7 Pivotal AI Events from Fall/Winter 2021 That Shaped Today's Image Generation Technologies
7 Pivotal AI Events from Fall/Winter 2021 That Shaped Today's Image Generation Technologies - DALL-E First Public Demo at NeurIPS Conference December 2021
During the December 2021 NeurIPS conference, held virtually, OpenAI's DALL-E had its inaugural public showing. This marked a key moment for the field of AI-driven image generation. The demonstration vividly illustrated DALL-E's ability to create images based on textual prompts. Interestingly, this event also reflected the collaborative nature of AI research, with researchers pooling resources for model training efforts. Discussions within the NeurIPS environment focused on the progress and future of AI-powered image generation, paving the way for refinements and new versions like DALL-E 2, released a year later, with improvements in image realism. The event was undeniably impactful, underlining the rapid progress in artificial intelligence and planting the seed for the next generation of sophisticated image generation technologies.
The NeurIPS 2021 conference provided the first public glimpse of DALL-E, OpenAI's intriguing project that aimed to bridge the gap between text and images. Held virtually in December 2021, the event served as a platform to showcase the AI's ability to translate textual descriptions into corresponding visual representations. It was fascinating to witness the early stages of this work that explored the potential for AI to grasp and manifest visual creativity.
Interestingly, DALL-E, introduced earlier that year, leveraged a modified version of OpenAI's GPT-3 language model, underscoring the growing relevance of transformer architectures in generative modeling beyond just text. This approach highlighted the core idea of DALL-E, which was to leverage the power of a language model, that had learned the intricacies of language from a vast dataset, to translate such learned knowledge into visual form.
The demo at NeurIPS emphasized DALL-E's aptitude for zero-shot learning, a feature that demonstrated its ability to handle new, unseen prompts. This was noteworthy considering it suggested that the model could go beyond just regurgitating training data and apply its learned understanding of the world to produce appropriate visuals. However, the limitations of DALL-E were also visible. Its struggles with generating accurate human anatomy or lifelike faces hinted at shortcomings in the training dataset or the model's architectural limitations.
Subsequent iterations of DALL-E (e.g., DALL-E 2) have addressed some of the initial limitations in quality and fidelity, showing how quickly the technology evolved. While the demo at NeurIPS 2021 was certainly intriguing, the ensuing discussion about copyright and the potential for AI-generated content to contribute to misinformation has been a vital point of debate and research that continues today. The NeurIPS conference, through its presentations and workshops, effectively highlighted the nascent field of AI-powered image generation and some of its early challenges and promises. It laid the groundwork for a rapidly evolving landscape, encouraging researchers to further explore and refine this promising area of artificial intelligence.
7 Pivotal AI Events from Fall/Winter 2021 That Shaped Today's Image Generation Technologies - Stable Diffusion Precursor CompVis Released Initial Research Paper
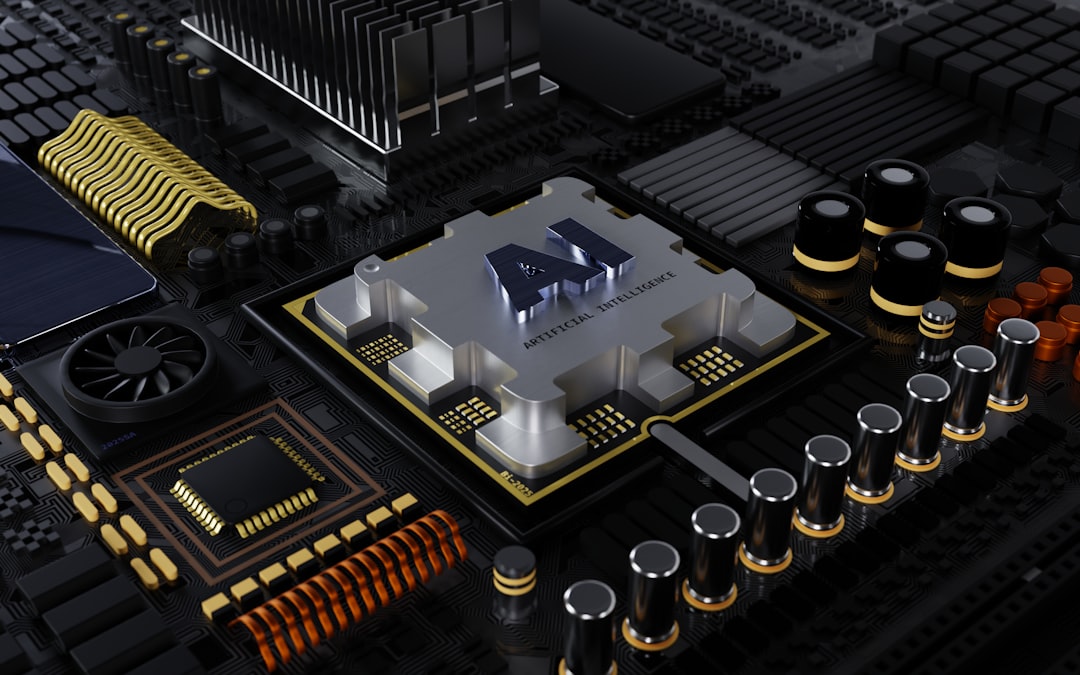
In the fall and winter of 2021, the research group CompVis released a foundational research paper that would eventually lead to Stable Diffusion. This paper, "High-Resolution Image Synthesis with Latent Diffusion Models," introduced a new approach to AI-powered image generation. The core concept was a latent text-to-image diffusion model capable of producing high-quality images from textual prompts. This initial work was done in collaboration with Stability AI and LAION.
One of the key innovations presented in this research was the use of a frozen CLIP text encoder for conditioning. This approach, in the midst of a flurry of new generative AI models, demonstrated a novel strategy for how text prompts can be used to influence image creation. While the core focus of this work was text-to-image generation, the researchers also incorporated features for inpainting and image variation, expanding the capabilities of the model.
The release of this research and the ensuing open-sourcing of related tools played a vital role in how AI image generation technology developed. This event provided the basis for what is now widely used technology and also exemplifies how collaborative efforts can lead to advancements in AI. The open nature of this early work fostered wider adoption and contributed to a surge of interest and subsequent work in AI-driven image generation.
CompVis's initial research paper, which later led to Stable Diffusion, was a fascinating development in the world of image generation. It introduced the concept of latent diffusion models, a departure from the then-dominant GANs. This approach involved a process of slowly "denoising" random noise into realistic images. This was a different way of thinking about image synthesis, compared to earlier methods that focused on directly generating images from randomness.
The paper highlighted how diffusion models could achieve surprisingly high levels of detail and visual fidelity, setting a new benchmark. It demonstrated how they could harness large datasets of image-text pairs to generate images that matched textual prompts remarkably well. This opened up new avenues for exploration within the AI community, with everyone rushing to experiment with these ideas.
One interesting facet of the paper was its exploration of model interpretability. The researchers showed that you could manipulate the diffusion process by adjusting specific parameters, giving more control over the output. It provided a degree of transparency into how these powerful systems worked.
However, it also highlighted the inherent limitations. It showed that model outputs were sensitive to the quality of the training data, which can lead to unwanted biases. This presented an ethical conundrum – how do we create powerful tools without amplifying biases present in the real world? The paper also highlighted the immense computational resources needed to train these models, which raised questions about the feasibility of their deployment on a wider scale.
Despite these limitations, the paper provided compelling evidence of the advantages of diffusion models through benchmarks that compared their outputs to other cutting-edge generative AI systems. It was clear that they were able to generate higher-resolution and more detailed images, which led to a lot of excitement.
Interestingly, this wasn't just a purely technical advancement. It also triggered wider discussions about the societal impacts of making image synthesis technology more accessible. It was clear that a powerful technology like this could be applied in many ways, and thinking through those implications was going to be crucial.
The initial research from CompVis served as a foundation for subsequent work in the field. It pushed forward the capabilities of AI-driven image generation and inspired a whole new wave of user-interface developments, allowing even non-experts to create high-quality visuals. The research team's work paved the path for future innovations in this space and sparked a new era of creative possibilities and discussions about their consequences.
7 Pivotal AI Events from Fall/Winter 2021 That Shaped Today's Image Generation Technologies - Google Brain Introduces Improved VQGAN Architecture November 2021
In late 2021, Google Brain researchers introduced a refined version of the Vector Quantized Generative Adversarial Network (VQGAN). This updated architecture aimed to tackle a key challenge in image generation: enhancing the quality of reconstructed images. It achieved this by incorporating an adversarial loss function, which improved upon the capabilities of its predecessor, the Vector Quantized Variational AutoEncoder (VQVAE).
The new design involved a two-stage approach that fundamentally revisits how images are encoded and decoded into discrete representations. This change sought to simultaneously improve image generation and comprehension tasks.
Tests conducted on ImageNet, a large image dataset, revealed significant performance boosts compared to the original VQGAN. These included notably improved Inception Scores and Fréchet Inception Distances, suggesting a marked improvement in generated image quality.
The architecture's improvements stem from refinements in how the "codebook" – essentially a lookup table of image features – is learned. These tweaks, along with architectural adjustments, resulted in a more efficient and faithful image reconstruction process. These enhancements make the updated VQGAN a meaningful step forward in the evolving field of AI-powered image generation. Notably, this iteration, dubbed ViTVQGAN, focuses on unsupervised representation learning, which has relevance in a broader range of visual AI applications. While improvements are apparent, it's still important to consider the role that such methods play in the larger conversation around AI's impact on creativity and image content in general.
In late 2021, Google Brain researchers unveiled a refined version of the Vector Quantized Generative Adversarial Network (VQGAN) architecture. This new iteration aimed to address some of the shortcomings of earlier image generation methods, particularly the Vector Quantized Variational AutoEncoder (VQVAE). They achieved this through a clever combination of techniques.
A major part of the improvement was the integration of adversarial loss functions into the model. This change shifted the focus from merely reconstructing images to optimizing them based on a more perceptual understanding of what makes a good image. Interestingly, the model was trained on ImageNet, resulting in good metrics (Inception Score of 17.51 and Fréchet Inception Distance of 4.17), when working with images at 256x256 resolution, showcasing improvements over earlier methods. The overall goal was a two-stage process that rethought how image information was encoded into a smaller set of features, aiming for better generation and overall image comprehension.
This two-stage process initially involved encoding images into a compressed format using a vector quantized approach. The improvements to VQGAN came from better codebook learning methods and some careful adjustments to the architecture itself, which resulted in a more efficient and faithful reconstruction of the input images.
Beyond the core VQGAN improvements, there was a related proposal for Vector Quantized Image Modeling (VIM). This idea involved using a transformer network to predict pixels in a sequential manner, effectively treating image generation as a language modeling task, which was interesting. The whole endeavor highlighted the crucial role of high-quality image reconstruction in these models, emphasizing its importance in both basic and conditional image generation scenarios.
This new architecture, also referred to as ViTVQGAN, was positioned as a way to improve unsupervised representation learning. It essentially tried to learn features from unlabeled data with a better image reconstruction capacity than the baseline VQGAN model.
It's worth noting that while these improvements were impressive, it was not without challenges. The complex nature of the model meant that it still struggled to create perfectly formed images for complex prompts. This isn't surprising, as translating a complex idea into a visually compelling image remains a difficult task. Further, training these models demands significant computational resources. The computational needs of this class of models has been a recurring pattern in recent years and it certainly raises questions about accessibility for researchers with fewer resources.
This work fit within a larger trend in AI towards multi-modal learning. These kinds of models were able to better integrate information from different sources, like text and images. This shift opens up opportunities for future AI systems that are better at understanding the complex relationships within the world. One other potentially exciting aspect of the model was its suitability for real-time applications, suggesting a possible future in games and similar interactive media environments.
Ultimately, this work isn't just interesting for its technical components. It has implications for a range of fields, including art and design. The ability to generate images with such fidelity compels us to consider the impact on originality and copyright. There are also ethical considerations to ponder related to how AI-generated images can affect our understanding of the world and media landscapes. It's important to consider those aspects as the technology progresses.
7 Pivotal AI Events from Fall/Winter 2021 That Shaped Today's Image Generation Technologies - Midjourney Founder David Holz Begins Alpha Testing
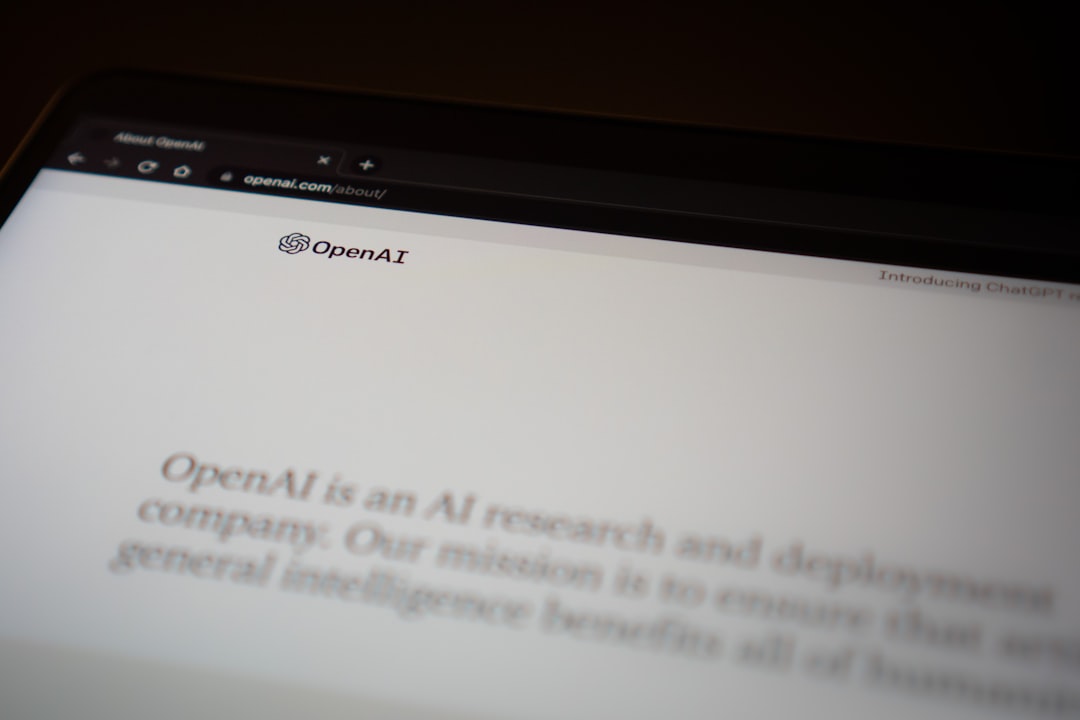
Midjourney, an independent research lab spearheaded by David Holz, has initiated alpha testing for its fourth iteration of the text-to-image synthesis model. Holz, a pioneer in user interface development, has focused Midjourney on expanding the scope of human creativity through AI-powered image generation. Their small team has been refining the model, which is currently accessible to subscribers via their Discord platform.
The alpha testing phase offers users a preview of the new features, which include the prospect of generating multiple images concurrently and the potential integration of an external image editor in future updates. Midjourney’s roadmap includes version 7, planned for release in the coming months. Holz's overarching goal is to democratize AI image generation, making it more practical and beneficial for professionals in a range of fields. It's a notable development in the continuing journey of AI-driven image creation. While promising, it also raises questions about how accessibility and creativity are intertwined with this evolving technology.
David Holz, the founder of Midjourney, comes from a background in human-computer interaction, having previously co-founded Leap Motion, a company focused on hand gesture-based interfaces. This experience likely influenced Midjourney's emphasis on user-centric design and exploration of new creative mediums.
Midjourney's initial foray into the AI image generation landscape was marked by an alpha testing phase using a selective approach. By inviting a limited group of users, Midjourney was able to collect targeted feedback on its then-new model. This strategy facilitated iterative improvement of the model and allowed it to adapt to user needs before broader adoption.
Interestingly, Midjourney opted for Discord as its platform for user interaction, providing a unique social component alongside the text-to-image generation process. This choice fostered a community centered around shared experimentation, collaboration, and the exchange of creative insights within the platform's environment. This community aspect is a significant departure from many other image generation platforms that were more focused on isolated or individual usage.
Holz placed a strong emphasis on community engagement, encouraging users to share their experiences, critiques, and suggestions for improvement. This was a crucial component of Midjourney's development, facilitating rapid adaptation and improvement based on direct user feedback. One might argue that this active community involvement, while perhaps time-consuming, contributed significantly to Midjourney's rapid growth and unique culture.
Midjourney's underlying AI architecture used a novel approach, drawing upon diffusion models for image generation. This offered a distinct advantage over many early models, enabling it to generate more coherent and high-resolution images from user-defined prompts. Whether or not this method truly represents a fundamental improvement in the field or was simply the dominant model of the time, it put Midjourney at the forefront of the technology.
While many early AI image generation tools focused on replicating photorealistic images, Holz envisioned a more artistic and expressive application for Midjourney. This perspective encouraged users to experiment with more interpretive and nuanced approaches to prompt design, resulting in a broader range of creative outputs. It remains to be seen whether this approach has resulted in more valuable art than simply replicating realism, though it certainly made the system more popular for a broader user base.
The alpha testing also raised practical ethical issues, including copyright considerations and ownership of the generated art. Holz's proactive engagement with these concerns is notable, as they have only become more prominent and complicated in the years since. Whether or not Holz and Midjourney were uniquely prescient or whether this was a general topic of discussion in the field remains debatable.
One hurdle to broader adoption of Midjourney has been the considerable computational resources required for generating images. While feasible for many researchers, especially those affiliated with research institutions and corporations, this accessibility constraint restricted wider use in certain settings, such as small design studios or independent artists who might not have ready access to powerful hardware. The rise of specialized co-processors designed specifically to improve this situation over the next decade has made this an increasingly less important issue.
Midjourney provided users with granular control over the output by introducing options for influencing image style and visual elements, allowing for experimentation with aesthetics and artistic approaches. The range of possibilities generated by these parameters certainly expanded the artistic range of the AI and led to a wider user base who found it more creative and interesting to use.
Holz's vision for Midjourney extends far beyond mere image generation. He aims to build a bridge between AI technology and human imagination, reshaping the role of artists in a world increasingly mediated by digital technologies. Whether or not AI systems ultimately enhance artistic expression is still a topic of much debate and has implications for the future of art education.
7 Pivotal AI Events from Fall/Winter 2021 That Shaped Today's Image Generation Technologies - Nvidia Releases StyleGAN3 With Better Face Generation October 2021
Nvidia's release of StyleGAN3 in October 2021 represented a notable step forward in AI-powered image generation, specifically in the realm of facial imagery. A key feature of StyleGAN3 was the introduction of an "alias-free" generator designed to address the pesky visual artifacts that plagued previous versions like StyleGAN2. This improved generator, coupled with refined training methods and tools for detailed image manipulation, offered users a more refined level of control over the generated images.
While this increased control and quality came at the cost of increased computational demand, StyleGAN3 still delivered high-quality output as evidenced by its Fréchet Inception Distance scores. This signifies its ongoing relevance as a key tool for image generation. The model's development, including collaboration with researchers at Aalto University, highlights the ongoing and collaborative nature of the pursuit of enhancing AI's capabilities in producing realistic and diverse visual content. StyleGAN3 is a reminder of the complexity and potential of AI-generated imagery, as well as the continued advancements in this area.
Nvidia's StyleGAN3, released in October 2021, represented a significant leap forward in image generation, especially for faces. It built upon the successes of StyleGAN2, but with a new approach that aimed to eliminate the artifacts that sometimes plagued earlier models.
StyleGAN3's key innovation was its "alias-free" generator. This new architecture was designed to be fully translation-equivariant, meaning the spatial relationship of features in a generated image remained consistent even after manipulations like moving the image. This is a crucial feature for creating images where aspects like faces or objects need to be seamlessly incorporated.
Nvidia also integrated improved training configurations into StyleGAN3. This wasn't simply about increasing training time but about better learning procedures that included techniques like multi-scale training. This allowed the model to generate finer details while still preserving the overall structure and coherence of the output images.
Further, the team introduced new tools that made understanding and controlling StyleGAN3 outputs much easier. For example, they developed tools for spectral analysis that allowed researchers to better understand how the generator produced images. It also included methods to interactively visualize and manipulate image generation features, including video generation capabilities.
Compared to StyleGAN2, StyleGAN3 is computationally more demanding. However, the results were quite impressive. The model maintained a high FID (Fréchet Inception Distance), a metric that shows how close generated images are to real ones. High FID values indicate very realistic generated images.
Researchers at Nvidia also collaborated with Aalto University in Finland on StyleGAN3's development, showcasing the collaborative nature of cutting-edge AI work and demonstrating Nvidia's commitment to pushing the boundaries of AI image synthesis. While the primary focus of StyleGAN3 was static image generation, its ability to maintain spatial relationships and create videos suggests a strong potential for application beyond static images, including animation and video game development. It was a clear indication that the technology had progressed beyond the relatively simple task of just creating still images and had matured to deal with more complicated visual generation challenges.
While technically a very successful model, StyleGAN3, like most image generation models, also raised ethical concerns. With its capability to generate very realistic images, it became clear how easily it could be used in generating fake media. This, in turn, heightened concerns about the proliferation of misinformation and highlighted the need for critical discussions around responsible use. However, this was not a problem unique to StyleGAN3. Many models of that era faced similar issues and continue to challenge us today.
Overall, StyleGAN3 stands as a notable example of how far the field of GANs progressed in the first couple of decades of the 21st century. Its architectural improvements, advanced training methods, and tools for understanding and controlling outputs demonstrate a clear advancement. It was a model that generated significant discussion around the technical and ethical considerations in the fast-growing field of generative AI. While we've moved past StyleGAN3 in the subsequent years, it played a major role in setting the stage for where AI image generation currently stands and continues to influence related work.
7 Pivotal AI Events from Fall/Winter 2021 That Shaped Today's Image Generation Technologies - Microsoft and OpenAI Launch DALL-E Research Preview
Toward the end of 2021, Microsoft and OpenAI unveiled a research preview of DALL-E, marking a significant step forward in image generation. This was part of a larger collaborative effort to broaden the reach of AI technologies by making powerful tools more widely accessible through the Azure OpenAI Service. DALL-E 2, and later DALL-E 3, allowed users to create more intricate and realistic images using just text descriptions, demonstrating the increasing potential of AI in creative fields. Importantly, Microsoft also incorporated safeguards like image watermarks, acknowledging the ethical concerns associated with AI-powered tools. The evolution of AI in image generation continues, prompting contemplation on its impact on both creative expression and responsible usage. The implications of AI are undoubtedly profound, impacting our approach to creativity and accountability in unexpected ways.
Microsoft and OpenAI's collaborative effort in launching the DALL-E research preview was a notable step forward in image generation technology. Building on their existing partnership, they made advanced AI models, including DALL-E 2 and later DALL-E 3, available through the Azure OpenAI Service. This move allowed researchers and developers wider access to experiment with the capabilities of these models and further develop the field.
The Azure OpenAI Service, now generally accessible, gives businesses and developers a platform to utilize powerful AI models like GPT-3.5, Codex, and, of course, DALL-E. DALL-E, initially introduced in early 2021, proved its ability to craft photorealistic images from natural language descriptions. With the newer DALL-E 3, the system's detail and accuracy in image generation significantly improved.
To enhance transparency and ensure proper attribution, Microsoft introduced "Watermarks" within Azure OpenAI Service. This feature subtly marks DALL-E-generated images to promote awareness of AI-generated content, which has since become increasingly important.
The collaboration's core aim is to make the benefits of these advanced AI models readily available to a wider audience. DALL-E's user interface is relatively intuitive, letting users describe the images they envision through text prompts, fostering creativity in a diverse range of fields.
At OpenAI's inaugural developer day, Satya Nadella emphasized the significance of empowering developers to build and distribute sophisticated AI models. Azure OpenAI Service facilitates this effort by providing a comprehensive platform for deploying and scaling DALL-E models.
The initial DALL-E release and its later versions were undeniably impactful. The question remains: how do these advancements affect creative fields and artistic endeavors? How do these models affect the broader notion of authorship and ownership of creations? These are questions that researchers continue to explore today, and the release of DALL-E via the Azure OpenAI service is a testament to the ongoing exploration into the practical applications of powerful AI models in areas like image generation.
7 Pivotal AI Events from Fall/Winter 2021 That Shaped Today's Image Generation Technologies - Facebook AI Releases Make-A-Scene Text to Image Model December 2021
In late 2021, Meta's AI division unveiled Make-A-Scene, a new text-to-image model. This model demonstrated impressive image generation capabilities, achieving top-tier results in image quality assessments, both by automated metrics and human perception. It could produce detailed images at a resolution of 512x512 pixels, a step forward in visual clarity compared to prior work.
Beyond simple text-based image generation, Make-A-Scene offered novel interactive elements. Users could adjust scenes with specific anchors or even integrate freehand sketches along with written descriptions. This innovative feature fostered greater creative freedom, potentially making the model useful for both experienced artists and people who aren't artists. Meta's research team presented the project as a way to better equip AI to understand and interpret visual elements, and potentially even animate children's drawings, showing a desire to connect the model with more human creative processes.
Despite the evident potential, this development also brought forth familiar concerns. How will Make-A-Scene and similar tools impact the realm of artistic expression in the long run? What safeguards should be in place to prevent the misuse of these tools for creating misleading or harmful content? Make-A-Scene and the subsequent events of the last few years show that the development of AI in image generation is raising questions that the field is still trying to answer.
Meta's (formerly Facebook's) AI division unveiled the Make-A-Scene text-to-image model in December of 2021. This model demonstrated a notable step forward in the ability of AI to not simply translate text into images but to also allow for greater control over the structure of the resulting image. Unlike many other systems at the time, which primarily focused on producing images from text descriptions, Make-A-Scene allowed users to guide the process, placing elements in desired locations within a scene. This provided users with a more participatory role in shaping the generated output.
This focus on user interaction was a compelling aspect of Make-A-Scene. The ability to define scene layout and composition within a generated image presented a powerful new direction in image generation, addressing limitations found in AI models that simply generated outputs based solely on textual prompts.
Of course, Make-A-Scene's ability to function effectively relied on the availability of a substantial amount of image-text data for training. The researchers demonstrated the strong relationship between training data quality and the resulting quality of the AI-generated image. This emphasizes the need for large, diverse, and carefully curated datasets to unlock the full potential of AI in creative fields.
Interestingly, the Make-A-Scene team emphasized feedback from a community of users throughout the model's development. It's a trend that we've seen in other successful AI projects, where gathering user input is used to refine and improve the functionality of the technology over time. This shift toward user-centered design highlights the ongoing development of AI towards greater practicality and adaptability to a wider audience.
One of the challenges of Make-A-Scene was that it required substantial computing resources for operation. This remains a hurdle for many researchers, especially those without affiliations with well-funded research labs or large corporations. The continued need for substantial processing resources in cutting-edge AI development underscores the ongoing concern of how to distribute and democratize access to these tools more fairly, fostering broader inclusion in the design of new technologies.
Naturally, Make-A-Scene's release triggered discussions about ownership and attribution of AI-generated art. It has become increasingly evident that AI-generated content raises new questions about intellectual property rights, and the need for stronger guidelines around their use has become more pressing as the technology evolves.
Make-A-Scene was designed to be flexible and could generate images across different styles and situations. This showcases the remarkable capacity for AI to adapt when it's provided with ample and diverse training data. The model's adaptability has implications across a wide range of fields, ranging from advertising to entertainment, and can lead to a surge in new ways of designing visual experiences.
The architecture and design choices that were incorporated into Make-A-Scene served as a foundation for later image generation projects. For example, the model's emphasis on semantic scene understanding formed a cornerstone of later research in enhancing the contextual awareness of AI in generating scenes.
A crucial aspect of Make-A-Scene's development was the incorporation of methods that allowed users to iterate on the model's output. This feature emphasized the collaborative nature of the interaction between human and machine in crafting creative outputs.
When we compare Make-A-Scene to other models of the same era, such as DALL-E, it becomes evident that it allowed for significantly more complex scene generation. The ability to guide the model in creating scene layouts rather than just relying solely on textual prompts indicated a new trend in the research field of focusing on the ability for AI models to generate images not just to be photorealistic but to also be compositionally artistic and to capture narrative elements.
These are a few of the important details about the Make-A-Scene model. It remains one of the important milestones in the progression of AI-powered image generation technology. It's an intriguing example of how we can continue to experiment with human-AI collaborations in the creation of digital art and experiences.
More Posts from colorizethis.io: