen 3 model represents a significant advancement in AI image generation, particularly in its ability to create images with exceptional realism and detail. It stands out by rendering intricate textures and fine details with a level of accuracy that surpasses some of its competitors. Google's ImageFX tool, powered by Imagen 3, makes this technology more accessible to users in the United States, making it a key player in the growing field of AI image creation. The easy access to this model in the US has also sparked discussions about the ethical and creative aspects of AI-powered tools, especially as its capabilities are compared to other readily available alternatives. Imagen 3 leverages a latent diffusion model to translate text prompts into compelling visuals, effectively bridging imagination and creation through the use of technology. While its free access is encouraging, it remains to be seen how it will impact the AI landscape.
Google's Imagen 3 model builds upon its predecessors, showcasing improvements in image quality through the use of a refined diffusion method. It excels at creating images from text prompts without needing specialized training, a capability known as zero-shot learning. This ability comes from its clever way of combining both visual and textual information to produce images that closely follow descriptions.
Imagen 3's training data is incredibly diverse, consisting of a huge number of pictures paired with captions, helping it to create a broader range of visuals and minimize the skewed outputs that often occur when training with limited data. One of the key draws of Imagen 3 is its focus on producing highly detailed and sharp images. This quality makes it attractive for tasks needing precise visuals, such as advertising or professional artwork.
Imagen 3 cleverly adapts its processing load based on the demands of a task or the number of users accessing it, allowing for more efficient resource management. Users have a significant degree of control over the generated images through a set of options that can alter style, color scheme, and even draw on specific artistic influences, providing a higher level of influence on the output.
Generating realistic-looking humans, a challenge for earlier models, is an area where Imagen 3 has seen significant advancement. When compared to other available tools, Imagen 3 performs extremely well in numerous evaluations that assess its ability to create realistic and contextually correct images.
However, Google has introduced a commercial licensing structure for Imagen 3, setting it apart from the open-access philosophy seen in many free AI tools. This introduces questions about the long-term accessibility and affordability of Imagen 3, particularly for smaller projects or individuals seeking to utilize high-quality AI generated content. This licensing model is something to consider when comparing Imagen 3 to other free options available.
Image Colorization vs
AI Image Generation Comparing Free Tools in 2024 - Comparing Output Quality Between Colorization and Generation
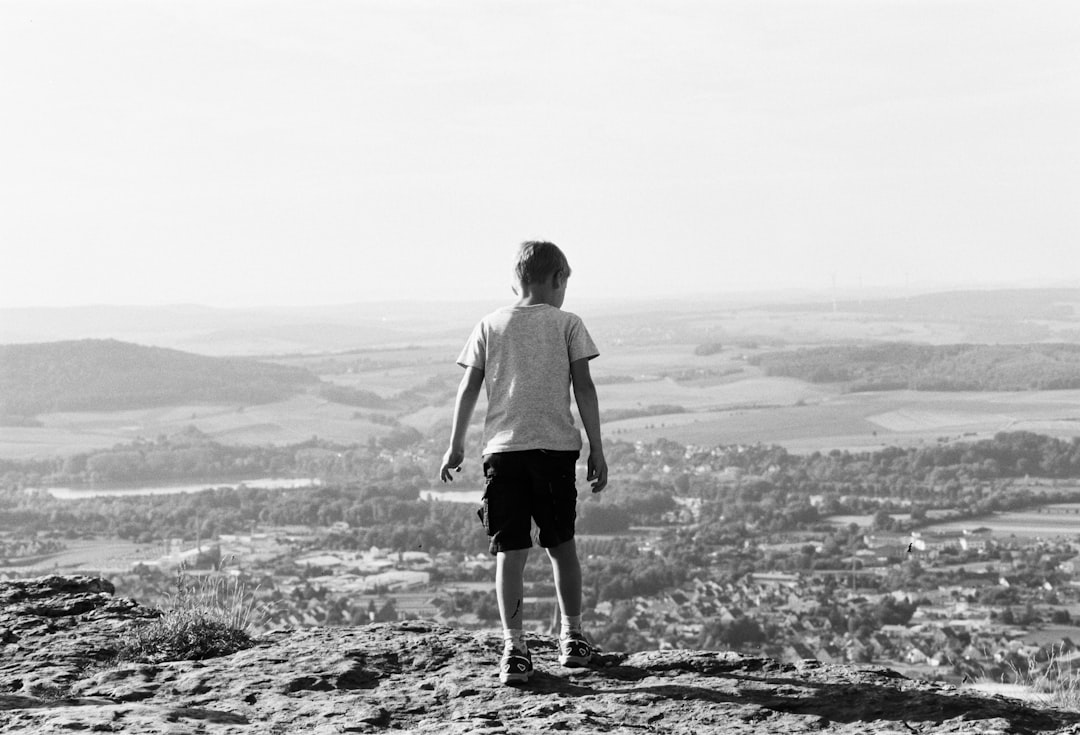
When comparing the output quality of image colorization and AI image generation, we see distinct differences in how accurately and convincingly each approach produces results. While colorization tools, using methods like Generative Adversarial Networks, have become highly skilled at applying color to images in a way that often seems very realistic, they still have trouble consistently getting the details and nuances of the scene right, and human viewers can disagree on whether the colorization is successful. On the other hand, AI image generation has progressed significantly in its ability to interpret complex prompts and create visually appealing images that fit within a specific context. Despite these advances, the quality of AI-generated images can vary greatly depending on the training data and the algorithms behind the tool. The field is always evolving, and understanding the subtleties of each technique will become more important as users rely on them for diverse applications.
When comparing the output quality of AI image colorization and image generation, it's clear that each approach has distinct strengths and weaknesses stemming from their underlying algorithms and training data. While both leverage deep learning, colorization techniques often favor convolutional neural networks (CNNs) for their ability to understand the spatial relationships within a grayscale image. In contrast, generative models, including GANs and diffusion-based approaches, prioritize the generation of entirely new content, which can lead to variations in quality when compared to colorized versions of the same image.
Furthermore, users typically have more control over colorization outputs. Many platforms offer adjustable parameters for hue and saturation, allowing users to fine-tune the final result. On the other hand, many image generation platforms offer limited control over stylistic elements, making it difficult for users to achieve their desired aesthetic.
Another difference lies in the stability of output styles. Colorization outputs can be susceptible to changes in the training data over time, reflecting shifts in artistic trends and biases within those datasets. In contrast, image generation models tend to maintain a more consistent style, defined by their underlying architectural design. While this can produce more uniform outcomes, it can also lead to a sense of homogeneity across outputs.
Interestingly, colorization tools often produce more realistic representations of the original objects, enhancing their appearance with color while largely maintaining original form and structure. Generative models, especially those lacking more advanced convolutional neural network structures, can struggle to create photorealistic images, especially when it comes to intricate textures and nuanced shadow play.
Processing speed is another differentiating factor. Colorization tools have seen a remarkable increase in processing efficiency, often colorizing an image within a few seconds. In comparison, image generation, particularly with complex images, can still take a few minutes, demonstrating the inherent complexity of the image synthesis process.
The original training data also plays a crucial role in influencing the outcome. Colorization models rely on these datasets to develop an understanding of how colors are used within different contexts. The diversity and richness of this training data directly impacts the quality of colorization. Generative models, conversely, leverage training data to improve the breadth of subjects and potential outputs, leading to significant variation in quality and accuracy across different models.
Some colorization tools incorporate edge detection techniques to identify key features before applying color, which leads to more visually coherent results where colors respect original boundaries. Generative networks, on the other hand, sometimes overlook these edges, leading to less-defined or inappropriately blended regions in the final image.
The methods used for assessing output quality also vary. Colorization tools frequently aim to achieve historically or culturally accurate colors based on the grayscale input. Generative models, however, tend to rely more on subjective assessments, often based on user preferences and community feedback.
Colorization tools have a clear objective—enhancing existing images. Image generation tools, on the other hand, tackle the more complex and abstract task of creative synthesis. This difference can sometimes lead to outputs that deviate from user expectations, depending on how prompts are interpreted by the models.
Finally, the role of user communities in the ongoing development of both types of tools is notable. While colorization platforms frequently refine their algorithms based on direct feedback about color fidelity, generative platforms utilize a broader array of user input to modify their visual styles and functionalities, shaping future development trajectories.
More Posts from colorizethis.io: