7 Critical Factors Why AI Song Extension Tools Like SunoAI Are Reshaping Voice Production Workflows
7 Critical Factors Why AI Song Extension Tools Like SunoAI Are Reshaping Voice Production Workflows - Voice Cloning Integration Makes Custom Vocal Tracks Ready in Under 4 Minutes
The integration of voice cloning into audio production workflows has brought about a significant shift. Now, crafting custom vocal tracks can be accomplished in a remarkably short timeframe, often within four minutes. This accelerated process not only streamlines production but also empowers creators with unprecedented agility. They can experiment with various vocal styles and intricacies, pushing the boundaries of their creativity with a newfound efficiency.
The rise of AI-powered tools like SunoAI has made it easier than ever to create high-quality, lifelike vocal sounds across a range of applications. Whether it's composing music, producing podcasts, or narrating audiobooks, these technologies have democratized access to professional-grade vocal capabilities.
The ongoing development in voice cloning ensures the expansion of readily available voices, presenting a wealth of options for creators to explore. This continuous evolution ensures that the efficiency of production workflows isn't compromised while also enabling an enriched palette of creative potential. There are still limitations and challenges inherent in these processes, such as the occasional glitches in capturing the nuances of human expression, which remain a key area of development. However, the potential impact on audio production is immense and we're likely to see it increasingly integrated into our media consumption in the near future.
The integration of voice cloning into audio production workflows has become remarkably efficient, with the ability to generate custom vocal tracks in under four minutes. This rapid turnaround is achieved through sophisticated algorithms that rapidly analyze and replicate the intricacies of a human voice, capturing subtle nuances like emotion and tone. We're now seeing the emergence of AI tools specifically designed for extending song segments and crafting unique vocal parts, a process that previously demanded significant time and resources.
Imagine a podcast creator needing a specific voice for a character or a narrator for an audiobook. Instead of scheduling and coordinating with a voice actor, they can quickly generate a high-quality voice clone with minimal audio input – as little as three minutes in some cases. This process is not only expeditious but also versatile. Platforms like ElevenLabs showcase the meticulousness of modern voice cloning, leveraging deep learning to replicate the characteristics of any voice with great precision. Moreover, the increasing availability of multilingual voice clones broadens the scope of applications for these technologies, enabling content localization across various markets effortlessly.
It's fascinating how the range of options in voice libraries continues to expand as new voices are added. And this extends to music production as well. The ability to craft unique, royalty-free AI vocals provides artists with a readily available creative tool for exploring novel musical styles without the complexities of navigating copyright issues. Some AI tools like Soundverse or Vocalize emphasize user-friendly design, allowing users to readily generate vocal tracks with straightforward text-to-speech functionalities.
While still evolving, these technologies are not limited to just replicating speech. Tools like Resemble AI showcase their potential for immersive audio experiences in gaming, film, and marketing through high-fidelity text-to-speech capabilities. Furthermore, the ongoing research and development into AI voice generation constantly seeks to enhance the quality and realism of the synthesized voices, inching closer to indistinguishable simulations of natural human vocalizations. As a result, voice cloning is steadily becoming a more viable component of professional music production and a broader spectrum of creative projects.
7 Critical Factors Why AI Song Extension Tools Like SunoAI Are Reshaping Voice Production Workflows - Neural Networks Now Support Multi Language Voice Generation Beyond English
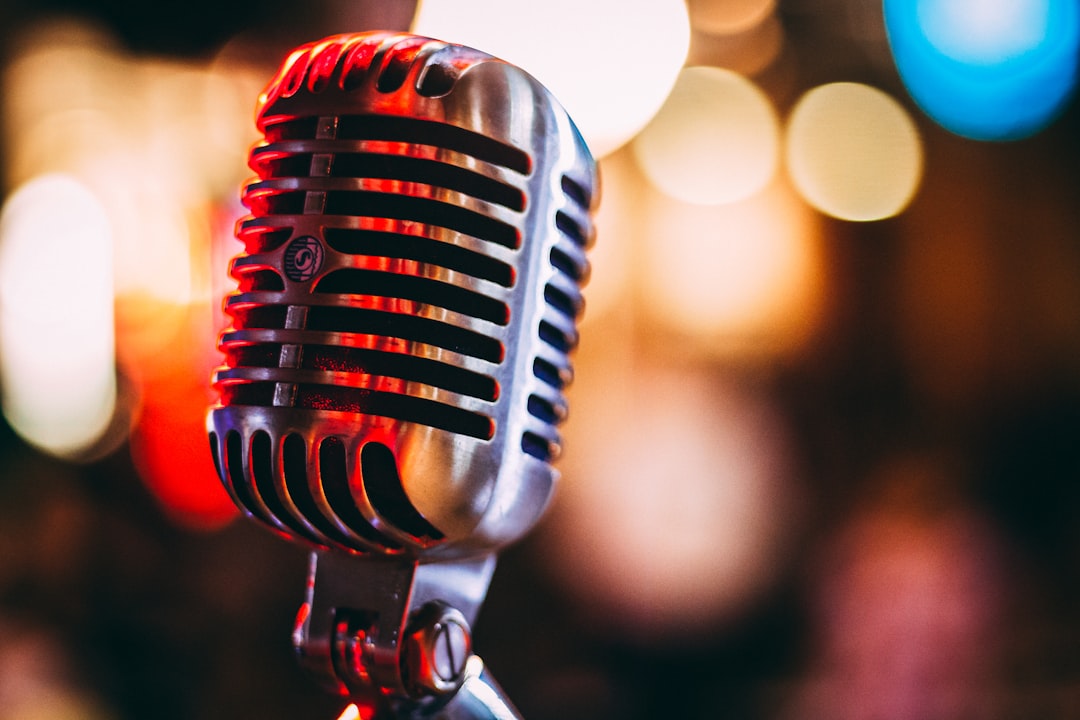
The ability of neural networks to generate voices in multiple languages represents a significant leap forward in sound production. We're no longer limited to English; voice creation now extends across over 140 languages and dialects. This development offers an exciting array of options for audio professionals, whether crafting audiobooks, podcasts, or other audio content. The availability of hundreds of neural voices allows creators to generate audio that feels more natural and conveys emotions effectively. Companies can now create branded audio experiences specifically tailored to different language groups.
Recent progress in deep learning has demonstrably enhanced the accuracy and realism of these AI-generated voices. The quality of synthetic speech is improving constantly, making it increasingly challenging to differentiate from human speech. This expanding field of possibilities opens doors for creators to explore unique auditory landscapes across a wide range of styles and languages. While still developing, the tools are becoming more robust and are proving invaluable for various creative endeavors. We can expect to see more refined and sophisticated audio experiences emerge as this technology matures.
Neural networks are now capable of generating synthetic speech in a wide range of languages, expanding beyond English to over 140 languages and regional variations. This opens up exciting possibilities for audio productions like audiobooks, podcasts, and even music, where content can be easily adapted for global audiences without relying on multiple voice actors for each language.
These advances in deep learning are allowing for not just the basic replication of sounds and accents, but also the capture of more subtle cultural elements and regional dialects within the generated speech. This increased accuracy can contribute to more authentic and engaging audio experiences, especially in scenarios where cultural context is important.
Interestingly, it's becoming possible to achieve this multilingual capability with a single, well-trained neural network, rather than having separate models for every language. This centralized approach is likely to simplify the development of new audio production tools designed for global markets. One intriguing aspect of this is the potential to blend characteristics of different languages to create completely novel voice designs. This could be a powerful tool for creative industries looking to appeal to broader audiences.
Beyond simple text-to-speech, we're seeing the integration of these multilingual voice models into interactive applications like video games and virtual reality. Here, they can greatly enhance user experiences through more engaging, language-specific dialogue and narratives.
A key part of this evolution has been the incorporation of attention mechanisms into the neural network architecture. These mechanisms help the models focus on the most relevant portions of the input text, allowing for more accurate and natural-sounding output. However, challenges remain. Ensuring that the delicate details of intonation, rhythm, and stress patterns specific to each language are accurately captured is a complex area still requiring further development.
Nevertheless, with these developments, content creators have a powerful new tool at their disposal. They can now translate their audio content – not just the words but also the emotional nuance and intended context – into multiple languages with relative ease. This can significantly increase audience engagement and create a more relatable experience for listeners across the globe.
Recent advances in vocal tract modeling have enabled speech synthesis systems to capture finer details of human voice quality, such as breathiness or resonance. These aspects are crucial for making synthetic voices sound more realistic and natural, regardless of the language they are speaking.
While the potential of multilingual voice generation is exciting, we also need to consider the ethical implications. Voice cloning, specifically, raises concerns about consent and potential misuse. As the technology matures, it will become increasingly important to establish clear ethical guidelines and standards to ensure responsible use in media production and beyond.
7 Critical Factors Why AI Song Extension Tools Like SunoAI Are Reshaping Voice Production Workflows - Machine Learning Algorithms Enable Real Time Voice Modification During Recording
Machine learning algorithms are fundamentally changing how audio is produced by allowing for on-the-fly voice alterations during recording. This is a powerful development, enabling smooth shifts in vocal qualities without compromising the original spoken content or the subtleties of human emotion. It's now possible to achieve things like instantly altering a voice's tone or texture while preserving its natural rhythm and speech patterns. Tools such as StreamVC demonstrate the potential for near-instant voice conversion with minimal impact on the recording, making them valuable for diverse audio production – whether it be crafting podcast episodes or narrating audiobooks. Moreover, advancements in real-time voice cloning mean a minimal sample of a person's voice is now needed to create a believable copy, dramatically cutting down the time and resources needed for voice training. The possibilities offered by these evolving algorithms are impressive, particularly when considering interactive storytelling or tailored audio, but fully capturing the nuanced richness of human expression remains an ongoing challenge and area for development.
The integration of machine learning algorithms into the recording process is enabling real-time voice modification, a capability that's transforming how we approach sound production, particularly in fields like voice cloning and audiobook creation. These algorithms can instantly adjust a voice's pitch, tone, and modulation during recording, which allows voice actors and podcasters to experiment dynamically, eliminating the need for extensive post-production tweaks.
One intriguing aspect is the ability of these models to analyze the emotional nuances embedded in a speaker's voice. They can then modify the voice while preserving the original emotion, a key feature for enriching audiobooks with expressive narration and for giving podcasts characters more lifelike voices. Interestingly, some algorithms even automate the harmonization of vocal tracks as they're recorded. This simplifies the process of producing intricate musical arrangements, a feat that previously relied on expert musicians or complicated software.
The development of deep learning techniques has resulted in more human-like voice modifications. By training on massive datasets, these algorithms are able to produce outputs that are remarkably coherent and incredibly close to the natural human voice. Additionally, creators can now build custom voice profiles, tailoring them to specific characteristics such as age, gender, accent, and even emotional inflection. This level of personalization enables diverse audio experiences for different applications.
Furthermore, the optimization of machine learning techniques is leading to reduced latency, which is critical for real-time feedback during live events or streaming scenarios. It's notable that these systems easily integrate with existing software, streamlining workflows and fostering a more agile approach to audio production. It's fascinating to see how they can leverage voice cloning techniques to generate a variety of voices from a single recording, which is perfect for producing a cast of characters or narrators in an audiobook with minimal effort.
In essence, these algorithms lessen the cognitive load for creators by automating a significant part of the voice adjustment process. This allows them to focus on developing content and narrative instead of getting bogged down in intricate sound manipulation. Some systems also include feedback loops to evaluate and improve voice synthesis quality in real-time. This ensures the modifications meet pre-set quality standards, thus improving the overall quality of the audio production.
While these algorithms are rapidly developing, there are always tradeoffs and challenges to consider. Finding the right balance between real-time modification and the nuanced emotional range of the human voice is an ongoing area of research. There's also the need to continually assess and refine the ethical implications of technologies that are capable of manipulating human voices. Nonetheless, real-time voice modification tools are evolving at a swift pace, shaping the audio landscape by presenting more creative control and options for artists and content producers in numerous applications.
7 Critical Factors Why AI Song Extension Tools Like SunoAI Are Reshaping Voice Production Workflows - Automated Batch Processing Reduces Audio Book Production Time by 60 Percent
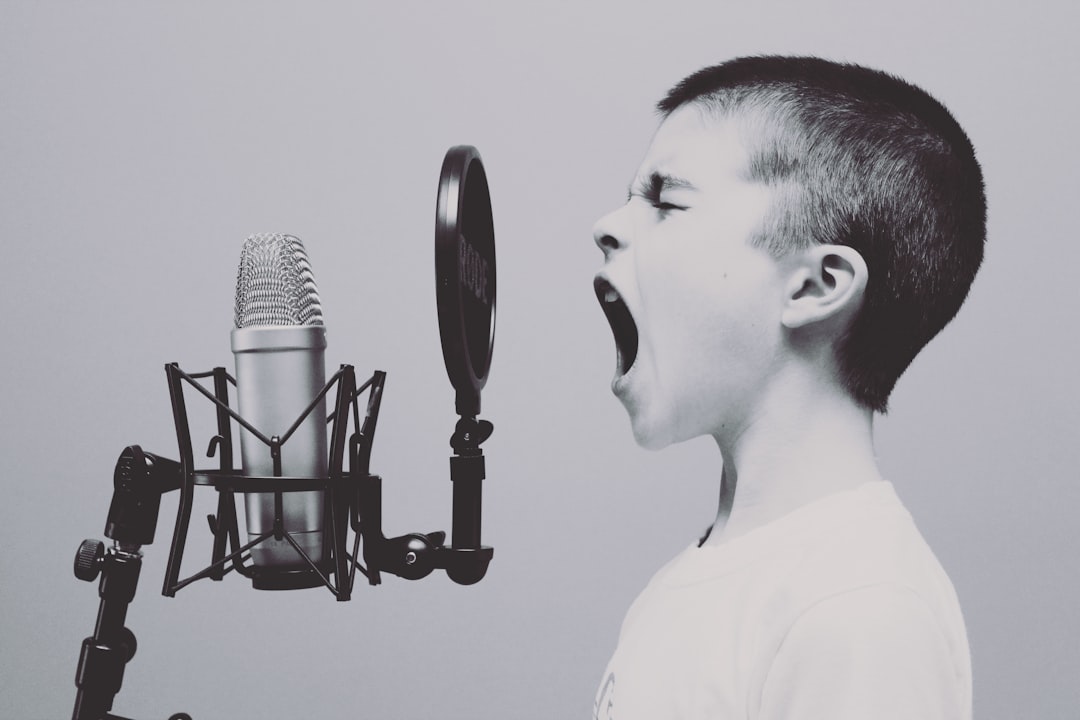
Automated batch processing has significantly impacted audiobook production, leading to a substantial 60% reduction in overall production time. This advancement streamlines the various steps involved in audio production, making the process much faster and more efficient. By automating repetitive tasks, creators can allocate more of their time and resources towards the creative aspects of audiobook production, leading to a more focused and productive workflow. The increased speed of production also translates to shorter project completion times, a major benefit in today's fast-paced content creation landscape. This increased efficiency potentially unlocks new opportunities for creativity and innovation within the realm of audiobooks. The capacity for rapid processing and manipulation of audio files allows creators to experiment more freely with different elements, fostering a more dynamic and exciting audiobook production process. While the field of automated batch processing is continuously evolving, its current impact on audiobook production is considerable. It is likely that this technology will continue to shape how audiobooks are developed and delivered in the future.
Automated batch processing has emerged as a game-changer in audiobook production, dramatically reducing production times by up to 60%. This improvement stems from the ability to streamline processes and handle multiple audio segments concurrently. It's fascinating how this approach minimizes the variability that can occur when different voice actors are involved or when manual adjustments are made, ensuring a consistent vocal quality across the entire audiobook. This consistency plays a crucial role in maintaining narrative integrity.
The effectiveness of these automated systems is driven by the analysis of massive datasets containing a wide array of vocal characteristics. By leveraging machine learning, the systems can continually adapt and refine their voice cloning capabilities to more accurately match desired vocal attributes. However, the implications extend beyond just audiobooks. We're seeing the benefits of batch processing applied to podcasting and even music production, indicating a broader potential impact on audio creation across numerous media.
Beyond the time savings, these systems frequently incorporate advanced audio effects and enhancements directly into the production pipeline. This approach results in a more polished final product that seamlessly integrates dynamic soundscapes and background elements, enhancing the overall listening experience. It's impressive how customizable these tools are. Content creators can set parameters for a batch of audio tracks—factors like tone, pitch, and pacing—with minimal manual input, which simplifies large-scale adjustments.
Furthermore, these systems often incorporate smart algorithms that deliver real-time feedback on audio quality during the recording phase. This feature empowers producers to make quick adjustments on the fly, ensuring that the final product consistently adheres to pre-defined quality standards and minimizes the need for extensive revisions. It's clear that as demand increases, automated batch processing offers a flexible and scalable solution capable of handling greater workloads without compromising audio quality. This adaptability is a major benefit for production studios seeking to expand their capacity or accelerate project delivery times.
The refinement of algorithms used in these automated tools has also allowed for a more accurate representation of intricate voice features like breathiness and resonance. This level of detail enhances the realism of synthesized voices, ensuring they more closely mirror the emotional nuances present in the original speech, ultimately enriching the storytelling experience. Moreover, creators can explore the inclusion of interactive elements in audiobooks using automated techniques. These interactive aspects, such as variable narration styles or character voices responsive to listener choices, offer a novel path forward in audiobook narrative design. While the technical advancements are impressive, the impact on creative expression is truly notable.
7 Critical Factors Why AI Song Extension Tools Like SunoAI Are Reshaping Voice Production Workflows - Advanced Natural Language Processing Improves Emotional Voice Range Generation
The use of advanced Natural Language Processing (NLP) is transforming how we generate voices, particularly in enhancing the range of emotions they can convey. NLP techniques allow for more refined analysis of emotional cues within spoken language, enabling AI-generated voices to more accurately reflect human emotional expression. With the ongoing development of voice cloning and synthesis, we're seeing the implementation of sophisticated algorithms that can adjust vocal characteristics on the fly to better communicate various emotions and moods. This is proving incredibly useful for a variety of applications, such as audiobook narration or character voices in podcasts. While this expansion of possibilities is beneficial for voice actors and content producers, it also highlights a persistent challenge: finding the ideal balance between the precise technical aspects of voice manipulation and the complex, nuanced tapestry of human emotion that makes audio experiences truly captivating. Through ongoing development, the combination of NLP and audio production has the potential to shape immersive and emotionally resonant auditory experiences. However, there's still much work to be done in ensuring these AI-generated voices authentically and naturally capture the multifaceted spectrum of human emotion.
The integration of advanced Natural Language Processing (NLP) is significantly altering how AI generates voices, particularly in its ability to capture and reproduce emotional nuance. NLP, the field focused on enabling computers to understand and process human language, is now able to analyze not just the literal words but also the underlying emotions embedded within them. This capability has led to a surge in the development of voice synthesis systems that can generate a wide spectrum of emotional tones.
Think of how a human voice can convey happiness, sadness, or anger through subtle variations in pitch, tone, and rhythm. NLP models are now learning to identify these cues and translate them into corresponding modifications in synthetic speech. This means that synthesized voices can now go beyond simply reading lines from a script and actually convey the emotions intended by the author or creator. This is a substantial step forward for creating more engaging and relatable audio content, whether it's for podcasts, audiobooks, or other audio-based applications.
One key aspect is the ability of NLP to capture phonetic nuances associated with different emotions. For example, a question asked with excitement will have a different pitch and timbre than a question asked with uncertainty. By learning these subtle patterns, voice generation systems are able to create a more realistic representation of emotional expression. Furthermore, this capability isn't limited to English. Ongoing research is focused on enabling NLP models to accurately reflect emotional differences across a diverse range of languages, a fascinating challenge given that emotional expression can vary significantly between cultures.
Another exciting development is the capacity to craft customized emotional profiles for AI voices. Imagine being able to fine-tune a synthesized voice to have a naturally cheerful tone or a more somber delivery. This feature empowers creators to choose the voice that best fits the narrative or atmosphere of their audio project. This ability to tailor the emotional character of a voice opens doors to a wide array of creative possibilities.
However, we're not just limited to pre-defined emotional states. Machine learning algorithms are now capable of making real-time adjustments to the emotional tone of a voice based on the content being narrated. This allows for truly dynamic storytelling, particularly in interactive scenarios. For instance, in an interactive audiobook, a character's voice might shift from a hopeful tone to a more apprehensive one based on the listener's choices.
Further advancements in NLP allow for data-driven personalization of emotional expression in synthesized voices. By tracking user preferences and interactions with AI-powered audio, systems can tailor voices to match individual listener's emotional tastes, which is a fascinating prospect in the domain of podcasting, where creating a unique and engaging experience for each listener is paramount.
It's also worth noting that researchers are exploring ways to layer multiple emotional tones within a single voice passage. This is a challenging but promising area because it mirrors the natural complexity of human speech, where we often transition between emotions within a single sentence or phrase. This technique can significantly enhance the listening experience in narrative-driven projects.
Another critical aspect is the automated detection of emotional intent within scripts. NLP models are becoming adept at identifying the emotional context of text and then selecting the most appropriate voice modulations to ensure the delivery matches the intended emotional impact. It's an ongoing process of refinement, but the potential for seamless integration of emotion and language is quite remarkable.
Furthermore, the field of voice cloning is evolving to include not just replicating a voice's sound but also its unique emotional characteristics. Imagine being able to capture the emotional range of a specific narrator and then use that to generate a synthetic voice with the same distinctive emotional signature. The applications of this for preserving the personality and style of narrators in audiobooks or podcasts are quite compelling.
These advancements are all fueled by the integration of machine learning into NLP systems. By training these models on extensive datasets of emotionally charged speech, we're moving closer to AI voices that can not only understand language but also express a broad spectrum of human emotion with increasing accuracy. As these models continue to improve, we can expect to see even more natural and emotionally nuanced AI voices emerge across a range of audio production workflows, including voice cloning.
The field is constantly evolving, with new and exciting developments continually emerging. As we delve deeper into the complexities of human language and emotion, we are undoubtedly on the cusp of witnessing a new era in audio content creation where AI-powered voices not only inform but also truly engage with listeners on an emotional level.
7 Critical Factors Why AI Song Extension Tools Like SunoAI Are Reshaping Voice Production Workflows - New Dataset Training Methods Enable Studio Quality Voice Synthesis from Home
Recent advancements in training methods for AI voice models are enabling the creation of incredibly realistic, studio-quality voices from home. This is made possible by new datasets and improved machine learning techniques that allow for more precise voice cloning. The result is a significant leap forward in the ability to generate voices for audiobooks, podcasts, and even music production. Tools like neural network-based singing voice synthesis (NNSVS) are particularly noteworthy for their ability to create expressive and diverse voice characteristics. This accessibility to high-fidelity vocal synthesis empowers content creators to easily incorporate compelling voice performances into their work. Yet, there's a constant need for improvement, especially in capturing the subtle emotional expressions that make human voices so unique and engaging. As this technology continues to evolve, it will be fascinating to see how well it can continue to bridge the gap between the artificial and the authentic in the realm of sound design.
Recent breakthroughs in voice synthesis have made it possible to generate distinctive audio from a remarkably small amount of source audio, sometimes needing as little as three minutes of recorded speech. This offers flexibility for voice actors, as they can produce diverse vocal variations with minimal input, thereby extending their creative output.
Emerging technologies in voice synthesis are starting to detect and replicate emotions in real-time during audio production. By examining subtle variations in speech patterns, these systems generate voices that react dynamically to the emotional context, leading to a more authentic feel for voiceovers in audiobooks or podcasts.
The training datasets for voice synthesis algorithms often contain extensive amounts of recorded speech, often totaling thousands of hours. This thorough process allows synthesized voices to achieve remarkable fidelity by capturing minute tonal fluctuations and subtle nuances, which are key to creating credible and relatable voice clones.
Interestingly, current voice generation systems can now blend vocal characteristics from multiple sources. This ability makes it possible to construct unique voice profiles by combining traits like pitch, accent, and emotional tone, thus offering creators a larger palette of voice options.
The quality of AI-generated voices has reached a level where it's frequently difficult for listeners to differentiate between human and synthetic speech. This raises intriguing questions concerning authenticity and reliability in audio productions, particularly in contexts like storytelling or news broadcasts.
Machine learning algorithms are not only adept at producing high-fidelity voice clones but also allow for extensive customization of vocal characteristics such as age and emotional inflection. This granular control lets creators target specific audience demographics or niche markets, potentially enhancing engagement with audio content.
Attention mechanisms play a key role in optimizing neural networks for voice synthesis. These frameworks enhance the network's ability to focus on critical parts of the input data, resulting in more lifelike vocalizations that retain the typical emotional flow of human speech.
Automated batch processing has transformed audiobook production by enabling multiple versions to be produced concurrently. This streamlined approach considerably decreases production time and minimizes inconsistencies that might arise from combining different recording sessions.
Researchers are now creating AI systems that learn from real-time interactions, adapting the emotional tone of a voice in response to audience feedback. This dynamic element could change the way we create interactive narratives in podcasts or audiobooks.
Despite significant advancements, capturing the full spectrum of human emotional expression in synthesized speech remains a challenge. The complexities of intonation, rhythm, and emphasis patterns found in different languages present a research frontier requiring continued exploration and innovative solutions.
7 Critical Factors Why AI Song Extension Tools Like SunoAI Are Reshaping Voice Production Workflows - Cross Platform Voice Model Compatibility Simplifies Post Production Workflow
The ability of voice models to work seamlessly across different audio platforms is revolutionizing post-production. This cross-platform compatibility means that creators can utilize a wider range of voice models without needing to adjust their tools constantly. This simplification is particularly beneficial for projects involving audiobooks, podcasts, and music where various voices and effects might be employed.
Tools like SunoAI are starting to incorporate this compatibility, leading to smoother workflows for tasks like voice cloning and synthesis. Sound designers, dialogue editors, and content creators can collaborate more easily because the underlying tools and voice models play nicely together.
But there are still hurdles. Replicating the subtleties of human expression consistently across different platforms remains a work in progress. This requires continued effort to refine and innovate within voice technology.
Despite these challenges, the landscape of sound production is shifting. This enhanced flexibility and accessibility makes experimenting with different voices and sounds more straightforward, offering improved possibilities for crafting captivating stories and enriching audio experiences.
The ability to use a single voice model across various audio production platforms, like podcasting and audiobook creation, has made workflows noticeably more streamlined. It used to be that if you wanted a certain voice across multiple projects, you would either have to use multiple voice actors, or risk the inconsistency that could come with using different models. Now, thanks to a greater degree of cross-platform compatibility, a single, custom-generated voice can be used across platforms. This saves time for the producer as they don't have to coordinate with multiple voice actors and ensures the sonic experience remains consistent, no matter where a listener encounters a piece of audio content.
The ability of modern neural networks to fluidly shift between vocal styles, accents, or tones offers newfound flexibility for creators. Imagine a sci-fi audiobook, where the protagonist has a distinctive alien speech pattern but still needs to converse in human-like tones at times. Neural network-based voice generation can handle these transitions with ease, allowing for diverse and dynamic vocal performances without the need to switch between different voice actors or undergo laborious post-production editing. It also saves time and resources that would have been dedicated to coordinating multiple voice actors with distinct styles.
AI-generated voices have become incredibly adept at emulating the complexities of human vocalizations. The ability to replicate vocal nuances like breathiness or warmth has elevated the believability of synthesized speech, a crucial step in creating engaging and natural-sounding audio. This is particularly important in narratives, where the voice plays a significant role in conveying the emotion and atmosphere of a story. The ability to recreate these features, without the involvement of a human speaker, is significant, and may well lead to questions about the need for human vocalists in some contexts in the future.
Emerging technologies are also allowing AI to understand and adapt to the emotional context of the narrative. In real-time, these algorithms can modify a voice's tone or inflection to reflect the changing emotional landscape of a story. This dynamism adds another layer of depth to the listening experience, enhancing the impact of audiobooks, for example, or allowing characters in a podcast to express emotions more naturally. The greater the realism, the more immersive the experience for the listener, and the more useful this technology will become for content creators.
Continuing this line of thought, new algorithms give producers the ability to rapidly generate various variations of a script with different emotional undertones or vocal styles. This offers incredible flexibility for producing custom versions of audio content tailored to specific audience demographics or genres. It's fascinating to think that a single story could be crafted into a multitude of variations by adjusting the voices and tone to target specific listeners. Whether it's for young children, or those more accustomed to audiobooks with a faster narration pace, these custom variations offer the potential for significantly more audience engagement.
While previously extensive voice libraries were needed, high-quality voice generation is now feasible with only a minimal amount of input audio, sometimes as little as three minutes. This has leveled the playing field, as content creators no longer need vast voice libraries to achieve a high level of audio quality. This could be particularly relevant for independent content producers, or small companies seeking to create podcasts or audiobooks without substantial upfront investments.
Using advanced techniques like attention mechanisms, voice cloning tools are increasingly adept at identifying and isolating the key nuances in human speech patterns. This results in outputs that are smoother and more natural, demonstrating greater fluency and coherence, a crucial step towards synthetic voices that sound genuinely human. By isolating specific features of human speech, it becomes possible to replicate voices in an almost indistinguishable fashion. It will be interesting to see where this goes in the future as the fidelity of the replicas increases.
Multilingual capabilities in AI voices have expanded beyond the basic ability to simply translate text to speech. They are now able to capture regional dialects and cultural variations in intonation, cadence, and pronunciation. This opens the doors for creators to produce localized audio experiences that feel authentic to diverse global audiences. The more immersive these localized audio experiences are, the more the listener may feel connected to the content, leading to greater overall consumption of audio content across diverse languages and cultures.
One of the most fascinating breakthroughs is the ability to merge characteristics from different voice sources into entirely new and unique vocal profiles. This allows creators to blend vocal qualities in new ways to achieve specific creative objectives for characters in audiobooks or podcasts. We might be able to use the soft tones of one voice and the gravelly character of another to create a voice that fits a certain personality or role, which in turn can make it more engaging for the listener.
While the advancements in voice AI are remarkably impressive, there's a critical need for ongoing discussion and development around ethical considerations. Specifically, the power of voice cloning creates the need for guidelines and standards that can prevent malicious use. Given the nature of the technology, safeguards and best practices need to be constantly reassessed to ensure the appropriate use of these powerful tools, especially with regards to consent, potential misuse, and deception. These are important points to consider as the technology develops and becomes more accessible to the public.
These factors suggest that AI-powered voice generation is becoming an increasingly important part of the audio landscape, revolutionizing how we produce, consume, and interact with audio content. However, a healthy dialogue and a commitment to ethical development will be necessary to ensure that these powerful technologies are used in a manner that benefits both creators and listeners.
More Posts from colorizethis.io: