7 Key Differences Between AI-Powered and Traditional Online Photo Editors in 2024
7 Key Differences Between AI-Powered and Traditional Online Photo Editors in 2024 - Automated Background Removal vs Manual Selection Tools
When it comes to removing backgrounds from images, the choice between automated AI-powered tools and traditional manual selection methods boils down to a trade-off between speed and control. AI background removal relies on advanced algorithms to analyze and automatically separate the subject from its surroundings. This approach is incredibly fast, making it ideal for quick edits and large batches of images. However, it can struggle with intricate or complex scenes where subtle distinctions are needed.
On the other hand, manual methods like using the lasso or pen tools in image editing software offer meticulous control. While this level of precision is invaluable for tasks demanding fine details and artistic finesse, it comes at the cost of time and expertise. Mastering these tools takes practice, and the editing process itself is slower compared to automated solutions.
Ultimately, the best approach depends on your specific needs. If speed and simplicity are paramount, AI-driven background removal is often the preferred option. But for projects where intricate adjustments and a high level of creative control are necessary, manual selection tools remain the go-to choice. As the technology continues to advance, the line between AI and manual techniques may blur, but for now, understanding their respective strengths and limitations remains crucial for making informed decisions in photo editing.
When it comes to removing backgrounds from images, we're seeing a shift from traditional manual selection tools towards automated solutions. These automated tools rely on sophisticated algorithms, often incorporating deep learning, to understand and interpret the content within an image. This approach can often be quicker and more accurate than manually selecting a subject using methods like the pen or lasso tools.
Interestingly, research suggests that even with intricate images, automated tools can achieve a consistent level of quality faster than a human user. This speed difference is substantial; where a skilled person might spend minutes isolating a subject, automated systems can produce similar results in mere seconds. However, this efficiency doesn't come without some caveats. Automated methods can sometimes introduce minor flaws, particularly around intricate details or edges. Hence, a degree of manual refinement often proves valuable to achieve the desired result.
While manual selection offers granular control for highly detailed edits, automated background removal has made significant progress in handling diverse image types and scenarios through learning from vast datasets. The accessibility of AI-driven solutions is also noteworthy. Anyone can potentially achieve professional-looking results, bypassing the steep learning curve associated with programs like Photoshop.
The rise of these automated tools has sparked discussion within creative fields. The focus is on the evolving relationship between efficiency and artistic control in image editing. Will reliance on automation diminish the importance of traditional image editing skills?
Some systems have begun using a blend of edge detection and semantic segmentation techniques to enhance background removal accuracy. These developments highlight ongoing innovation in image processing technology. And as these technologies mature, we're likely to see the lines between automated and manual editing become increasingly blurred. Tools that combine the best of both approaches—providing users the freedom to seamlessly integrate manual refinements within automated processes—are becoming increasingly common. This fusion of technology and human creativity could represent the future of background removal and image editing in general.
7 Key Differences Between AI-Powered and Traditional Online Photo Editors in 2024 - Real Time Style Transfer vs Preset Filters
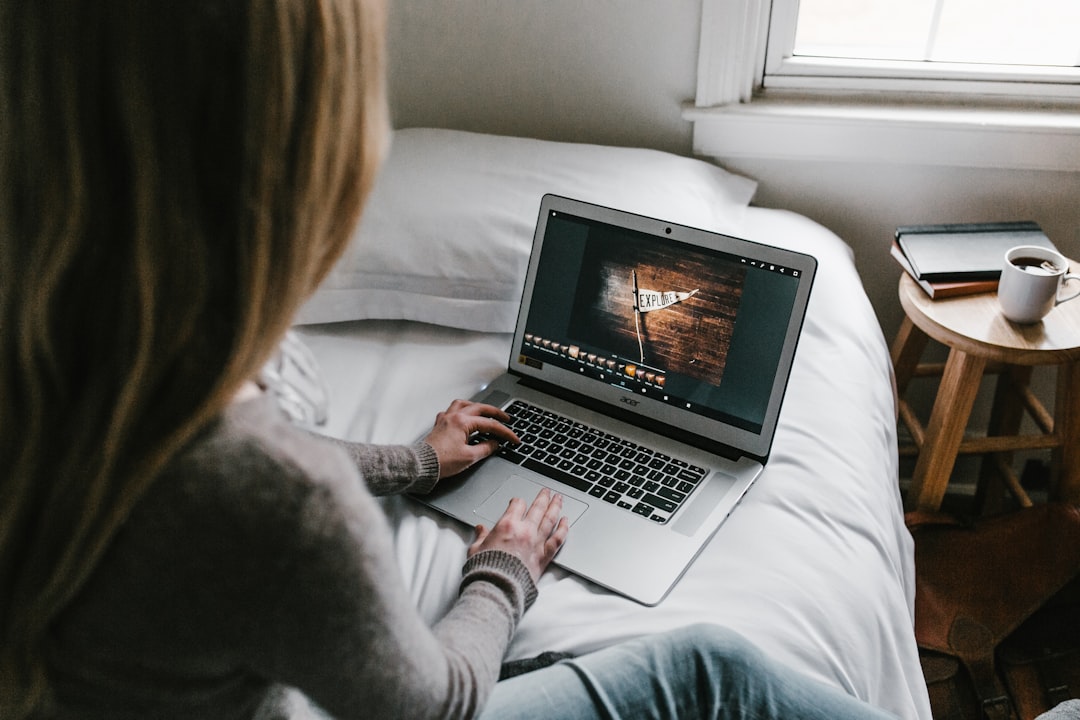
The landscape of online photo editing has shifted in 2024, with the rise of real-time style transfer offering a stark contrast to traditional preset filters. AI-powered style transfer leverages deep learning to seamlessly blend artistic styles onto images, delivering near-instant results. This capability opens up a much wider range of artistic options, but the sophisticated algorithms require significant processing power. As a result, high-quality real-time style transfer often relies on robust hardware like GPUs, potentially leading to slower performance on less capable devices.
Preset filters, on the other hand, provide a much quicker and simpler editing experience. They simulate various styles without the intricate neural network processing of style transfer, making them faster and more accessible. However, the range of styles available in preset filters is usually more limited, and users have less control over the final look compared to real-time AI-powered options.
The evolution of AI capabilities is steadily making advanced style transfer techniques more commonplace and efficient. The question for users then becomes one of striking a balance between the speed of readily available filter options and the breadth of creative possibilities offered by real-time style transfer. It highlights a key tension in modern image editing—the desire for both fast, simple solutions and sophisticated, nuanced creative control.
Real-time style transfer, powered by deep learning algorithms like convolutional neural networks (CNNs), offers a dynamic approach to altering image aesthetics. It essentially learns from a vast collection of artistic styles and applies them to an image in real-time. The complexity of the style chosen can significantly impact the processing time and resulting output, a stark contrast to preset filters. Preset filters, on the other hand, are computationally simpler, applying pre-defined adjustments with limited flexibility. They often focus on basic color and lighting modifications without the same level of pixel-level manipulation that style transfer provides.
Style transfer's ability to adapt to different images and styles arises from its reliance on neural networks, which learn to understand and mimic artistic techniques in a way that rules-based preset filter algorithms cannot. However, this comes at a cost—real-time style transfer is far more demanding on hardware resources. It needs powerful processors, particularly GPUs, to handle the intricate calculations necessary for real-time processing. This can lead to challenges when using the technology on mobile devices or systems with limited processing capabilities, unlike preset filters, which are generally designed for lighter workloads.
One of the key aspects of style transfer is its capacity for producing diverse and unique outcomes based on factors like the input image, selected style, and stylization level. This adaptability creates a more personalized editing experience compared to the standard, often uniform, results of preset filters. Interestingly, research suggests that people often find images altered through style transfer more visually appealing and creative due to the novel textures and patterns it generates. This unique ability to extend beyond typical editing aesthetics can be both a strength and a hurdle for adoption.
While preset filters are generally easy to use and accessible to users of any experience level, mastering style transfer can require a more in-depth understanding of neural networks and artistic principles. Additionally, many current style transfer models have limitations regarding image resolution, potentially impacting their effectiveness with very high-resolution images. This contrasts with preset filters, which can generally handle images of varying sizes without major performance hiccups. Finally, while style transfer allows for the combination of multiple styles in innovative and hybrid ways, achieving the desired result often necessitates meticulous parameter adjustment to avoid distortions or visual artifacts. Preset filters, in contrast, typically deliver predictable outcomes with minimal need for user intervention.
The field of AI-powered image editing is constantly evolving, and while real-time style transfer presents significant potential for creative expression, its current limitations and learning curve might restrict its wider adoption among casual users. Nonetheless, the advancements in processing power and ongoing research suggest that style transfer may become more accessible and practical for a broader audience in the future.
7 Key Differences Between AI-Powered and Traditional Online Photo Editors in 2024 - Smart Object Detection vs Manual Layer Management
When editing photos online, you have a choice between two main ways to work with different parts of an image: smart object detection and manual layer management. Smart object detection utilizes artificial intelligence to automatically identify and group elements within the picture. This automation makes editing much faster and more adaptable. In contrast, manual layer management is a more traditional approach. It requires users to manually adjust and control each layer individually. This gives the user a lot of control but takes time and a certain level of skill to master. The rise of AI in editing is leading to tools that can process photos faster, although sometimes this speed comes at the cost of finer control that experienced editors might want. Choosing between these two approaches involves deciding between the convenience of AI's automation and the artistic control of more traditional editing techniques.
When it comes to manipulating image elements, the contrast between smart object detection and manual layer management becomes apparent. Smart object detection, powered by AI algorithms, can automatically pinpoint and categorize elements within an image with remarkable precision. For instance, these algorithms often achieve accuracy rates exceeding 90% in recognizing and segmenting objects, a level of detail that frequently outstrips the reliability of manual layer management, especially in complex images. This sets a higher bar for automated systems, demanding consistently accurate results.
In contrast to manual layer management, which relies on user input and a solid grasp of the software, AI-driven object detection learns iteratively from vast datasets. This continuous learning allows it to adapt and improve its performance over time, a feature inherent to AI that manual methods simply can't replicate. It's akin to an automated system refining its skills based on experience, which can lead to progressively better outcomes.
Smart object detection leverages advanced techniques like convolutional neural networks (CNNs) and metrics such as Intersection over Union (IoU) to refine the boundaries of objects. This makes it especially good at identifying and separating intricate details and patterns that manual methods may struggle with without considerable effort. It highlights the efficiency of specialized AI approaches for tasks demanding fine detail.
Interestingly, studies have shown that automated object detection can shave up to 70% off editing time for images with multiple layers or complex backgrounds. This emphasizes the potential gains in efficiency compared to manual methods, where adjustments can be painstakingly slow. It challenges the traditional approach of relying solely on manual edits.
Regarding image format compatibility, smart object detection tools are built to handle a wide range of file types and resolutions without sacrificing quality. Manual layer management, however, can be more prone to errors and inconsistencies during format conversions, a factor that highlights the benefits of more adaptable AI approaches. This means AI systems may be less impacted by variability in image source.
AI-powered object detection systems can often provide real-time previews as the detection process happens. Users get instant feedback on their selections, making the experience interactive. Manual methods typically involve rendering the changes, which can slow down the workflow and make quick adjustments less straightforward. The capacity for real-time previews underscores the usability improvements in AI-driven systems.
The reliance on intelligent algorithms in object detection also introduces the ability to understand the context of an image. For example, AI can identify relationships between objects, something manual methods often lack due to their focus on isolating individual layers. Contextual awareness within images enhances the level of understanding and potentially the accuracy of the outcome.
An unexpected consequence of smart object detection is its ability to boost accessibility. Individuals with limited technical expertise can produce results comparable to those of skilled professionals simply by using automated systems. This significantly lowers the entry barrier to image editing compared to the steep learning curve associated with manual methods. It can democratize image editing for broader user groups.
Furthermore, collaborative workflows can significantly benefit from smart object detection. Multiple users can simultaneously work on an image, making edits based on shared, automated outputs. This promotes real-time collaboration and creative exploration, a benefit not readily available with manual layer methods due to their linear and single-user nature. Collaborative workflows are inherently more interactive and open to diverse perspectives.
Finally, while smart object detection excels at rapid execution, some believe it could foster over-reliance on automation, potentially hindering the development of traditional image editing skills. This prompts us to contemplate the importance of striking a balance between harnessing cutting-edge technology and maintaining a foundation in established image editing techniques. We should acknowledge that AI can potentially impact how humans learn and practice existing skills.
7 Key Differences Between AI-Powered and Traditional Online Photo Editors in 2024 - One Click Portrait Enhancement vs Manual Skin Retouching
The difference between using a one-click portrait enhancement feature and manually retouching skin highlights a core tension in photo editing today. AI-driven tools can swiftly smooth skin, detect faces, and generally improve portraits with just a click, saving significant time for users focused on speed. This automated approach is a boon for quick edits and handling lots of photos. However, manual skin retouching offers a level of detail and control that AI can't always match. Experienced editors can achieve highly personalized results with careful adjustments, crafting unique looks that cater to individual preferences. While AI excels at processing large numbers of images, the artistic element of skin retouching often benefits from a human touch, ensuring a more refined, bespoke outcome. The ideal choice depends on the balance you seek between speed and precise artistic control.
In the realm of online photo editing in 2024, the rise of AI has introduced a compelling dichotomy in portrait enhancement: the speed and accessibility of "one-click" solutions versus the nuanced control offered by traditional, manual skin retouching. AI-driven one-click tools are remarkably fast, capable of reducing editing time by as much as 80%. This is due to sophisticated algorithms that analyze and modify multiple image elements simultaneously. The consistency these algorithms provide across many images contrasts with the potential for variability that comes with manual techniques, which are often influenced by the editor's individual preferences and skill level.
Furthermore, AI systems are trained on enormous datasets of skin tones and textures, allowing them to make more informed decisions about adjustments compared to a retoucher working solely from experience or personal aesthetic. However, one-click solutions aren't without drawbacks. They often falter in scenarios involving unusual lighting or intricate skin issues like blemishes or scarring, areas where the adaptability and subtle control of human retouching excels. These automated tools also incorporate a broader spectrum of corrections, including automatic tweaks to exposure, contrast, and saturation, which may not be the primary focus of a manual retouch.
The simplicity and ease-of-use offered by these AI tools have undeniably democratized image editing, enabling individuals without specific training to generate high-quality results. This contrast with manual retouching, which often has a steeper learning curve, is stark. However, reliance on these automated solutions could possibly impede the development of fundamental manual retouching skills, potentially leading to a reliance on technology that might hinder the user when faced with situations where AI isn't sufficient.
Though AI systems offer instant visual feedback, they might lack the profound understanding that an experienced editor possesses when it comes to the subtle gradations of skin tone, potentially leading to outcomes that seem artificial in some contexts. The tailored artistic approaches inherent to manual retouching allow for flexibility and can be more readily adjusted to specific requirements of the portrait subject. This contrasts with the typically faster but less customizable approaches of one-click enhancement tools. Finally, user surveys suggest that while many individuals welcome the speed and convenience of one-click portrait enhancement, a significant segment still favors manual retouching for crucial projects, recognizing the irreplaceable element of human artistic control. The ongoing debate between automation and human expertise continues to shape the landscape of online image editing, and both approaches likely will play important roles depending on the user's goal.
7 Key Differences Between AI-Powered and Traditional Online Photo Editors in 2024 - Generative Fill and Expansion vs Traditional Clone Stamp
The rise of AI in photo editing has brought about powerful new tools like Generative Fill and Expansion, which offer a stark contrast to the traditional Clone Stamp. Generative Fill utilizes AI to produce new image content based on user prompts, allowing for a seamless integration into existing photos. This differs significantly from the Clone Stamp, whose function is simply to copy and paste existing pixel data to cover unwanted areas. Taking this further, Generative Expansion enables the expansion of an image's canvas while intelligently filling the new area with generated content—a task impossible with the Clone Stamp's inherent limitations. This AI-driven approach opens up new possibilities for creativity and complex image manipulation, in contrast to the more basic, pixel-level adjustments of the Clone Stamp. It's clear that AI-powered editing tools like Generative Fill and Expansion are changing the landscape of photo editing, allowing users to go beyond simple edits and explore more intricate creative possibilities. While traditional methods like the Clone Stamp still have their uses, they are limited in comparison to what AI-driven options can offer, especially as the technology continues to evolve throughout 2024.
Generative Fill employs sophisticated algorithms to understand and extrapolate the characteristics of an object within an image and then intelligently fills the surrounding area. In contrast, the traditional Clone Stamp tool simply copies pixels from elsewhere in the image, lacking any deeper understanding of context. This difference is quite noticeable in the level of detail and realism achieved.
Generative Fill delves into complex computations to analyze the image and predict what should be generated, while the Clone Stamp relies heavily on user-defined selections. This can lead to noticeable seams or imperfections that disrupt the overall cohesiveness of the image, especially in areas where there's a difference in textures or lighting.
Research suggests that generative models have the capacity to learn from a vast array of images, allowing them to synthesize new image sections that seamlessly integrate with the existing photograph. They can mimic the light, texture, and color dynamics of the original image remarkably well. The Clone Stamp, being a more simplistic tool, doesn't have this capability and can produce rather rigid results.
Generative Fill can adapt to different artistic styles and often incorporates subtle variations to ensure a realistic outcome. This differs significantly from the Clone Stamp, which generally results in a uniform application and lacks the nuanced detail that's often present in the original portions of the image.
The efficiency of Generative Fill is impressive, considerably reducing editing time. Complex tasks that might take hours with a Clone Stamp can be completed in seconds using Generative Fill. This is due to its ability to automatically fill areas based on the surrounding image rather than requiring the user to manually copy and adjust pixel by pixel.
For creative workflows, users often find that Generative Fill greatly enhances their ability to visualize completed edits quickly. In comparison, relying solely on the Clone Stamp can restrict creativity due to its labor-intensive and time-consuming nature. This difference in speed and ease of use can make a major difference in creative output.
Generative Fill also incorporates a "content-aware" approach, enabling it to recognize patterns and generate fills that seamlessly blend into the surrounding environment. The Clone Stamp, however, often struggles to achieve natural-looking blends because it lacks this inherent contextual awareness.
Studies have shown that the visual consistency achieved through generative processes is generally seen as superior to results from Clone Stamping. Clone Stamping often leads to inconsistencies in lighting and texture that are apparent to trained eyes or those who look carefully.
A potential challenge with Generative Fill is that it can sometimes overinterpret the complexities of an image, leading to artifacts or unrealistic results that need further manual refinement. With a Clone Stamp, users have direct control, but that control comes at a cost of reduced editing efficiency.
As Generative Fill technologies continue to mature, they're developing the ability to learn and improve based on user interactions. This creates a continuous feedback loop that refines the image generation process over time. In contrast, traditional Clone Stamping lacks this capacity for adaptive learning. It can be argued that this ongoing improvement in image understanding is a huge advantage in the field.
7 Key Differences Between AI-Powered and Traditional Online Photo Editors in 2024 - AI Upscaling Technology vs Standard Resolution Enhancement
AI upscaling technology has fundamentally changed how we enhance image resolution compared to older, standard methods. Traditional resolution enhancement typically involves simply copying or blending existing pixels to create new ones. While seemingly straightforward, this often leads to images that appear blurry or pixelated, especially when significantly increasing the resolution. In contrast, AI upscaling uses sophisticated machine learning to analyze the image and "guess" what new details should be added. This allows for a much more natural and realistic increase in resolution, maintaining clarity and bringing out more intricate textures and colors. AI-powered upscalers can handle resolutions like 4K and beyond, producing results that are significantly superior to what was previously possible with standard methods.
Despite the clear advantages of AI upscaling, it's important to remember that the older methods still exist and have their own niche applications. Users need to understand the strengths and limitations of each approach to decide which one is best suited for their specific projects and goals. The photo editing landscape is constantly evolving with AI becoming increasingly prominent. As of 2024, understanding the differences between AI and traditional upscaling techniques is crucial for making informed decisions and getting the desired results from online photo editing tools.
AI upscaling leverages complex algorithms to analyze an image's structure and textures, predicting and filling in missing details to produce a higher-resolution output. In contrast, traditional upscaling relies on simpler interpolation methods, which simply replicate existing pixels, often leading to noticeable blurriness or artificial artifacts.
AI upscaling models are trained on vast datasets of high-resolution images, using deep learning to understand how textures and details should look. This enables them to produce more convincing and sharper upscaled versions of low-resolution photos compared to traditional methods that lack this training, leading to less accurate representation of missing details.
AI upscaling stands out for its speed, often upscaling images in real-time or within a few seconds. Traditional methods are frequently much slower, especially when dealing with complex images, demanding manual adjustments that significantly increase editing times.
Surprisingly, AI upscaling techniques have shown a capability to improve image quality while maintaining the integrity of the original source image. Traditional methods can inadvertently distort certain features, leading to a less authentic representation of the original photo.
While traditional upscaling tools uniformly apply enhancements across an entire image, AI can adaptively enhance specific image areas based on their context. This targeted approach helps create a more coherent and natural-looking visual output compared to the often less visually consistent results from standard upscaling.
Research suggests that people generally find images enhanced using AI upscaling techniques more visually appealing than those processed through traditional methods. This preference reflects a perception that AI techniques provide a better understanding of intricate details and nuanced clarity in images.
AI upscaling utilizes advanced techniques like Generative Adversarial Networks (GANs) to generate entirely new pixel data. This ability to create and fill in textures and gaps not present in the original image is unique to AI and represents a higher level of creativity and depth that traditional methods cannot match.
Interestingly, many current AI upscaling methods incorporate a feedback loop within their learning processes. As they process more data and get feedback, they continue to refine their ability to improve image quality over time, unlike traditional upscaling methods, which remain static.
Another consideration is compatibility. AI upscaling techniques are often designed to handle a wider range of input sources and formats, allowing them to upscale a broader variety of images without significantly affecting the quality. Traditional methods may struggle with some file types and details, which can limit their versatility.
Finally, while AI upscaling is undoubtedly transformative, it does have a tendency to sometimes overinterpret patterns in an image, leading to an appearance of excessive artificial enhancement that might not be present in standard upscaling methods. This tension between pushing technological boundaries and preserving the authentic characteristics of the source image represents a critical issue in this evolving field of digital image manipulation.
7 Key Differences Between AI-Powered and Traditional Online Photo Editors in 2024 - Cloud Based Processing vs Local Computing Power
The choice between cloud-based processing and using the computing power of your own device is a fundamental shift in how online photo editing, particularly with AI, is performed. Cloud computing, with its remote servers and internet-based services, allows for a more flexible and accessible approach to editing. Users gain the benefit of powerful tools without needing top-tier hardware on their own systems, leading to lower upfront costs and easier collaboration. Plus, cloud services often receive automatic updates, freeing users from the burden of software maintenance. On the other hand, local computing maintains tighter control over your data since it doesn't need to be transmitted online, which is essential when dealing with sensitive information. Depending on the situation, local processing might be preferable if internet connectivity is unreliable. Essentially, choosing between these options boils down to evaluating the convenience, accessibility, and collaborative advantages of cloud-based editing compared to the security and control that local processing delivers. As AI photo editing tools evolve, understanding these trade-offs will only become more important.
When comparing how AI-powered photo editors handle processing, we see a split between using remote cloud resources and relying on the processing power built into a user's computer. Cloud processing, using distant servers connected via the internet, can adjust the amount of computing power it uses on the fly. This is especially beneficial when a user is doing something computationally intensive, such as processing very high-resolution images, as the cloud service can essentially call in more resources to keep things moving smoothly. But relying on cloud services comes with the drawback of introducing a delay in the processing due to the time it takes to send data back and forth. In comparison, using the processing power in a person's computer offers practically instant results, making it the speedier option when it comes to dealing with big files or making quick edits.
Privacy becomes a concern when we rely on cloud services because the images are sent to remote servers, which inherently puts them at risk of unauthorized access. In contrast, keeping data local on a person's own computer minimizes that risk, keeping control within a single device. Furthermore, cloud-based photo editing needs a stable internet connection, and if the connection drops, users might lose access to their work or have editing tools become unavailable. In comparison, processing on a local machine doesn't have this dependency, ensuring consistent functionality even if the user is offline.
One significant benefit of cloud-based AI is that the models powering editing features can be updated or retrained continually using new datasets, improving performance and feature accuracy. In contrast, locally-installed software often needs to be manually updated by the user, which could potentially lag behind the most advanced editing technologies. However, while cloud services might seem cheaper due to not having to buy hardware upfront, the subscription fees for continuous access to them can add up over time. This could lead to costs exceeding the initial purchase price of comparable local software or upgrades for more avid users.
Cloud services can also offer specialized, advanced features like unique AI tools as they become available. Local computing systems might have difficulty implementing these due to limitations on their hardware. The downside is that cloud performance can vary based on external factors like server load and internet speed in a specific geographic location. In contrast, the processing power of a local machine stays relatively consistent, making the performance more predictable during edits.
Users who have local computers can also customize their machine to enhance the capabilities they need for editing, like buying specialized components for dealing with high-resolution images. This is in contrast to cloud providers, which typically use standard, general-purpose hardware that might not be the best fit for everyone's editing needs. Additionally, cloud services might have restrictions on the number of images processed or the size of the files a user can handle, hindering users working on larger projects. These limits don't typically affect users who choose to process their photos locally, which allows them to have more flexibility in how they manage their photo edits.
More Posts from colorizethis.io: