The Science Behind AI-Powered Image Colorization How Algorithms Breathe Life into Black and White Photos
The Science Behind AI-Powered Image Colorization How Algorithms Breathe Life into Black and White Photos - Understanding the Basics of AI-Powered Image Colorization
AI-powered image colorization has emerged as a transformative technique, allowing for the automatic addition of color to black and white images. This process, previously the domain of skilled artists, now relies on sophisticated algorithms that estimate the missing RGB color values. These algorithms, often powered by deep learning, essentially make educated guesses about colors based on the patterns and features present in the grayscale image. This process, a core aspect of computer vision, involves assigning specific colors to each pixel.
The use of large training datasets has been crucial in enhancing the accuracy and speed of these AI colorization tools. These systems essentially learn from millions of examples, building a knowledge base to understand relationships between shades of gray and associated color information. This learning approach has opened doors for applications beyond mere aesthetic enhancement. AI-powered colorization finds utility in a range of disciplines, including areas like medical imaging where it can assist in visualizing otherwise hard-to-interpret information.
As the field of generative AI continues to progress, we're witnessing a rise in more capable and accessible tools for image colorization. The increasing sophistication of these algorithms indicates a future where the line between authentic and digitally enhanced color continues to blur, presenting both opportunities and potential pitfalls for image interpretation.
Essentially, AI colorization involves teaching computer algorithms to understand the nuances of grayscale images and then "fill in" the missing color information. This is achieved by training them on vast collections of images, where each black-and-white picture has a corresponding colored version. The algorithm learns to associate patterns and features in the grayscale image with specific colors, allowing it to make educated guesses about what the original image might have looked like in color.
However, these guesses can be subjective. Sometimes the AI might produce colors that weren't actually present in the original, instead relying on its learned associations of similar scenes or objects. This highlights a crucial aspect of the technology: it's based on learning, not necessarily on recreating an exact copy. It's more about interpretation than absolute truth.
To refine these guesses, techniques like attention mechanisms are employed. These allow the algorithms to focus on specific areas or elements within the image, aiding in more accurate and detailed color assignments. It seems different types of images, like portraits versus landscapes, require subtly different approaches as the typical color schemes associated with each type of image differ.
Despite significant progress, there's still a degree of uncertainty in certain scenarios. If the original image is inherently ambiguous in its color palette, the AI might struggle to produce a consistent result. There are methods to assess the quality of the colorization like PSNR and SSIM, but these measurements don't always completely align with what we humans perceive as aesthetically pleasing or realistically colored.
Intriguingly, some AI colorization systems allow user feedback to influence the final result. This suggests a fascinating interplay between artificial intelligence and human creativity. Further, some more advanced methods let users guide the colorization process by providing their own suggestions or preferences, effectively making the AI colorization more collaborative.
Expanding the scope beyond still images, researchers are applying these ideas to colorizing video footage. This poses greater challenges, since the AI must account for the dynamic nature of video, including motion and maintaining color consistency across frames. The field is continuously evolving, and it'll be exciting to witness how the technology progresses.
The Science Behind AI-Powered Image Colorization How Algorithms Breathe Life into Black and White Photos - The Role of Convolutional Neural Networks in Color Estimation
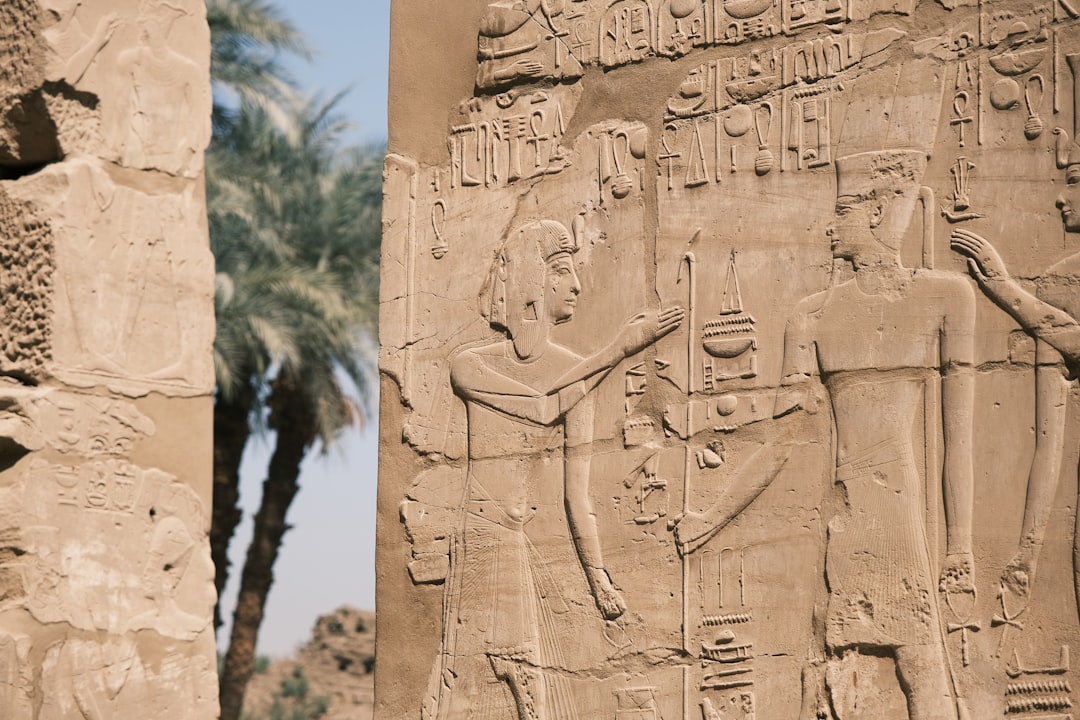
Convolutional Neural Networks (CNNs) are at the heart of modern color estimation in AI-powered image colorization. They excel at recognizing and understanding the meaningful details within a grayscale image, forming the basis for predicting and assigning appropriate RGB color values. This capability allows CNNs to automatically breathe life into black and white photos, offering a significant improvement over the previous, manual colorization techniques which often resulted in inaccurate and less vibrant outputs.
CNNs learn by processing vast quantities of image data, building a complex understanding of how different shapes, textures, and patterns relate to specific colors. This learning process enables them to perform colorization tasks with increased speed and accuracy. However, it's important to acknowledge that CNNs, like all machine learning models, can be subjective in their color choices. While they've proven adept at associating certain image elements with specific colors, they don't always perfectly recreate the original colors, highlighting that image colorization is fundamentally about educated estimations rather than perfectly replicating past colors. There's an inherent interpretation element embedded within the process that must be considered when viewing the final results. Despite this, the role of CNNs in advancing color estimation has been transformative, opening up new possibilities for enhancing images and potentially even aiding in diverse fields beyond mere aesthetic enhancement.
Convolutional Neural Networks (CNNs) have proven particularly effective in the realm of color estimation due to their inherent ability to discern patterns. They excel at recognizing complex features within grayscale images, allowing them to make informed guesses about the missing color information. The multi-layered structure of CNNs, with each layer progressively extracting more abstract information, allows for the capture of subtle variations in texture and shading. These nuances, which are often key indicators of color, can be leveraged to improve colorization accuracy.
Maintaining image integrity during colorization is important, and the incorporation of skip connections within CNN architectures helps achieve this. These connections facilitate the flow of spatial information across layers, preventing the loss of crucial details that might otherwise affect the final colored output, particularly in intricate image areas.
Rather than starting from scratch, researchers often employ transfer learning with CNNs in color estimation. This technique leverages pre-trained CNN models that have been trained on vast image datasets. By fine-tuning these pre-trained models for specific colorization tasks, the need for extensive training data and computational resources is minimized.
Data augmentation techniques are a common strategy in training CNNs for colorization. These methods involve manipulating existing images – such as rotating, flipping, or subtly adjusting colors – to artificially increase the size of the training dataset. This broader dataset improves the model's robustness and generalizability, ensuring better performance across a wider range of scenarios.
Attention mechanisms have emerged as a key innovation in CNNs for image colorization. By integrating these attention mechanisms, the CNN can focus on specific regions within the image, enabling it to make more relevant color choices. The focus on specific areas allows the model to be more sensitive to context and thus make color assignments that are more appropriate for the scene or object depicted in the image.
Another interesting approach is adversarial training. Here, two neural networks compete against each other to refine color estimation accuracy. One network attempts to create colorized images, while the other scrutinizes the output, assessing its authenticity. This constant back-and-forth fosters improvements in colorization, leading to results that are more realistic.
The ability of CNNs to perform colorization in real-time presents exciting possibilities across diverse domains. Virtual and augmented reality are just two areas where this capability is highly valuable, enabling users to experience immersive environments with instant colorization feedback.
Despite the impressive advancements, CNNs still face limitations, particularly in situations where the original grayscale image is inherently ambiguous or presents unusual subjects. In these cases, CNNs may struggle to produce a consistent and believable colorization. This challenge highlights that solely relying on automated interpretation without incorporating human input can be problematic.
The continual development of new CNN architectures, including the innovative integration of Generative Adversarial Networks (GANs) has pushed the frontiers of colorization technology. The combination of these approaches is now capable of producing high-quality colorized images that often closely mirror the appearance of real-world photographs. The journey toward perfect colorization is an ongoing process, but the integration of CNNs and new techniques continues to push the boundaries of this challenging and exciting field.
The Science Behind AI-Powered Image Colorization How Algorithms Breathe Life into Black and White Photos - Advancements in Deep Learning Techniques for Automatic Colorization
Deep learning has spurred remarkable progress in automatically colorizing grayscale images. These advancements have led to algorithms that are significantly more accurate and efficient than their predecessors. Modern approaches emphasize training models on extensive image datasets, enabling them to learn complex relationships between grayscale features and corresponding color information. This learning process involves predicting the missing RGB color values based on the patterns and nuances within the original black-and-white photos.
Convolutional neural networks (CNNs) play a pivotal role in this process, demonstrating a remarkable ability to extract fine-grained details from images. CNNs are instrumental in generating colorized outputs that are both visually appealing and contextually appropriate, a significant leap beyond previous, often inaccurate manual approaches. The field continues to explore new directions, including techniques like self-regularization and adversarial training, with the aim of creating even more refined colorization methods. However, it's crucial to acknowledge that the subjectivity of color choices remains a central issue. While AI-driven colorization has come a long way, the final output often reflects an educated guess, not necessarily a perfect reproduction of the original colors. This raises interesting questions about the authenticity of the resulting images and how we interpret their perceived reality.
The field of automatic image colorization using deep learning has seen significant strides, with a growing reliance on generative models like Generative Adversarial Networks (GANs). These systems pit two neural networks against each other, one generating colorized images and the other evaluating their realism. This adversarial setup has proven particularly effective in refining the quality of colorized outputs, leading to results that often more closely resemble real photographs.
Integrating attention mechanisms into these deep learning models has further enhanced their capabilities. By focusing the algorithm's attention on specific parts of an image, these mechanisms allow for more contextually relevant color assignments. This means the algorithm can now better grasp the scene or object depicted and apply colors that are more aligned with those commonly found in similar situations.
Recent developments have also highlighted the benefits of multi-scale feature extraction. By examining images at different resolutions, these techniques capture both fine details and broader context, leading to improvements in the accuracy and overall quality of the colorization.
Interestingly, incorporating user input into the training process has proven to be a valuable strategy. By including examples of colorizations that humans find appealing, researchers can guide the algorithm towards learning human preferences for color aesthetics, balancing the algorithm's output with what we consider desirable. The training datasets themselves are also evolving, now incorporating a broader range of images. This broader scope often includes not only standard color photographs but also artistic interpretations of black and white images, giving the models a more diverse understanding of potential colorization styles.
The ability to colorize videos has also progressed significantly. Advanced deep learning techniques now utilize temporal information across frames to maintain visual consistency during motion and varying light conditions. This complex task has posed unique challenges, but recent successes suggest these models can accurately colorize scenes that have dynamic movements, adding a significant new dimension to the applications of this technology.
Despite the advances, a key limitation remains: the inherent subjectivity of human color perception. A model might generate a vibrantly colored image, but if it doesn't align with human aesthetic preferences, it can fail to be perceived as pleasing or natural. This highlights the need for continued research that considers human feedback as a crucial aspect of the training and evaluation processes.
The quest for real-time colorization has also gained momentum. Improvements in algorithmic efficiency have made it feasible for deep learning models to process images quickly enough for applications like live streaming or interactive media. This capability opens doors for a more engaging user experience with instant color feedback.
Researchers are also exploring unsupervised learning methods for colorization. This approach could potentially reduce the reliance on large, labelled datasets, making colorization technology more accessible for a broader range of applications and less dependent on extensive curated datasets.
A promising area of future development lies in the exploration of hybrid models that merge rule-based methods with deep learning approaches. This could potentially provide a balance between the creative freedom allowed by deep learning and the structured outputs that rule-based systems can offer. This fascinating area opens up avenues for integrating human creativity more directly within these algorithmic workflows, potentially blurring the line between artist and AI.
The Science Behind AI-Powered Image Colorization How Algorithms Breathe Life into Black and White Photos - Challenges in Accurately Replicating Historical Color Information
Accurately recreating the colors of historical black-and-white photos presents a major challenge for AI. The fundamental problem is that grayscale images inherently lack the complete color information needed for a perfect reconstruction. While AI algorithms are getting better at predicting and assigning colors, they often rely on patterns and associations learned from training data. This means their color choices are often interpretations rather than direct replications of original colors, introducing a degree of subjectivity into the process.
The lack of readily available historical context for many black-and-white photos further hinders accurate colorization. AI models might not have the specific information needed to understand what colors were truly present in the original scene. This can lead to inaccuracies that affect the historical authenticity of the colored images.
Furthermore, if the training datasets are limited, AI algorithms might not learn a wide enough range of colors that would have been used in a particular historical period. This can produce colorizations that appear dull or not true to the era depicted. As this technology develops, balancing advanced AI methods with the goal of preserving historical color accuracy remains an important issue.
Accurately replicating historical color information presents a unique set of challenges for AI colorization algorithms. Our understanding of color and the tools used to reproduce it have evolved considerably over time. Older photographic processes often lacked the ability to faithfully capture the full spectrum of colors, creating a discrepancy between the actual colors of a scene and how they were recorded.
Furthermore, the aging and deterioration of the physical materials used in these older images themselves introduce difficulties. Fading and discoloration can alter the original colors, making it hard for AI to determine what the original hues truly were. Human memory and interpretation also contribute to the problem, since individual experiences and cultural contexts can heavily influence how we recall and perceive historical colors. It's inherently subjective, making it difficult to define a truly "accurate" color for a historical image.
The lack of detailed spectral information in many historical photographs further complicates matters. Without the precise data about the colors present in the original image, AI models must rely on educated guesses about color associations, introducing a degree of uncertainty into the colorization process. They often end up relying on learned patterns, not solid color information, to guide their estimations.
When faced with scenes that are inherently ambiguous, or have a variety of textures or lighting conditions, AI algorithms struggle to reach a consistent result. Without strong contextual clues, the final color choices can differ significantly, based entirely on the algorithm's prior training experiences. Additionally, AI colorization models often rely on training datasets which might not fully represent all historical contexts and artistic styles. This can lead to a bias in the output, favoring certain periods or styles over others.
The psychology of color adds yet another layer of complexity. Colors carry emotional weight that varies depending on individual and cultural perspectives. Even if AI could perfectly reproduce a historical color, it might not evoke the same emotional response in a modern viewer, or be interpreted as the original creator intended.
Even with the advancements in deep learning, current AI colorization algorithms often prioritize speed over absolute color accuracy, limiting the level of detail and fidelity possible. This inherent trade-off must be kept in mind when considering the outputs of these algorithms. Similarly, due to inherent uncertainties in the estimation process, AI systems can only provide a spectrum of probable colors, not definitive reproductions, especially in scenes with dynamic lighting conditions where color can drastically change.
When user input is sought for refinement, it introduces its own form of variability. Different users will likely have distinct aesthetic preferences, and thus provide differing suggestions for color, adding another layer of uncertainty and complexity to the process of objectively recreating the original colors. All of these aspects, combined, make accurately replicating historical color information a continuously evolving challenge for AI researchers.
The Science Behind AI-Powered Image Colorization How Algorithms Breathe Life into Black and White Photos - The Process of RGB Value Assignment in Grayscale to Color Conversion
The core of AI-powered image colorization hinges on the process of assigning RGB values to grayscale pixels. This involves algorithms intelligently estimating the original color based on patterns and features found in the grayscale image. These algorithms, often leveraging deep learning methods, learn from vast training datasets to establish connections between shades of gray and corresponding color information. The efficacy of this RGB assignment process is significantly influenced by the power of deep learning tools, especially convolutional neural networks (CNNs), which are remarkably skilled at discerning intricate details and patterns.
However, the process isn't without its limitations. Color perception, being subjective, poses a challenge for AI as the color choices made may not always perfectly reflect the original colors, potentially leading to variations in the perceived authenticity of the colorized image. The balance between automated color decisions based on learning and the incorporation of human creative input becomes critical in improving the colorization results and ensuring an aesthetically pleasing final output. This intricate interplay between artificial intelligence and human creativity continues to shape the evolving field of image colorization.
The process of assigning RGB values during the conversion of grayscale images to color relies heavily on the statistical relationships between grayscale intensities and the colors learned from vast datasets. These relationships, though useful for establishing general patterns, might not always align with our natural perception of color.
AI models often struggle to precisely assign colors in areas with intricate details. For instance, a pixel representing a textured fabric might receive a color that simplifies the variety of shades and patterns in the original, leading to a somewhat flattened and less nuanced visual representation.
Grayscale images frequently depict objects with cultural significance, and the meaning of those objects can be heavily influenced by cultural norms around color use. Thus, AI systems need to understand historical contexts to generate accurate color assignments. This is exceptionally challenging, as it requires them to disentangle centuries of color symbolism and its variation across societies.
When a grayscale image has multiple possible interpretations, the colorization process can easily fall into a trap. Without uniquely identifying features, AI algorithms are likely to rely on common color associations instead of a historically faithful approach. This leads to a colorization that's often generic rather than historically accurate.
Training datasets that lack a wide spectrum of colors can result in visually uninspired results. If a model lacks training examples from certain eras or cultural expressions, its colorizations might not accurately capture the intended richness of the original subjects.
Real-world lighting can drastically alter how we perceive colors, yet AI models often ignore these environmental cues. This can lead to visual inconsistencies, especially when dealing with scenes with dynamic lighting or shadow play.
While the goal of AI is objective analysis, the subjective nature of human color perception complicates things. Different people might disagree on what constitutes an "accurate" colorization, unintentionally influencing AI training data and the eventual outputs with individual biases.
Extending colorization to video presents a significant challenge due to the need for consistency between frames. Maintaining color accuracy as scenes and lighting change can easily lead to inconsistencies, especially in dynamic environments with motion.
Generative approaches like GANs illustrate the interesting dichotomy between creativity and interpretation that underlies AI colorization. These models are adept at creating realistic-looking results, but these results don't necessarily reflect the actual colors. This raises questions about the authenticity of the produced images.
Fundamentally, the core of assigning RGB values in AI colorization relies on educated guesses based on learned associations, rather than a direct replication of historical color information. This emphasizes the interpretive role that AI plays in artistic representation.
The Science Behind AI-Powered Image Colorization How Algorithms Breathe Life into Black and White Photos - Applications and Limitations of AI Colorization in Photo Restoration
AI colorization has significantly impacted the field of photo restoration, offering a way to breathe life into black and white images by automatically assigning colors to each pixel. Utilizing sophisticated deep learning methods like convolutional neural networks (CNNs) and generative adversarial networks (GANs), these algorithms can produce visually compelling colorized images, making the process accessible to a wider audience. This automation has democratized colorization, which was once limited to skilled artists.
However, AI colorization is not without limitations. Its reliance on learned patterns from training data can lead to subjective interpretations of color, potentially deviating from the actual colors in the original scene. This is particularly true when dealing with historical photos where color information may be scarce or uncertain. In these instances, the resulting colorization might not accurately reflect the original photograph, potentially altering the historical context and perceived authenticity of the image. Furthermore, the introduction of modern color conventions into older photographs can disrupt the established aesthetic and cultural interpretations associated with the original black and white.
The quest for accurate historical color representation remains a challenge in AI colorization, forcing a continuing discussion about striking a balance between the technological power of AI and the preservation of the original image's inherent qualities. As the technology continues to advance, there's a growing need to carefully consider how AI-powered colorization can be ethically used to enhance the understanding and appreciation of historical photographs without compromising their authenticity and historical context.
AI colorization, while a powerful tool, faces several limitations when applied to photo restoration, especially historical images. One key challenge is the inherent **ambiguity in color selection** that arises when images lack clear subjects or details. The AI, guided by its training data, might generate colors that don't accurately reflect the original scene, potentially distorting the historical record.
Furthermore, **cultural influences on color perception** present a hurdle. Color meanings vary across cultures and time periods, yet AI might struggle to capture these nuances. A colorization output may not align with the historical context or symbolism associated with the subjects within the image.
Adding to these challenges are the **features of historical images** themselves. Many older photos have inconsistencies due to the limitations of the photographic processes of their time, like uneven chemical development. This creates a gap between the original scene and the information captured in the grayscale image, making it difficult for the AI to accurately infer intended colors.
**Dynamic lighting conditions** found in historical photographs further complicate the task. Grayscale images often capture scenes with variable light, yet AI models might not always account for these environmental factors during colorization. This can lead to outputs that feel visually flat or do not reflect the actual lighting of the scene.
Similarly, **maintaining temporal consistency in video colorization** is a challenge. As scenes change and lighting conditions shift, keeping the colors consistent across video frames is complex. Rapid shifts can lead to disruptions and discrepancies that break the viewer's sense of continuity.
Another roadblock is the inherent **subjectivity of aesthetic preferences**. User feedback during colorization can differ significantly because individuals have different notions of beauty and color aesthetics. This makes it tough to arrive at a universally agreed upon "authentic" or "appealing" colorization.
The core issue is that AI relies on **prediction vs. reality**. Because AI uses learned associations to predict colors, the outputs often represent possibilities, not necessarily the definitive, original colors. The same input might lead to multiple colorizations, each reflecting the AI's subjective interpretation.
Moreover, the **limitations of training datasets** can impede accurate colorization. If the datasets used to train the AI don't include a broad range of historical colors and color palettes, the outputs might lack vibrancy or fail to represent the full richness of the original.
**Generative Adversarial Networks (GANs)**, though adept at producing realistic looking results, don't inherently ensure historical accuracy. While GANs improve realism through competition between networks, the produced colors might not reflect the original hues. This raises questions about the validity of AI-produced colors.
Ultimately, AI's role in colorization is largely **interpretive rather than purely technical**. It often makes educated guesses about color assignments based on learned associations, rather than basing it on concrete historical evidence. This signifies a blurring of lines between technological image enhancement and artistic interpretation.
More Posts from colorizethis.io: