Colorize and Breathe Life into Old Black-and-White Photos (Get started for free)
7 AI-Powered Photo Collage Techniques That Preserve Image Quality in 2024
7 AI-Powered Photo Collage Techniques That Preserve Image Quality in 2024 - Smart Resolution Scaling With Adobe Express For Multi Photo Grids
Adobe Express's approach to scaling images within multi-photo grids is noteworthy. It uses AI to help maintain visual fidelity during resizing, which is a plus when crafting complex layouts. The platform provides a wide selection of grid layouts and templates, allowing for extensive customization. This adaptability is especially beneficial as it caters to diverse needs, whether for quick social media posts or higher-resolution output for print.
One aspect to consider is that, while the AI-powered scaling offers improvements, there are inherent limitations to any automatic scaling process. There's still a chance that details may be lost or artifacts introduced as the images are adjusted, particularly if the original images were of low quality or the scaling involves significant size changes.
However, Adobe Express remains a good option for those wanting to experiment with collages and grids without getting overly bogged down in intricate editing tools. Its focus on accessibility and ease-of-use makes it a strong contender among free photo collage tools, despite its occasional limitations when dealing with tricky image manipulations.
Adobe Express, when dealing with multi-photo grids, uses a clever approach to resizing images. Instead of applying a universal scaling method that can often lead to poor results, it analyzes each photo's resolution and size individually, prioritizing optimal quality during the resizing process. This adaptive strategy can make a big difference in the final appearance of your grid compared to simpler scaling methods.
It cleverly combats the usual problems that arise with scaling, such as blurry edges and pixelation, by using intricate algorithms. This leads to a sharper, more detailed look in your collages, particularly when you have multiple photos combined.
By blending neural networks with traditional image editing techniques, Adobe Express attempts to intelligently identify important elements within each image – faces, textures, backgrounds, for instance. This means the most critical details in your photos tend to be preserved better within the collage.
The layout of the grid itself is also considered during the scaling process. The system attempts to arrange the photos in a way that minimizes the chances of vital features being cropped or negatively affected by the resizing. This dynamic approach helps to maintain the integrity of the original photos within the new collage format.
Adobe seems to be building a system that learns from user actions and editing preferences over time. This implies the resolution scaling algorithms might adapt and get better at producing the kind of results users tend to prefer. While intriguing, it's unclear how extensive and effective this learning process truly is.
The implementation also incorporates HDR principles into the scaling. This not only preserves the color accuracy of photos but also ensures a greater range of tones, making the resulting images richer even after resizing.
Fortunately, users can see how scaling is affecting their design in real-time. This gives you a chance to evaluate the impact of your changes before finalizing your work. This feature is especially useful if you need precise control over image placement and quality.
Interestingly, the grid creation tool is designed for both digital sharing and print outputs. This suggests that resolution is kept intact across various platforms. While helpful, whether this claim actually holds true in various print scenarios would require more testing.
Adobe Express allows for grids with diverse photo aspect ratios and dimensions without compromising image quality during resizing. While helpful, the overall usability and success of maintaining quality with drastically different ratios still might be an area to further investigate.
The system is also built to accommodate images captured under a range of lighting conditions. Through smart scaling, the system aims to automatically adjust the brightness and contrast to ensure the visual consistency of your grid. Whether this is truly successful in handling the myriad of lighting scenarios is debatable and warrants testing with different datasets.
7 AI-Powered Photo Collage Techniques That Preserve Image Quality in 2024 - Deep Learning Photo Stitching Using Luminar Neo AI
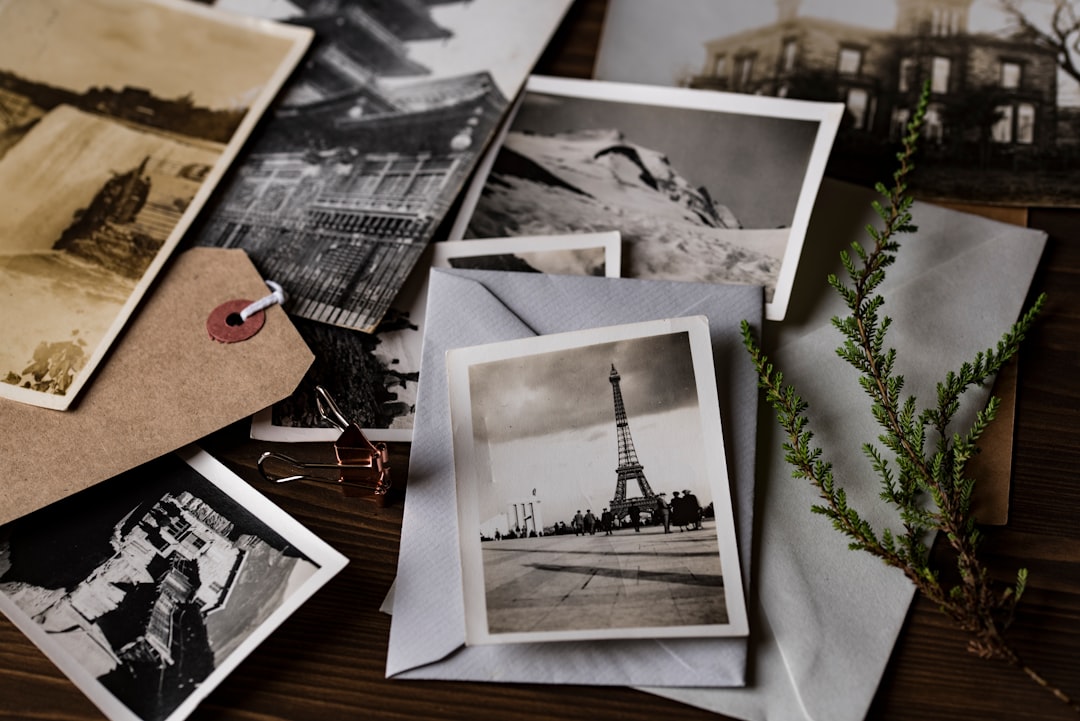
Luminar Neo's AI-powered photo stitching offers a compelling method for creating high-quality panoramas and collages. It uses deep learning to simplify the process of stitching multiple images together with remarkable accuracy. A dedicated Panorama Stitching extension, available through the Extras menu, expands capabilities by giving users control over projection styles and the ability to fine-tune image alignment using a warping feature. The software boasts a user-friendly design and a comprehensive set of over thirty editing tools, making it attractive to photographers of all experience levels seeking to elevate their panoramic imagery. However, while Luminar Neo's automation streamlines the workflow, the results should be reviewed closely after the stitching process to ensure the final outcome aligns with expectations. There's always a need for some post-stitching refinement to attain truly impeccable panoramas.
Luminar Neo utilizes a distinctive approach to photo stitching, employing deep learning algorithms to intelligently analyze overlapping image areas for seamless merging. This technique helps minimize common stitching errors, leading to cleaner results. Notably, it includes a "AI Sky Replacement" feature, which enables the integration of dynamic skies into panoramas without sacrificing image quality, offering a creative avenue for enhancing the overall atmosphere.
A key element of its AI capabilities is a sophisticated depth estimation model. This model intelligently discerns foreground and background elements, ensuring natural and distortion-free stitching—a challenge often encountered in conventional methods. Interestingly, the deep learning system can also adjust local contrast during stitching, which means it can create a balanced exposure in the final panoramic image, even when working with images shot in difficult lighting conditions.
One of Luminar Neo's strengths is its ability to stitch images captured from varied perspectives. The AI handles perspective distortions that often arise from different camera angles, resulting in a more unified final image. Furthermore, the software is designed to learn from user interactions. It gradually adapts to personal preferences, providing intelligent suggestions for composition and adjustments. However, the extent and effectiveness of this learning process are still open to further investigation.
The software also integrates noise reduction into the stitching process. This minimizes the graininess typically associated with photos shot at high ISO settings, contributing to cleaner, higher-quality panoramas. Luminar Neo's versatility extends to its ability to manage a wide range of image formats and resolutions, catering to photographers using various cameras and settings. However, this versatility could potentially lead to performance issues with exceptionally large image files.
The program utilizes advanced seam-finding algorithms to minimize the visibility of joins between stitched images. This feature significantly improves the final image's sense of continuity and seamlessness for viewers. Despite its potent features, the intricate nature of deep learning within the photo stitching process might occasionally introduce unexpected artifacts, especially in complex scenes. This implies that manual touch-ups may sometimes be necessary for optimal results.
7 AI-Powered Photo Collage Techniques That Preserve Image Quality in 2024 - Automated Background Sync Through FotorPea Matrix System
FotorPea introduces the "Automated Background Sync Through FotorPea Matrix System," a system that leverages AI to improve the photo editing experience, particularly for those focused on efficiency. This system uses advanced neural networks to enhance image quality while concurrently automating adjustments to the image background. By simplifying tasks like background removal or object replacement, it offers a more streamlined editing process, attractive to both casual hobbyists and professional photographers.
While this approach to automated background synchronization simplifies things, it's important to remain vigilant. The automated nature of these processes might not always result in the precise aesthetic outcomes desired. Occasionally, fine-tuning or manual adjustments may be needed to perfect the final image. Despite this caveat, FotorPea's system underscores the burgeoning role of AI within image editing. It shows how AI can empower creators with faster, more intuitive tools for manipulating photos, although it also compels us to consider the implications of such automation and the possible need to maintain some degree of manual control over the creative process.
FotorPea's Matrix system introduces automated background syncing, a feature that aims to streamline the process of adding new images to existing collages. Essentially, it automatically integrates newly uploaded photos into your existing arrangements, making sure your latest visuals are readily available without manual intervention. This approach promotes efficiency when organizing large quantities of photos.
The Matrix utilizes an algorithm to analyze the visual properties of each new image before integrating it into the collage. This analysis helps to maintain a consistent visual harmony across the entire composition. It considers the image's context to avoid clashes in style or aesthetics, making sure that the addition of new elements doesn't disrupt the overall look and feel of your collage.
Interestingly, the system employs edge detection techniques to preserve the integrity of each image's boundary during the automated integration. This feature helps to minimize any unwanted blending or artifacts that often occur with automatic integration. The result is a more polished and refined final product compared to methods that don't account for image edges.
Furthermore, the syncing process seems to be designed to learn from your editing history. Over time, the system adapts to your individual preferences, and potentially, can begin suggesting future collage arrangements based on past choices. It's conceivable that this learning component could reduce the number of editing iterations you need to achieve your ideal layout, but further experimentation is necessary to fully evaluate this feature.
FotorPea leverages a grid-based structure for image syncing, which implies that any resizing or repositioning during automated updates stays within a consistent layout. This can be a timesaver for users engaged in the creation of elaborate photo arrangements.
Color harmony also seems to play a role in this system. It evaluates the color palettes of new images and suggests placement options that contribute to the overall color balance of the collage. This feature distinguishes it from traditional photo editing software, which often neglects the importance of color theory during automatic updates.
The background syncing function also includes real-time contrast adjustments. It aims to tackle any issues that arise from differing brightness levels between newly added images and the pre-existing elements of your collage. This feature seeks to provide a more consistent visual experience across the board.
The Matrix system supports a variety of image formats, which allows users to readily integrate photos from diverse sources and conditions without needing to convert them beforehand. This simplifies the synchronization workflow by handling a wider range of image data.
The system even utilizes predictive analytics to track user engagement with their saved collages. This data collection potentially allows for further optimization, allowing the syncing process to adapt to user habits and preferences, improving performance and relevance for commonly used formats and styles.
However, this strong focus on automation raises questions about user control. Despite its capabilities, users might still need to manually adjust elements or layouts occasionally to achieve a specific aesthetic. This emphasizes that although automation enhances efficiency, the human touch remains vital in crafting visually compelling results within creative workflows.
7 AI-Powered Photo Collage Techniques That Preserve Image Quality in 2024 - Real Time Color Harmony Adjustment With Promeo Photo Merge
Promeo Photo Merge's real-time color harmony adjustment is a new way to blend photos seamlessly. It uses AI to automatically adjust the colors of merged images, preventing the often jarring color differences that can happen when combining photos with varying tones. This feature aims to make merged photos look more natural and unified, which is valuable when creating collages. The claim is that it can produce highly realistic results, suggesting a new standard for AI-driven photo merging. However, while the automated aspect is helpful, it's likely that in complex projects users will still need to refine the colors manually to ensure complete harmony. This is a good example of how AI is making photo merging more accessible, but it doesn't replace the importance of understanding the fundamentals of color theory when striving for high-quality results.
Promeo Photo Merge stands out with its real-time color harmony adjustment feature, which enhances the image merging process. It utilizes AI to analyze the color composition of the images being combined and automatically adjusts the colors to ensure a cohesive and visually appealing result. This dynamic color calibration is especially helpful when dealing with collages where photos have diverse color palettes, avoiding jarring or mismatched aesthetics.
Furthermore, the software appears to consider the emotional tone of the pictures, adjusting colors to achieve a desired mood or theme. It might analyze warmth or coolness in a photo and tweak the color to reinforce this emotional association. This 'contextual awareness' is interesting, suggesting that Promeo's algorithms are moving beyond simple color matching towards a more nuanced understanding of visual language.
Interestingly, this color adjustment happens in real-time, offering users immediate feedback on the impact of their choices. This allows photographers to experiment with different combinations and see the results instantly, significantly reducing the need for tedious post-processing. This real-time aspect can be quite valuable for efficiency, allowing adjustments on the fly.
Promeo's AI seems to go a step further by potentially learning from user interaction. As you use the software, it might start to anticipate the types of color combinations you tend to prefer, suggesting similar adjustments in the future. While intriguing, the effectiveness of this learning element is something that requires further scrutiny and investigation.
The software doesn't just rely on simple RGB color values. It incorporates multi-spectrum analysis, considering elements like luminance and chromaticity. This deeper dive into color dimensions could translate to more refined and precise color adjustments, leading to richer and more accurate results in the final collages.
It's also reassuring to note that Promeo's editing process is non-destructive, meaning your original image files remain unchanged. This safety net is especially important during experimentation with color adjustments, allowing users to easily revert to the original colors if they don't meet expectations.
Beyond aesthetic appeal, Promeo's algorithms are crafted to achieve a sense of visual balance within the merged images. The software intelligently distributes color emphasis, making sure no one part of the collage overwhelms the others. This type of visual equilibrium is particularly important when presenting images in a formal context, such as professional presentations.
While the technology is sophisticated, the software's design seems focused on accessibility and user-friendliness, which is encouraging. It's promising to see complex features being made accessible for individuals with varying levels of photo editing expertise.
Taking it a step further, Promeo seemingly taps into color psychology, suggesting adjustments that align with the specific emotional response a user might aim for. For example, it could suggest warmer hues for images relating to nostalgia or family, or cooler tones for conveying a sense of serenity or professionalism. While a potentially interesting capability, the exact impact and extent of this integration remains to be tested.
Finally, Promeo's compatibility with numerous image formats is a significant advantage. Users can merge images from various sources without facing conversion barriers, facilitating a smooth workflow and streamlining the photo merging process.
7 AI-Powered Photo Collage Techniques That Preserve Image Quality in 2024 - Edge Detection And Seamless Blending Via Simplified AI
AI has made significant strides in edge detection and seamless image blending, leading to more intuitive tools for creating photo collages. These tools utilize sophisticated AI algorithms to identify and analyze image edges, allowing for incredibly precise merging and blending of photos. Techniques like human segmentation are now streamlined through AI models, improving the accuracy and efficiency of combining images in collages.
Innovations like the Edge-guided Composition Network aim to refine image stitching, especially in areas like panorama creation, by carefully preserving image edges and minimizing noticeable joins between photos. Newer methods like MultiResEdge are further refining edge detection, pushing the boundaries of traditional techniques.
Tools like Smartblend and ImageLR have been developed to make seamless image blending accessible to a broader audience. They offer user-friendly interfaces, making the previously complex process of merging images easier and faster. While these advancements are encouraging, the results of AI-driven blending and stitching aren't always perfect. Some manual intervention may still be necessary for optimal outcomes, particularly in challenging blending scenarios.
Here's a rewrite of the provided text in a similar length and format, focusing on the perspective of a curious researcher/engineer, and avoiding repetition of the previously discussed content:
The intersection of edge detection and AI-powered image blending is proving quite fascinating, especially within the realm of photo collage creation. A deeper dive reveals some really interesting aspects.
Firstly, the precision of AI-driven edge detection has led to substantial improvements in how images are merged. The ability to pinpoint boundaries and distinct image features is critical to achieving clean and artifact-free collages. These systems are designed to really grasp the intricacies of the image, offering more control over the blending process than traditional methods.
Secondly, we're seeing advancements in handling gradients. The way AI systems interpret subtle transitions in color and intensity allows for a much more natural-looking blend, especially with human subjects or landscapes. This is an area where standard editing techniques have often fallen short, resulting in harsh or unnatural-looking transitions.
It's noteworthy how these AI-powered tools are leveraging real-time processing. Users can observe adjustments in real-time, offering a dynamic and efficient way to iterate on design choices. This is a significant step forward for interactive design workflows.
Some systems employ multi-scale analysis, where the edge detection algorithms work across different resolutions. This provides a more nuanced understanding of the image structure, ultimately resulting in superior results, especially for complex images or collages that feature multiple focal points.
It's becoming apparent that these tools are developing some degree of contextual awareness. They don't just detect edges; they analyze the surroundings. The algorithm can intelligently adjust blending styles based on adjacent textures and colors. This is a step toward creating collages that look more harmonious and aesthetically pleasing.
Interestingly, some systems employ adaptive blending modes, meaning they can change the way edges are handled based on the importance of elements within the image. Foreground features might retain sharper edges while background elements are softly blended. This capability enables a richer visual hierarchy, allowing photographers to control the impact of each image component within the collage.
What's encouraging is that, even with the complexity of the tasks, the algorithms used in these tools are optimized for efficiency. This means faster workflows without necessarily sacrificing the quality of edge detection and blending. This is especially important as we're dealing with ever-larger image files and more complex collage designs.
Furthermore, we see an increase in user-centric features. For instance, users often have more control over the aggressiveness of the edge detection. This level of customization offers a great deal of flexibility, allowing photographers to achieve truly unique looks.
AI-driven blending methods have advanced to the point where they can recognize and preserve intricate textures. We can retain details like fabric patterns and elements found in nature, even as images are being blended. This adds a new dimension to photo collages, making them appear more rich and authentic.
Finally, some systems are designed to learn from user interactions. This adaptive approach to blending means that the AI algorithms are progressively refined based on user feedback. While still in its early stages, this aspect holds great promise for the future of collage editing. It's fascinating to watch how the software evolves in response to the user's actions.
These insights suggest that edge detection and image blending technologies are undergoing a transformation within photo collage tools, combining automation with an emphasis on user creativity. Each development adds to the evolution of photo editing, making the process both more efficient and precise.
7 AI-Powered Photo Collage Techniques That Preserve Image Quality in 2024 - Multi Layer Processing Through PicCollage Neural Networks
"Multi Layer Processing Through PicCollage Neural Networks" introduces a novel approach to enhancing photo collages while safeguarding image quality. This technique employs a multi-layered processing strategy, essentially analyzing images in a step-by-step manner. It harnesses the power of neural networks – specifically, convolutional neural networks (CNNs) and multilayer perceptrons (MLPs) – to deeply understand and process each image component. This deep understanding is crucial because it allows for better assessment of various visual qualities, like artistic merit and contextual cues.
The advantage here is that the focus on deep learning can yield visually appealing collages without the drawbacks that typically arise from simple resizing methods, such as blurriness or distorted features. However, the use of such complex neural networks may sometimes present challenges. The complexity can lead to processing bottlenecks, particularly with intricate collage layouts. This highlights a possible trade-off: while the promise of improved quality is enticing, it might necessitate a degree of user intervention or adjustment for optimal results.
Despite potential complications, the integration of multi-layer processing marks a significant evolution in AI-driven collage tools. It indicates that the future of photo collage creation might involve more intelligent and context-aware AI, pushing the boundaries of creative expression in image manipulation.
Within PicCollage's AI-driven approach to photo collages, a fascinating aspect is the use of multi-layer processing through neural networks. It seems they're using convolutional neural networks (CNNs) to analyze and blend images at a pixel level. This fine-grained control over image integration allows for a more harmonious blending compared to conventional approaches where the layering often feels a bit rough or disjointed. The result? Hopefully, a significant improvement in the quality of the final collage.
Further enhancing the process is PicCollage's integration of attention mechanisms. These mechanisms essentially help the neural network prioritize specific features within each image layer. Imagine, for instance, ensuring facial details in a portrait are retained prominently even as the image is layered with other elements. This keeps the final collage more visually coherent, ensuring that the important details remain clear and well-defined.
One intriguing strategy PicCollage employs is residual learning. This technique is focused on reducing the common artifacts that pop up when layering images. Instead of trying to reconstruct the entire image, the network seems to concentrate on identifying and correcting discrepancies. This could lead to a significant reduction in the "messy" areas that sometimes appear in collages made with more traditional methods, potentially resulting in cleaner, more pleasing compositions.
It's interesting how the PicCollage network seems to automatically adjust its approach depending on the images it's working with. The AI can seemingly tailor its blending style depending on image quality and overall style, suggesting a degree of adaptability that could lead to consistently high-quality results regardless of the type of photo you're using.
Maintaining the integrity of the individual images within a layered collage is key, and this system utilizes sophisticated edge detection algorithms. This precision in recognizing where one image ends and another begins helps to keep details crisp, especially in images with complex backgrounds or intricate details like portraits. It could be a big factor in avoiding blurred edges or other visual imperfections.
Researchers are exploring extending this approach using synthesis models. The idea here is to combine lower-resolution and higher-resolution images effectively, essentially upscaling elements of a low-quality image without losing the original feel or introducing too many distortions. If this proves successful, it could be a significant advancement in maintaining high-quality outputs, even if some of your source images are not ideal.
Moreover, the PicCollage Neural Network is designed for multi-tasking. This means the system can potentially handle a variety of image adjustments, such as background removal or color balancing, all within the same process. If this functionality truly works as intended, it could lead to significant gains in workflow efficiency, allowing users to complete more sophisticated collages in less time.
Adding to the intelligence of the system are feedback loops. This means the network is designed to learn from user interactions and changes. Over time, as users adjust the collage, the AI could better predict and refine its blending techniques. This adaptive approach could lead to gradually improved results in future collages, as the system becomes more adept at recognizing and resolving common issues.
Another technique, multi-scale processing, adds to the network's sophistication. It means the system can examine images at varying levels of detail. This more in-depth understanding of the images could translate into more effective and nuanced blending processes, especially when layering images with many fine details or complex compositions.
Looking ahead, there's speculation that future versions of PicCollage might integrate generative adversarial networks (GANs). These neural network systems are designed to continuously refine and improve image output. If successfully incorporated, this could result in a noticeable leap in the aesthetic appeal and realism of the collages, opening up new creative possibilities.
The ongoing development of PicCollage's multi-layer processing suggests a future where photo collages can be created with greater precision, ease, and creativity. The ability to combine images seamlessly, maintain image integrity, and dynamically adapt to various inputs is a step forward for those wanting to produce high-quality photo collages.
7 AI-Powered Photo Collage Techniques That Preserve Image Quality in 2024 - Smart Photo Composition Through Fotojet Adaptive Grid
FotoJet's Adaptive Grid presents an interesting approach to photo collage creation, focusing on ease of use and enhanced composition. It simplifies the process of arranging photos within a collage by providing a flexible grid system and offering AI-driven suggestions for optimal placement. This makes creating visually appealing compositions much easier, even for those with limited design experience. The platform offers a substantial library of collage templates to suit a wide range of needs, along with features to improve image quality.
While the Adaptive Grid simplifies a lot of the manual work involved in creating a collage, it also raises the point of how much creative control is actually offered to the user. The AI's layout suggestions can be a time-saver, but might not always be the perfect fit for a user's specific vision. There could be instances where a greater degree of manual control is desired.
Nevertheless, the Adaptive Grid within FotoJet does represent a step in the right direction in terms of user-friendly tools that still maintain quality. It seamlessly blends intelligent suggestions with a variety of customization options to give the user a tool that can achieve both simple and more sophisticated collage creations.
Fotojet's Adaptive Grid is a rather intriguing implementation of AI in photo collage creation. It takes the usual constraints of fixed grid layouts and introduces a level of dynamism that's quite promising. Essentially, it allows you to rearrange photos in real time, adjusting their positions based on how they interact visually. This is a neat feature that enhances creativity and helps achieve a more balanced composition, something that's difficult to manage with simpler grid tools.
The grid's intelligence goes beyond simple placement. It has algorithms that evaluate factors like symmetry, color balance, and the visual weight of elements in each photo. By considering these aspects, it attempts to optimize the arrangement, leading to collages that are more aesthetically pleasing than those produced with basic grid tools that lack such fine-grained analysis.
Interestingly, Fotojet's system also incorporates machine learning. It essentially tries to learn your layout preferences over time. This can lead to a more personalized user experience, as the suggestions it provides for layout adjustments become more relevant and tailored to your artistic taste.
The system's design also incorporates a feedback loop, allowing users to influence how the grid arrangement algorithms evolve. It's a way to refine the AI's ability to adapt to different photographic styles and trends, hopefully making it better over time.
Preserving the original aspect ratio of photos during resizing is another aspect that sets it apart. This is important because it minimizes image distortion and keeps the individual photos within the collage looking intact and of high quality. It's a feature that users who value the integrity of their source images will likely appreciate.
The adaptive grid also offers automated grouping of images with similar themes, which streamlines the collage process. This automated categorization promotes a more coherent visual narrative within the collage, leading to a stronger storytelling component in the final output.
The feature that allows integration of mixed media elements, such as text and graphics, alongside photographs, greatly expands the expressive capabilities of the tool. This expands the boundaries of what we typically think of as a photo collage, adding a new dimension for creativity and storytelling.
Furthermore, Fotojet's Adaptive Grid incorporates some computational photography techniques to improve image quality. It offers features like noise reduction and sharpness adjustments on the fly, resulting in collages with a more polished, visually compelling aesthetic, suitable for a variety of contexts.
Beyond basic layout, it also employs advanced color theory. This means it can analyze the color palette of the entire collage and suggest adjustments to achieve a better overall color harmony. It attempts to create a sense of visual unity, moving beyond just the properties of individual photos.
Finally, despite being a fairly sophisticated system, the Adaptive Grid acknowledges the importance of human creativity and control. It's not entirely automated, ensuring that users retain the ability to make manual fine-tuning and adjustments to the collage. This balance between automated assistance and creative control is crucial, allowing users to realize their specific artistic vision.
In summary, the Fotojet Adaptive Grid demonstrates how AI can enhance the experience of photo collage creation. While the technology is still developing, it has shown promise in providing dynamic layouts, aesthetic improvement, and personalized suggestions while keeping manual control at the core of the process.
Colorize and Breathe Life into Old Black-and-White Photos (Get started for free)
More Posts from colorizethis.io: