Colorize and Breathe Life into Old Black-and-White Photos (Get started for free)
The Ethical Implications of AI-Powered Skin Tone Editing in 2024
The Ethical Implications of AI-Powered Skin Tone Editing in 2024 - AI Algorithms Favor Lighter Skin Tones in Image Editing
The way AI algorithms edit images has revealed a concerning tendency to favor lighter skin tones. This bias often leads to altered images that inadvertently diminish the natural appearance of darker skin tones. Underlying this issue is a reliance on older skin tone classification systems like the Fitzpatrick scale, which were primarily designed for lighter skin and don't adequately capture the full spectrum of human skin color.
The ethical implications of this bias are significant, extending beyond mere aesthetics. These algorithms are not only impacting how individuals are portrayed in images but also potentially perpetuating damaging social stereotypes connected to skin tone. In response to these concerns, innovative metrics like the "Hue Angle" system are being developed to help better identify and quantify the problem of underrepresentation in training data used to develop AI algorithms.
Moving forward, developers need to be more aware of these biases and work towards creating more inclusive AI systems. Achieving fair and accurate representation of all skin tones in image editing is essential to ensure that digital platforms do not contribute to harmful biases and instead promote authenticity and respect.
AI algorithms, when applied to image editing, have shown a tendency to favor lighter skin tones. Researchers have observed that these algorithms often struggle to accurately represent darker skin tones, leading to misinterpretations of color and potentially distorted features. This discrepancy is likely tied to the composition of training datasets, which frequently lack a diverse representation of skin tones. The over-reliance on data primarily featuring lighter skin creates a bias that disproportionately impacts the quality of edits for darker skin tones.
It's been suggested that the built-in adjustments for skin tone in many automated editing tools can unintentionally reinforce stereotypes and biases. This can raise ethical concerns about the implications of how different skin tones are presented in a wide range of media, particularly in advertising and marketing. We've also seen some evidence that users may feel compelled to modify their appearance to fit societal norms—norms that seem to be reinforced by how these algorithms operate.
Furthermore, there's a growing recognition among developers that current methods for testing bias in these algorithms might not be sufficient. Existing evaluation strategies often fail to fully capture the natural variability of skin color. This has sparked interest in new approaches, like "Hue Angle", that are aimed at improving assessments of skin tone representation within AI training data. A more nuanced approach to measuring bias is essential. While a few engineers advocate for a more representative range of skin tones in training datasets, we also see instances where generative AI seems to amplify existing biases related to skin tone, which only makes matters more challenging.
These algorithmic biases are not simply aesthetic concerns, they potentially impact representation and visibility across diverse platforms like social media and in different industries. The potential downstream effect of these biases might be far-reaching, with implications that need further investigation. Consequently, ongoing research is critical to ensure that future developments in AI, particularly in image editing, lead to equitable and accurate representation for all skin tones. It’s vital that the growing use of these AI tools doesn't inadvertently worsen existing inequalities. To that end, it’s worth exploring the possibilities of future regulatory oversight for these systems to guarantee fairness and equitable representation.
The Ethical Implications of AI-Powered Skin Tone Editing in 2024 - Sony's Research Reveals Gaps in Traditional AI Bias Tests
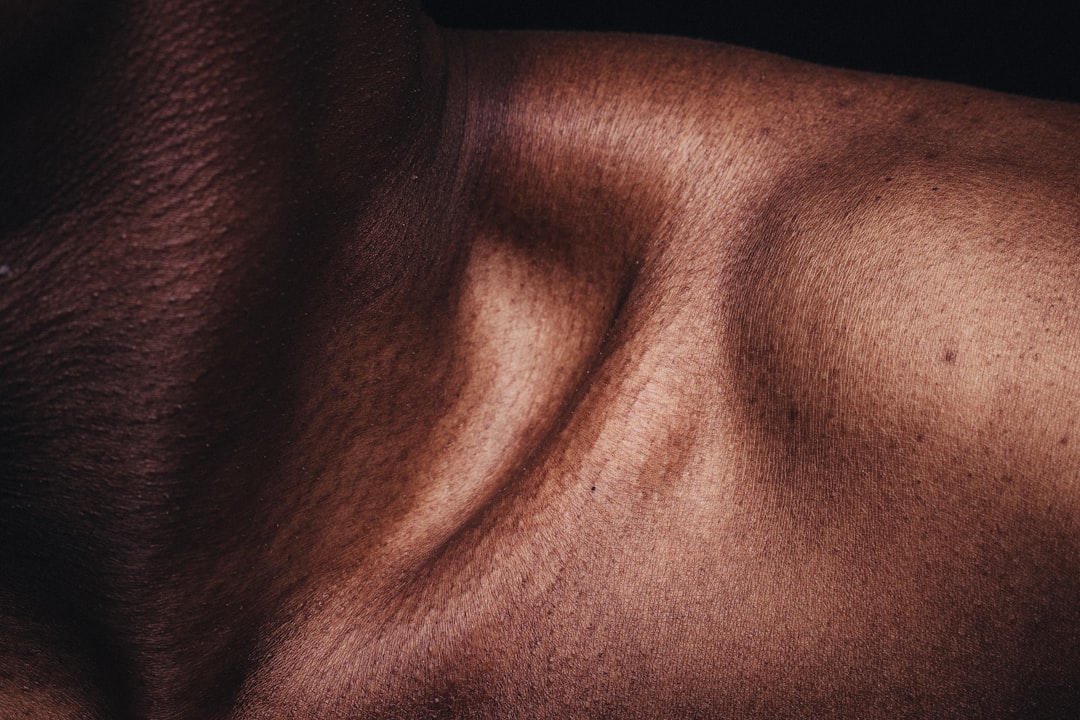
Sony's research has brought to light a crucial issue: conventional methods for assessing bias in AI, especially when it comes to skin tone, are insufficient. Their findings indicate that existing tests often fail to capture the subtle differences in skin hues, highlighting the complex nature of AI bias. The reliance on tools like the Fitzpatrick scale, initially designed for lighter skin, further reveals the limitations of current approaches in adequately representing the full spectrum of human skin tones. This research emphasizes the need for innovative ways to identify and address AI bias more accurately. Moving forward, it's crucial to develop new assessment tools that encompass a broader range of skin tones. As AI becomes increasingly integrated into society, it's vital to ensure that its development doesn't inadvertently reinforce existing biases or create new ones. Failure to address these issues in the development and evaluation of AI algorithms risks perpetuating and even amplifying societal inequities.
Sony's recent research suggests that standard methods for detecting bias in AI, especially when it comes to skin tone, fall short. They've found that these tests often don't fully capture the range and nuances of human skin color. This suggests we need to rethink our approaches and develop more sophisticated methods beyond the typical metrics used.
It seems many bias assessments focus mainly on things like how accurate the AI is and its error rate. They overlook how the AI actually represents different skin tones. This implies developers may need to incorporate more qualitative evaluations of representation in their assessments.
Interestingly, the study reveals inconsistencies in automated skin tone adjustments during real-time editing, particularly in scenarios with varying lighting. This brings to light some limitations in the current AI algorithms designed for these tasks.
It’s also noteworthy that even algorithms intended to reduce bias can sometimes make things worse. If the calibration process doesn't consider the original artistic intent or cultural background of an image, it can inadvertently increase the disparities.
The research indicates that user feedback in refining AI skin tone algorithms hasn't been thoroughly integrated. This creates a disconnect between technological improvements and the actual experiences of people with darker skin. This disconnect might point to the need to incorporate a more participatory design process.
The shortcomings in current bias assessments seem to mirror a broader pattern across different industries where speed and efficiency often take precedence over ethical considerations. As AI tools become more prominent in creative fields and advertising, the issue of accountability becomes more critical.
There's a clear link between how well skin tone editing algorithms work and the socioeconomic groups that contribute data to train them. This highlights that biases aren’t just inside the algorithms themselves; they’re rooted in broader societal contexts and structures.
Further, many of these algorithms appear to have a preference for specific cultural standards of beauty. This suggests that, even with improved training datasets, inherent biases might still persist unless we critically examine the cultural norms that influence the development of these systems.
Researchers are also finding that bias isn’t something fixed within an algorithm. It can evolve over time as the AI learns and adapts to new data. This underscores the importance of continuously monitoring and adjusting these systems throughout their development and deployment.
Lastly, the findings imply that cross-disciplinary collaboration is vital in resolving the limitations of current bias tests. Bringing together psychologists, ethicists, and engineers could pave the way for fairer AI systems in image processing and related areas.
The Ethical Implications of AI-Powered Skin Tone Editing in 2024 - Limitations of the Monk Scale for Skin Tone Classification
The Monk Scale, introduced in 2023, aimed to improve upon existing skin tone classification systems like the Fitzpatrick scale by providing a wider range of skin tones. This was a step towards greater inclusivity, especially given the Fitzpatrick scale's limited representation of darker skin tones. However, the Monk Scale is not without its own limitations when applied in real-world scenarios, particularly within AI image editing. There's a risk that the scale simplifies the natural spectrum of skin tones, potentially resulting in mischaracterizations when integrated into automated processes. Additionally, despite the scale's efforts to foster inclusivity, it cannot entirely eliminate the historical biases present in the datasets used to train AI algorithms. This raises serious ethical questions about the potential for these tools to inadvertently reinforce social inequities. As we move forward in this field, it's crucial to critically assess the effectiveness of tools like the Monk Scale. We need to ensure that these classification systems genuinely promote fairness and accurate representation for all individuals, rather than potentially contributing to existing biases.
While the Monk Scale aimed to improve upon the limitations of the Fitzpatrick scale by providing a broader range of skin tones, it still falls short in several key areas when it comes to accurate and nuanced skin tone classification, particularly for darker skin tones. The scale's design doesn't fully address the unique characteristics of melanin-rich skin, leading to a lack of granularity when distinguishing between subtle shades. This can be a problem in various applications, including image analysis, where accurately capturing these subtle variations is critical for a true representation of skin tone.
Furthermore, there's a concern that relying solely on the Monk Scale may inadvertently contribute to colorism. Its framework might unintentionally reinforce existing societal biases that favor lighter skin tones, potentially leading to less accurate and potentially biased representations in AI systems. The criteria used in the Monk Scale's categorization, while aiming for inclusivity, can sometimes lead to misrepresentation in AI-powered image editing, especially when it comes to preserving the natural appearance of darker skin tones.
Moreover, the Monk Scale's approach doesn't adequately factor in the effects of environmental elements, like lighting conditions and background, which can significantly impact how skin tones are perceived in digital images. This oversight can contribute to inaccurate skin tone assessments and subsequently, lead to unintended alterations in images when these algorithms are applied.
Beyond technical shortcomings, the Monk Scale also overlooks the psychological dimensions of skin tone. It fails to consider how inadequate skin tone classification in digital spaces can potentially impact self-perception and the way individuals view themselves in relation to broader social norms over time.
Further complicating the situation, researchers have noted that tools relying on the Monk Scale struggle with variations in skin texture and undertones. This can lead to inconsistent results in automated image editing tools that rely on accurate skin tone detection for adjustments, particularly in applications where fine details and nuanced representations matter.
The limitations of the Monk Scale raise questions about its suitability in the context of the ever-growing creation and consumption of digital content. In an age where authenticity and inclusivity are highly valued, the scale's restricted scope may not be adequate for achieving accurate and diverse representations in visuals.
There's a growing sense that the current limitations of the Monk Scale are hindering advancements in image processing technologies. The need for more comprehensive skin tone classification systems that go beyond the parameters of the Monk Scale is becoming more pressing as developers seek to build AI tools that offer a more accurate representation of the full spectrum of human skin tones. The binary approach used in the Monk Scale, while an improvement over older scales, simply doesn't reflect the full complexity and spectrum of human skin pigmentation. The pursuit of frameworks that are capable of capturing this rich diversity in a more accurate manner becomes crucial for fostering more equitable and inclusive applications of AI in areas like image editing.
The Ethical Implications of AI-Powered Skin Tone Editing in 2024 - Skin Tone Bias in AI Affects Healthcare and Employment
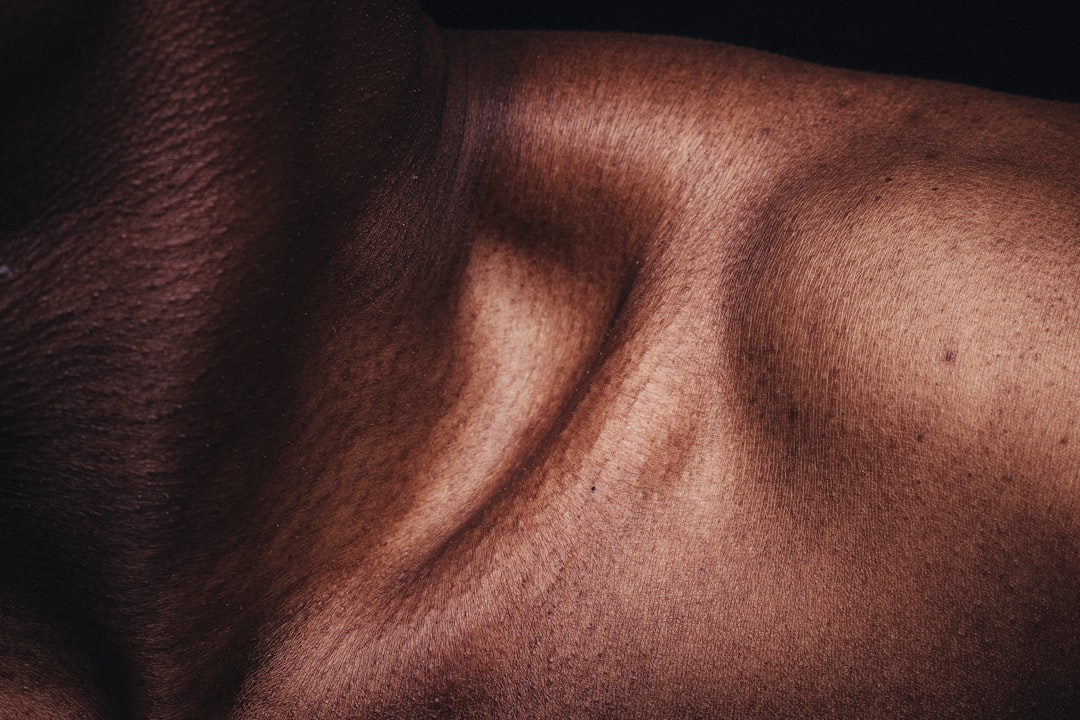
AI's increasing use in healthcare and employment has brought to light a concerning issue: skin tone bias. Algorithms trained on data that doesn't adequately represent the full spectrum of skin tones can lead to inaccurate and potentially harmful outcomes. For instance, in healthcare, biased AI can affect diagnosis, particularly in fields like dermatology, where accurate skin analysis is crucial. This can erode trust and exacerbate existing health disparities. Similarly, in employment, biased algorithms used in hiring processes can lead to unfair and discriminatory practices, perpetuating systemic inequalities.
These biases can arise from the use of older skin tone scales that don't capture the diversity of human skin, leading to skewed datasets. As we become more aware of the detrimental impact of these biases, there's a growing push to improve data representation and develop more inclusive AI frameworks. This is crucial to ensure that AI benefits all people, regardless of their skin tone, and to avoid inadvertently amplifying existing social inequalities. Addressing these biases requires thoughtful consideration and careful development of AI systems that promote fairness and equity across various fields.
AI systems, particularly those trained on datasets primarily featuring lighter skin tones, are showing concerning biases that can have real-world implications in areas like healthcare and employment. For instance, in healthcare, diagnostic tools trained on limited skin tone variety may struggle to accurately identify skin conditions that are more common in individuals with darker skin tones. This can lead to misdiagnoses and potentially delayed or inadequate treatment, worsening existing health disparities.
Similarly, AI algorithms used in recruitment, such as those that screen resumes, might inadvertently favor candidates with lighter skin tones, reducing the diversity of talent pools and perpetuating existing inequalities within organizations. We've also seen indications that facial recognition technologies used in security systems exhibit similar biases, often being less accurate in identifying individuals with darker skin tones, leading to higher error rates.
A deeper look at the training data reveals that many of these biases are rooted in historical underrepresentation of darker skin tones in healthcare data. This historical bias can influence the outcomes of AI systems, potentially skewing diagnoses and treatment recommendations. This also points to the concerning idea that individuals from historically marginalized communities might adjust their online behavior to fit what AI systems, and potentially society as a whole, deems attractive.
Beyond healthcare and employment, the implications of skin tone bias can be seen in areas like finance. AI systems used for loan approvals or credit scoring have shown a tendency to disadvantage individuals with certain skin tones, exacerbating financial inequalities.
The growing awareness of these biases has prompted concerns within the medical community. Researchers are calling for more rigorous audits of the data used to train AI systems in healthcare to ensure a more inclusive representation of skin tones. Furthermore, the controversial use of AI in defining beauty standards for marketing has raised ethical concerns regarding potential psychological impacts on individuals and how it may perpetuate harmful ideas about skin tone.
Although some progress has been made in creating more inclusive skin tone classification systems, a lot of research indicates that reliance on historically biased datasets persists. This can limit the effectiveness and applicability of these systems in the real world.
It's clear that the ethical implications of skin tone bias in AI extend far beyond individual experiences. If unchecked, it can lead to a general decline in public trust in AI technologies, especially those employed in sensitive fields like healthcare and hiring. These industries, more than others, require transparency, fairness, and demonstrably accurate results, and a failure to address skin tone bias could lead to further skepticism about AI's usefulness in sensitive areas. As engineers, it's imperative that we grapple with these issues and aim to build AI systems that serve everyone fairly and accurately, regardless of skin tone.
The Ethical Implications of AI-Powered Skin Tone Editing in 2024 - Advanced Facial Recognition in Beauty Industry AI
The beauty industry's adoption of sophisticated facial recognition powered by AI is transforming how products are developed and personalized, offering new avenues for customized skincare and diagnostics. This shift towards hyper-personalization is undeniably exciting, yet it necessitates a careful examination of its ethical implications. While these AI systems promise tailored experiences, they often struggle with accuracy and fairness when dealing with a diverse range of skin tones, especially those darker than the standard. This inherent bias in the algorithms continues to raise concerns about potential biases and reinforces the urgent need for regulations and oversight. Furthermore, the industry must address issues surrounding privacy and surveillance as these technologies become more ubiquitous. The risk of AI inadvertently perpetuating or even amplifying pre-existing societal preferences for lighter skin tones is a significant concern. Moving forward, the beauty industry has a responsibility to ensure that AI-driven solutions promote inclusion and fairness for all, lest they exacerbate existing inequalities rather than promoting a more equitable beauty landscape.
The beauty industry's embrace of AI has led to the development of sophisticated facial recognition systems, capable of identifying over 200 unique skin tone attributes. This level of detail goes beyond the simpler light-to-dark classifications of older systems, offering the potential for more accurate representation of a wider range of skin tones. However, research reveals a persistent challenge: facial recognition often misclassifies individuals with darker skin tones due to a lack of diversity in the data used to train these systems. Studies have shown that algorithms can struggle to accurately discern attributes like texture and blemishes, underscoring the impact of initial biases in training datasets on real-world applications.
The application of facial recognition in the beauty realm extends beyond simply enhancing aesthetics. These technologies are increasingly influencing online beauty standards and consumer behavior. The growing popularity of skin tone-filtering tools raises concerns about the perpetuation of unrealistic beauty ideals, potentially skewing perceptions of attractiveness across different demographic groups.
It's interesting that while these systems can detect subtle variations in skin undertones that even human experts might miss, these advanced capabilities are not always utilized effectively due to the limited diversity of skin tones present in training data. This means that models primarily trained on lighter skin tones can inadvertently exclude features common in darker skin, like freckles or natural skin textures. These features may be ignored or incorrectly portrayed, leading to misrepresentations.
Furthermore, AI-driven beauty recommendations often lean towards Eurocentric beauty standards, raising ethical concerns. These recommendations can unintentionally reinforce harmful stereotypes, potentially undermining the inherent beauty of diverse skin tones and contributing to the perpetuation of biases in digital depictions. Additionally, the ability of AI to analyze skin conditions using facial recognition varies greatly across skin tones. Certain conditions, such as hyperpigmentation, might not be consistently detected in darker skin, complicating even basic analyses.
We also see challenges when these technologies are applied across borders. Systems built in regions with predominantly lighter skin populations can struggle in areas with a higher concentration of darker skin tones, limiting their utility and leading to product mismatches in global markets. In some cases, this mismatch has resulted in an increase in cosmetic product returns as consumers discover that the AI-powered shade recommendations don't accurately reflect their actual skin tones.
The potential for ongoing misrepresentation becomes a significant factor, especially considering how biases can adapt within software based on user interactions. This reinforces the importance of maintaining a critical perspective during algorithm development and implementation, alongside ongoing adaptation and potentially, future regulatory measures to address these evolving biases. The ethical landscape of using advanced facial recognition in beauty is multifaceted and demands constant attention to ensure that AI tools promote inclusivity and fairness, rather than inadvertently exacerbate existing disparities.
The Ethical Implications of AI-Powered Skin Tone Editing in 2024 - Ethical Concerns of Generative AI in Professional Editing
The integration of generative AI into professional editing presents a complex set of ethical challenges. Concerns regarding the privacy of data used to train these systems, the potential for copyright infringements, and the risk of spreading misinformation are just some of the issues that need careful consideration. The capacity of generative AI to produce highly convincing synthetic content, including deepfakes, raises serious questions about authenticity and the reliability of information in the edited work. This further complicates the existing roles and dynamics between authors and editors, potentially altering traditional publishing practices. Additionally, inherent biases within the AI's training data can amplify existing social disparities, leading to potentially unfair and harmful outcomes. As the use of generative AI expands, there's an increasing call for transparency and accountability in its application. Furthermore, the evolving landscape demands a thorough exploration of how to align the use of AI with fundamental societal values, ensuring that its benefits are broadly shared while mitigating potential harms.
Generative AI (GnAI) presents intriguing possibilities for collaborative creativity and editorial tasks, especially within publishing. However, its increasing use in professional editing also introduces complex ethical issues, especially as these systems frequently carry forward existing biases embedded in their training data. The underrepresentation of darker skin tones within training datasets can amplify existing inequalities, leading to unfavorable outcomes in industries reliant on accurate image editing.
GnAI's unique adaptive learning capability allows it to evolve based on the data it's exposed to. This constant adaptation can unfortunately worsen biases if the training data remains skewed, making it challenging to ensure fair representation within the editing process.
Editors using GnAI might unintentionally contribute to the propagation of stereotypes because the automated suggestions often lean towards lighter skin tones. Since the inner workings of these algorithms aren't always transparent, it can lead to a consistent reinforcement of societal biases that editors may not consciously endorse.
Recent research reveals a gap between the potential of GnAI and the understanding of skin tone representation amongst developers. Many engineers haven't received the necessary training or insight into the intricate variations within the spectrum of human skin color, which can act as a roadblock to building more equitable systems.
Beyond bias, integrating GnAI into image editing also raises privacy concerns. These AI systems frequently rely on substantial databases to analyze and modify images, potentially leading to the unintentional exposure of personal data or images without explicit consent, creating ethical violations.
Traditionally, GnAI models are assessed based on their performance metrics like accuracy and speed. This focus can overlook the ethical dimensions of representation, resulting in systems that fall short of adequately capturing the full range of skin tones.
The rapid integration of GnAI in professional editing might outpace the development of regulatory structures that could ensure fairness. Without timely intervention, there's a risk of the technology deepening existing biases within creative industries where inclusive representation is vital.
As GnAI continues to mature, the accountability of developers and engineers becomes increasingly important. A failure to scrutinize the outputs of these systems could result in persistent biases that impact numerous individuals, especially those with darker skin tones.
The psychological effects of biased GnAI are profound. The inaccurate portrayal of skin tones can influence societal beauty standards and alter self-perception. This isn't limited to individual experiences, but potentially impacts broader cultural dynamics.
Finally, the continued use of older skin tone classification systems like the Fitzpatrick scale within GnAI models can hamper progress. Novel, inclusive frameworks are needed to address the historical biases inherent in current editing technologies, guaranteeing that all skin tones are represented accurately and fairly.
Colorize and Breathe Life into Old Black-and-White Photos (Get started for free)
More Posts from colorizethis.io: