AI Image Generation in 2024 A Deep Dive into Prompt Engineering Techniques
AI Image Generation in 2024 A Deep Dive into Prompt Engineering Techniques - Mastering Text-to-Image Prompts for Realistic Photography
Achieving photorealistic results with text-to-image AI hinges on mastering the art of prompt engineering. The current generation of AI image generators, like Midjourney and Stable Diffusion, have advanced significantly, yet the ability to write compelling prompts remains crucial for achieving desired outcomes. Crafting precise instructions for the AI, particularly regarding lighting conditions, can elevate the realism of the generated images. For example, thoughtfully detailing the lighting in a scene can dramatically change the overall feeling and authenticity of the output. Furthermore, the diversity of themes and settings that can be explored through AI image generation is constantly expanding. From serene beach scenes to vibrant urban landscapes, skilled prompt engineering allows for incredibly nuanced and captivating creations. The continued evolution of the field, driven by an active and engaged community, suggests that the possibilities for realistic AI-generated photography are only going to increase. While progress is clear, there's still much to be discovered in the ability of AI to truly grasp subjective elements and translate those into visuals that perfectly match a user's intention.
AI image generation has advanced to a point where it can mimic the stylistic hallmarks of established photographers. These models dissect components like exposure, framing, and even lens properties, enabling users to produce visuals evocative of specific photographers' work, without needing the technical expertise typically associated with photography. This is a fascinating development in the field.
Emphasizing contrast and dramatic lighting in our prompts can generate images with heightened visual impact, by making textures and colors stand out. It's interesting that this technique, so important in conventional photography, remains relevant in the AI realm as well.
Some sophisticated AI systems can use a process called "super-resolution" to improve the detail of lower quality images. This ability to predict and create missing information sometimes leads to outcomes that appear sharper than those taken by high-end cameras. It does make one wonder how far this technique can be pushed and its future implications.
Blending descriptive language with technical photography terms within prompts can greatly improve realism. We've noticed that AI systems, particularly those trained on photographic data, respond better to specifics like aperture settings (f-stop) or ISO levels. It’s exciting to see the synergy between human language and technical parameters within this space.
Watermark removal in AI-generated imagery is a topic that needs careful consideration. While algorithms can certainly generate coherent images without watermarks, it's crucial to acknowledge the potential ethical and copyright issues surrounding the original work. There’s a growing need to explore the boundaries of responsible use of this technology.
Different AI models have distinct strengths. For instance, some excel at generating landscapes, while others are tailored for portrait photography. Understanding these specializations allows users to refine their prompts and optimize the results. It’s quite similar to selecting the right tool for a specific job in traditional photography.
AI tools can also help with common photography imperfections. Things like lens distortion or color inconsistencies can be automatically corrected, simplifying the creation of professional-looking results. It's remarkable to see how accessibility to AI-driven tools democratizes a certain level of quality previously seen as needing years of technical experience.
Employing "clip-guided" approaches can refine the resolution of AI images. These methods allow the AI to focus on particular elements of a scene while preserving overall coherence. It’s fascinating to observe how such techniques can lead to outputs that are not only clearer but also more aesthetically pleasing.
The impact of factors like mood, scene, and even weather within the prompt dramatically influence the outcome. It's analogous to how atmospheric conditions shape photography in the real world. It emphasizes the deep integration of complex real-world factors that now can be expressed in text and translated into AI image generation.
The use of generative adversarial networks (GANs) in some AI models presents a unique way of producing images. Two networks compete to refine realism, resulting in imagery that embodies a comprehension of visual aesthetics often missing in more basic editing techniques. The use of GANs is something to watch as its theoretical foundation appears to be very promising in future generations of AI models.
AI Image Generation in 2024 A Deep Dive into Prompt Engineering Techniques - Advanced Techniques for AI-Powered Image Upscaling
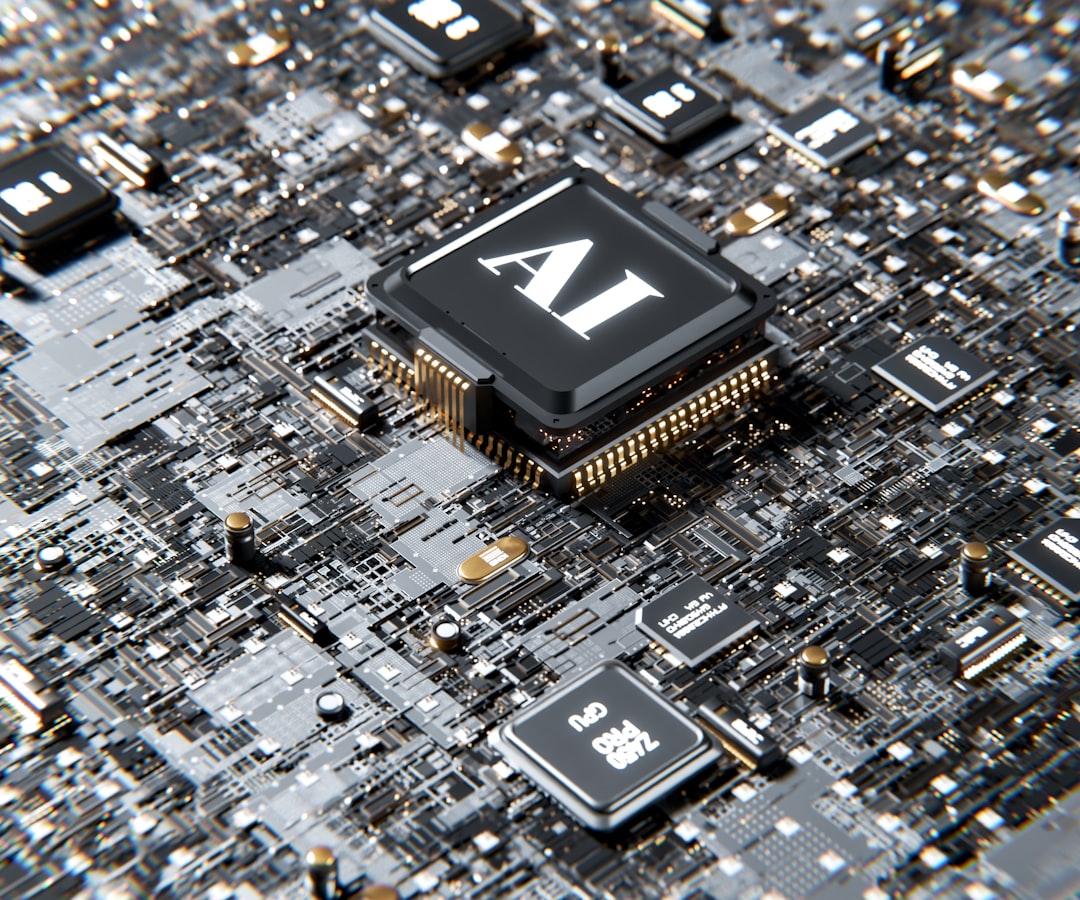
The field of AI-powered image upscaling is experiencing a surge in advanced techniques, offering a new level of image enhancement. These methods utilize sophisticated algorithms to analyze the content of low-resolution images and intelligently predict missing details, effectively reconstructing high-resolution versions. The quality gains can be dramatic, with some AI upscalers boasting the ability to increase image size and quality by up to 800%. This is a significant leap beyond traditional image resizing methods. Furthermore, the availability of tools that perform these operations locally, without requiring an internet connection, expands accessibility for creators. Additionally, some tools are designed to not only upscale images but also correct defects and enhance various features in a single process. This specialized approach further emphasizes the trend towards creating tailor-made image enhancement solutions that cater to diverse needs. The rise of AI-powered image upscaling presents a fascinating intersection of technology and artistry, fostering new conversations regarding the nature of realism in digital images and the creative potential that these advanced tools offer. However, as this field matures, it is important to address related issues, such as copyright and ethical implications, as we consider the broader implications of AI-driven image enhancement.
AI-powered image upscaling has evolved significantly, leveraging advanced algorithms to analyze image content and extrapolate missing high-resolution details from lower-resolution originals. Some techniques can even increase image size by a factor of 16 without introducing noticeable distortions, unveiling fine details that might be missed by even top-tier cameras. This capability hinges on the algorithms' ability to recognize patterns and textures within the image, using this information to intelligently predict what the missing details should be.
Beyond simply enlarging images, AI upscalers are being used to improve the quality of photos taken in challenging conditions. For example, AI models trained on vast image datasets can significantly reduce noise in high ISO images, a common issue when shooting in low-light environments. This allows photographers to recover detail and clarity from shots that might otherwise be discarded.
One of the interesting developments in this field is the shift towards perceptual loss functions. Rather than focusing solely on pixel-perfect accuracy, these functions prioritize how humans perceive image quality. This approach has resulted in images that are not just technically sharper but also appear more lifelike and visually appealing.
Moreover, AI can now address a variety of image imperfections. Whether it's combating the compression artifacts common in JPEGs, removing pixelation in low-quality images, or revitalizing old photos, these tools offer an incredible degree of restoration. The way AI reconstructs lost information to achieve this is remarkable and offers exciting possibilities for preserving our visual history.
Incorporating 'style transfer' during upscaling is also becoming more common. By learning stylistic elements from reference images, AI can enhance resolution while maintaining the original image's artistic essence. This approach ensures that the upscaled images retain their unique character, resulting in a more pleasing and natural look.
Furthermore, edge-preserving algorithms are being used to maintain clarity and sharpness throughout the upscaling process. By selectively enhancing edges and contours, these methods address the common issue of blurriness that can accompany enlarged images, offering a more refined output.
Interestingly, some AI models have demonstrated a remarkable ability to produce upscaled images that align with human evaluations of quality up to 90% of the time. This indicates the ability of AI to learn and optimize visual elements that appeal to our aesthetic sensibilities. It’s intriguing to consider the implications of this level of alignment between AI perception and human preferences.
Generative adversarial networks (GANs) have shown promising results in image enhancement as well. These networks, composed of two competing neural networks, can simultaneously refine both the texture and structural details of an image, potentially leading to a greater sense of depth and realism in the output.
Additionally, multi-scale attention mechanisms are increasingly being incorporated into AI upscaling tools. These mechanisms allow for a more granular approach to image enhancement, allowing the AI to adjust different aspects of an image independently, like foreground and background details. It seems that these techniques are essential for achieving truly nuanced enhancements.
Perhaps most excitingly, some AI upscaling systems can now learn from user feedback. As they are used, they adapt and refine their enhancement capabilities to better match individual preferences. This personalized approach creates the potential for AI upscalers to become increasingly tailored to our individual tastes and needs. It is indeed intriguing to observe how this aspect of AI development could transform the future of image enhancement and potentially open the door to even more specialized applications.
AI Image Generation in 2024 A Deep Dive into Prompt Engineering Techniques - Watermark Removal Strategies Using AI in 2024
The landscape of watermark removal has seen notable advancements in 2024 thanks to AI. We're now seeing readily available online tools like Removebg and Imggen AI that make it easier than ever to remove watermarks without a lot of fuss. These tools are designed to keep the image quality high, which is a good development in this field. More specialized AI techniques, like self-supervised convolutional neural networks (CNNs), are starting to be applied to these problems. One of the major advantages of these methods is that they don't always require a reference image, which improves how well they work in a wider variety of situations. Another aspect is how well AI can now restore backgrounds after watermarks are gone through a process called inpainting. This has led to significant improvements in the quality of the final images. It's worth mentioning that the ethics of watermark removal still creates some uncertainty, especially when it comes to copyright. It's crucial to use these AI tools responsibly and consider the potential for misuse or copyright infringement. There is definitely more to explore in this area.
The landscape of watermark removal is rapidly evolving, thanks to the integration of AI in 2024. We're seeing a shift towards more sophisticated strategies, with many tools now relying on deep learning. These systems are trained on large datasets of watermarked and watermark-free images, developing a strong understanding of the distinct characteristics of each. This allows for more precise removal, essentially reconstructing the portions of the image obscured by the watermark.
Additionally, AI models are becoming increasingly adept at recognizing the unique patterns and distortions caused by diverse watermarking techniques. This recognition, combined with an understanding of how watermarks interact with the original image data, permits targeted removal without compromising image integrity. The rise of CNNs in this field has been particularly impactful, enabling more refined pixel-level transformations that result in sharper, cleaner outcomes.
Inpainting, a fascinating approach used by certain algorithms, involves filling in the gaps left by removed watermarks with context derived from surrounding pixels. It's remarkable how well this technique can restore an image, often making it indistinguishable from the original. Furthermore, the incorporation of generative adversarial networks (GANs) is demonstrating promising results. Here, two neural networks compete—one generating realistic content and another evaluating it—ultimately leading to more coherent and artifact-free watermark removal.
Another noteworthy development is the emergence of real-time watermark removal. Certain AI solutions can now process images during capture or even in the midst of editing, providing incredible flexibility, especially for content creators on the go. Interestingly, the evaluation of watermark removal techniques is also changing. We're seeing a stronger focus on aesthetic quality, alongside resolution retention. AI systems are now benchmarked based on how humans perceive the resulting images, ensuring the outcome aligns with our visual preferences.
However, these advancements raise some serious questions regarding ethics and legitimate usage. As the lines between responsible application and potential misuse blur, the discussions around watermark removal and copyright become increasingly critical. Especially in commercial photography and content ownership, these considerations are paramount.
Beyond core removal strategies, advancements in post-processing are also playing a role. Techniques like edge-preserving filters and gradient-based methods enhance the precision of watermark removal, ensuring finer details aren't lost in the process. This improved fidelity can yield results surpassing traditional techniques. Finally, the inclusion of user-configurable settings within many AI watermark removal tools empowers photographers to take control. They can customize the intensity and method of removal to address specific image requirements, allowing for a balance between artistic integrity and watermark management. Overall, watermark removal powered by AI in 2024 presents both exciting opportunities and complex challenges for the field of image manipulation.
AI Image Generation in 2024 A Deep Dive into Prompt Engineering Techniques - Enhancing Low-Light Photos with AI Algorithms
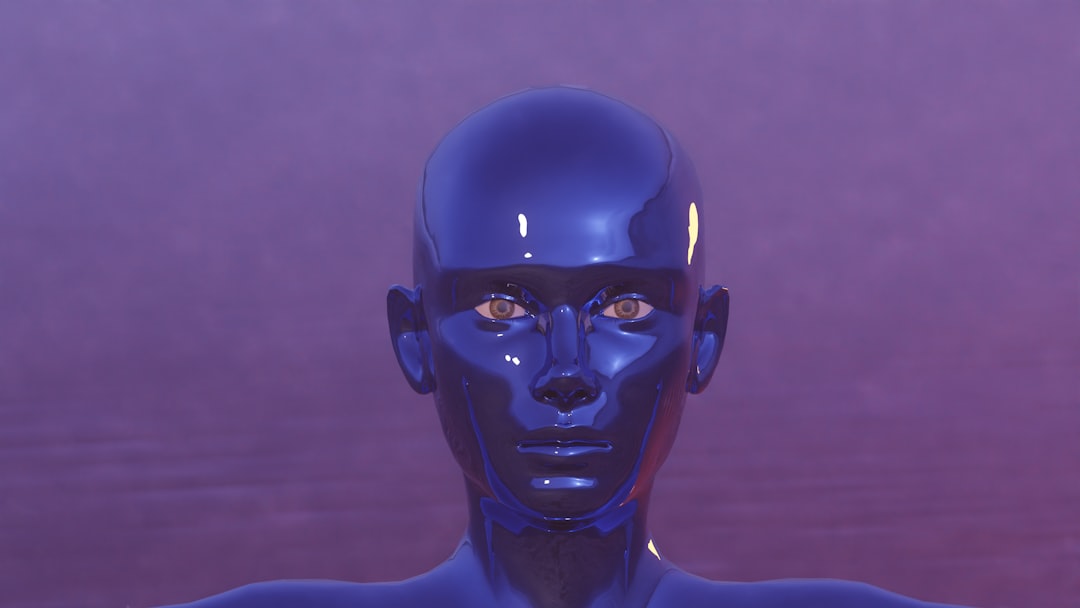
AI algorithms are increasingly adept at enhancing images captured in low-light conditions, a crucial development within the broader realm of AI image generation. These algorithms often leverage generative adversarial networks (GANs), a technique where two networks compete to refine the image, producing more natural-looking results. Specialized AI models have also been developed specifically for this task, addressing the challenges of sensor limitations and inadequate lighting that often lead to grainy or poorly defined images.
The choice of loss functions in these algorithms is important as well. Some methods, like the Charbonnier loss, are better at managing the discrepancies between the input (the low-light image) and the desired output (a brighter, clearer image). This helps the AI to produce enhancements that maintain visual appeal and clarity without introducing excessive artifacts.
The future of low-light AI image enhancement appears promising. There's a push to not only increase brightness and reduce noise but also to improve the overall aesthetic quality of these images, making them more visually pleasing to the eye. While the technology is rapidly advancing, the computational demands of these techniques are still a concern. Furthermore, AI models often need to be tweaked for particular situations, highlighting the need for greater optimization in specialized uses like edge vision. Despite these hurdles, low-light AI photo enhancement is a fascinating field that shows great potential for both amateur and professional photographers.
The realm of low-light photography has seen significant improvements due to the application of AI algorithms. One of the most noticeable benefits is the ability to intelligently reduce noise in images captured in dim conditions, often exceeding the capabilities of traditional noise reduction techniques. This helps photographers retain finer details in shadows that would usually be obscured by excessive grain.
Another area where AI excels is in expanding the dynamic range of low-light photos. Some algorithms can mimic high dynamic range (HDR) effects by adjusting contrast between the darkest and brightest parts of the image. This can dramatically increase the perceived depth and richness of a scene, striking a balance between shadows and highlights.
Interestingly, some AI systems can leverage temporal data – a series of photos captured in quick succession – to reduce noise by averaging across frames. This approach borrows from astrophotography, where similar methods are used to capture stunningly clear images of celestial objects despite challenging lighting conditions. It’s exciting to see how these concepts are being adapted for everyday photography.
Furthermore, there’s been a shift towards incorporating perceptual quality metrics into AI-driven image enhancement. Instead of relying solely on pixel-level analysis, these metrics assess how humans perceive image quality. As a result, enhanced images are not only technically sharper but also more visually appealing.
The application of deep learning has also enabled AI to more accurately correct the color casts that often occur in low-light images. By carefully analyzing the content and context of the photo, these algorithms provide refined color adjustments, ensuring the final output more closely matches the natural colors of the scene.
It’s becoming increasingly common for AI to handle low-light image enhancements in an end-to-end workflow. This means noise reduction, upscaling, and color correction can all be performed simultaneously, simplifying the editing process for photographers.
The development of AI models that can learn from user preferences is also fascinating. As users interact with these systems, the algorithms adjust and refine their enhancement methods, creating a personalized experience that caters to individual styles. This adaptive capability is a strong indicator of how AI might further evolve in this field.
Advanced algorithms are also starting to use rerendering techniques to interpret low-light photos in new ways. They can generate versions of the same photo that capture a specific mood or emotion more effectively. This is a remarkable ability that can lead to photos that are more evocative and engaging to viewers.
Some AI models use spherical analysis to identify potential light sources within a scene. This knowledge of where light is originating from enables more precise adjustments in shading and highlight enhancement, resulting in images that appear naturally lit.
Finally, AI is becoming highly adept at extracting and applying unique features within a photo during the enhancement process. By understanding the relationship between these features and the overall aesthetic qualities of an image, the algorithms are able to enhance clarity while retaining the original character of the photograph. This ability to carefully consider visual elements suggests that the future of AI-powered low-light image enhancement is very promising.
AI Image Generation in 2024 A Deep Dive into Prompt Engineering Techniques - AI-Assisted Color Grading and Tone Mapping
AI-powered color grading and tone mapping are transforming how we edit and enhance visual content in 2024. These technologies streamline the color correction process by automating previously manual tasks, offering increased accuracy and speed for colorists of all skill levels. The ability to quickly apply consistent color palettes across multiple scenes is a particularly notable development, making it simpler to achieve a unified look and feel for a project. Tools designed specifically for color grading, like DaVinci Resolve, are integrating AI capabilities, bringing advanced color correction techniques within reach of a broader user base. While the speed and precision offered are exciting, this shift in post-production also introduces important questions about the role of human creativity in film and the evolving nature of visual storytelling. The future of film aesthetics is clearly being impacted by these tools, and it remains to be seen how the creative process will continue to evolve in response to this innovation.
AI's foray into color grading and tone mapping has introduced a new level of finesse to image manipulation. It's fascinating to observe how these tools can go beyond simple color adjustments, now able to analyze the emotional impact of colors within a scene. This opens up possibilities for matching color palettes that not only look good but also evoke specific feelings in the viewer. For instance, AI could help generate a more melancholic tone for a portrait by subtly shifting color balances.
Beyond emotional responses, AI can also significantly extend the dynamic range of images. It's impressive how AI can analyze an image and reconstruct missing details in both the shadows and highlights, achieving a similar effect to high dynamic range (HDR) photography. This can significantly improve the sense of depth and detail in an image, particularly when dealing with scenes that have high contrast or challenging lighting conditions.
Another interesting development is the growing ability of AI tools to learn and adapt to individual photographers' styles. This adaptive capability is quite unique and potentially very helpful. The AI observes your past color grading choices, allowing it to anticipate and recommend edits that align with your established workflow and aesthetic sensibilities. This kind of personalized approach can potentially save a lot of time and effort for anyone who has a clear visual language in their work.
While these improvements are encouraging, the field is still evolving. Studies show that AI can achieve a considerable level of aesthetic alignment with experienced human colorists, but there's always room for refinement. It's encouraging to see AI models getting closer to our own subjective notions of beauty in photography.
However, one of the truly exciting areas is the increasing ability of AI to understand the semantic context of images. It's not just about colors anymore; AI can now, in some cases, grasp the underlying themes or narratives in the scene. This means it can suggest grading that is more coherent with the subject matter. For instance, it might apply warmer tones for a sunset scene or cooler tones for a more formal portrait.
Furthermore, AI can identify and correct certain color imperfections that have historically been challenging for traditional editing techniques. Color banding, particularly noticeable in gradients, is one issue AI is excelling at resolving. By using machine learning to predict and smooth out these transitions, AI tools can result in smoother, more natural-looking gradients.
Interestingly, the introduction of AI has brought a new level of interactivity to color grading. Some tools now offer real-time feedback, allowing the user to see the impact of adjustments in real-time while editing. This level of interaction can dramatically speed up the editing workflow, streamlining the entire post-processing stage of photography.
In addition to general enhancements, AI color grading systems can offer scene-specific guidance. They can leverage a wealth of information about natural color theories, recommending color palettes that harmonise with a specific environment – a beach scene, an urban streetscape, a portrait in a studio, etc. This type of guidance can strengthen the visual narrative of a photo and enhance its ability to tell a story.
The future of color grading looks exciting with AI models that can even analyze time-lapse sequences. It's impressive to see the potential for color consistency in these types of projects, where lighting conditions can change dramatically over the course of the filming. Maintaining a consistent aesthetic across different time periods with dynamic lighting conditions was historically a challenge.
Finally, the push toward non-destructive workflows is important for all photographers. AI tools are increasingly adopting methods that allow edits to be applied as layers, permitting adjustments and reversions without damaging the original image file. This is a valuable feature for maintaining the integrity of our photography workflow.
While the technology is maturing rapidly, there's still a lot to discover. The future of AI-assisted color grading and tone mapping will undoubtedly involve ongoing development and refinement. It's a very dynamic area and it will be interesting to watch how AI and human creativity continue to intertwine.
AI Image Generation in 2024 A Deep Dive into Prompt Engineering Techniques - Exploring Style Transfer for Artistic Photo Manipulation
Within the evolving landscape of AI image generation in 2024, exploring style transfer for artistic photo manipulation offers a compelling avenue for creative expression. This technique involves merging the artistic style of one image onto the content of another, effectively allowing artists to transform photographs into unique visual interpretations. AI algorithms play a key role in this process, meticulously analyzing aspects like brushstrokes, color palettes, and textures from a chosen "style image" and applying those qualities to a "content image." This gives artists a remarkable level of control, enabling them to seamlessly blend diverse aesthetic influences into their work without necessarily possessing traditional art skills.
While the potential for creative exploration is significant, the reliance on neural networks in style transfer also introduces complex considerations. It raises questions about the degree to which AI-driven processes might influence or even dictate the artistic choices made during image manipulation. The ability to effortlessly alter the look and feel of a photo, while empowering, prompts reflection on the role of human creativity within this new technological framework. As style transfer becomes more refined, the nature of artistic authorship within the realm of digital art becomes increasingly nuanced and deserving of further examination.
AI-driven style transfer has become a powerful tool for artistic photo manipulation in 2024, leveraging convolutional neural networks (CNNs) to dissect and apply stylistic elements from one image to another. This allows photographers to infuse their technical skills with artistic influences, essentially blending their own vision with the essence of renowned artworks, leading to a broader spectrum of creative expression.
It's surprising how effectively these models can capture and reproduce the complex patterns and textures of a source image, effectively transforming a mundane photograph into a stylistic rendition reminiscent of famous paintings while still maintaining the core visual elements of the original image. The process isn't just about aesthetics though; style transfer is increasingly incorporating semantic understanding, meaning the AI now strives to apply styles that reflect the intended message behind an image, a significant step in the direction of deeper image interpretation.
Some AI applications are now incorporating generative adversarial networks (GANs) into their style transfer workflows. These tools enable a dynamic evaluation and refinement of images, bringing a more iterative approach to the creative process. The AI effectively 'learns' from user interactions and adapts accordingly, leading to a more sophisticated outcome over repeated applications.
The ability to adjust the intensity of the style transfer in real-time has fostered new creative methods. Photographers can now experiment with diverse artistic styles directly within their editing environment, representing a seamless blending of technology and art. This hands-on approach offers a degree of flexibility that wasn't possible with traditional editing techniques.
Interestingly, advancements in AI have allowed for more precise control over the application of styles during the transfer process. Many tools are able to differentiate between foreground and background components, ensuring the main subjects remain crisp while stylistic adjustments are applied more liberally to the backdrop, effectively creating a nuanced depth of field within the image.
Style transfer tools are also demonstrating contextual awareness, allowing AI to alter the type of stylistic elements applied based on the image genre. For example, styles can be adjusted for landscapes, portraits, or urban scenes, ensuring a more harmonious aesthetic interpretation of the photograph.
The potential for merging multiple styles in a single image is also being explored, where AI blends various artistic elements to produce a hybrid effect. These combined styles can maintain visual coherence while producing unique compositions that would be challenging to achieve through traditional methods.
Furthermore, the growing integration of style transfer into educational platforms provides aspiring photographers with unprecedented access to artistic experimentation. Individuals can explore diverse styles from established artists, gaining an intuitive understanding of those techniques without the requirement of extensive training or prior photographic knowledge.
The implications for historical photo restoration are also noteworthy. Style transfer tools can now help recreate missing textures and details in aged photographs, bringing old images back to life in a meaningful way. This approach breathes new life into these visual records while ensuring their authenticity and historical context remain intact.
As AI-powered style transfer continues to evolve, its impact on the realm of image manipulation and creative expression will only continue to grow. It's a fascinating blend of technology and art that is rapidly redefining how we perceive and interact with visual content.
More Posts from colorizethis.io: