AI Photo Editing in 2024 How Machine Learning Revolutionizes Image Enhancement
AI Photo Editing in 2024 How Machine Learning Revolutionizes Image Enhancement - AI-Powered Automatic Background Replacement
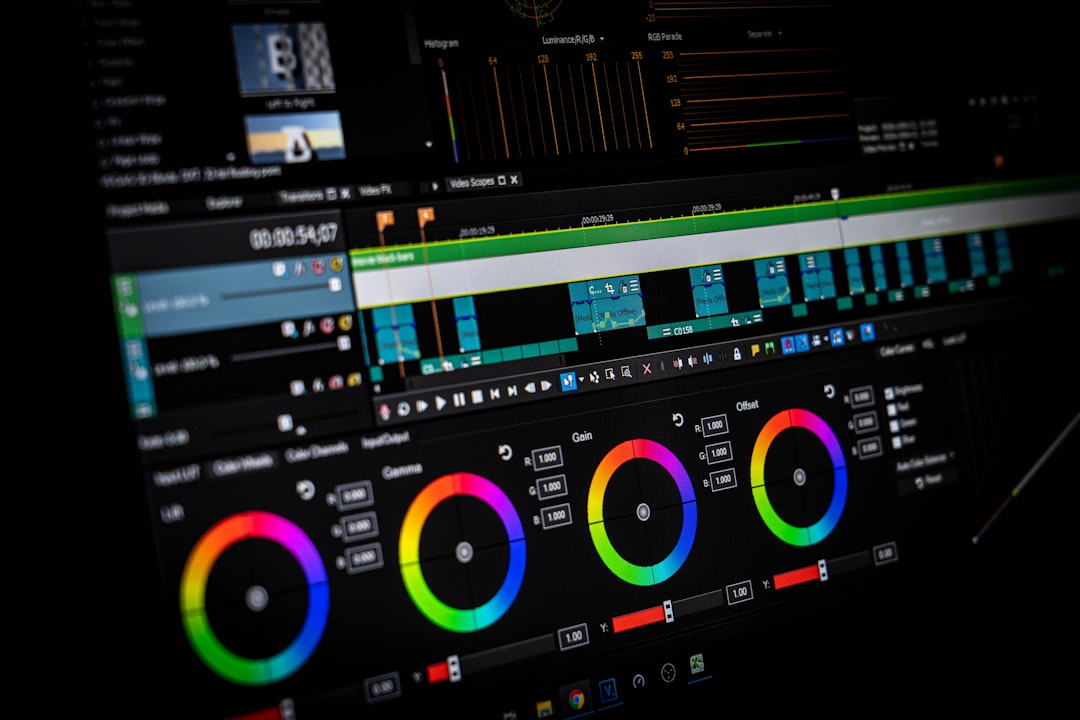
AI-powered automatic background replacement is a game-changer in photo editing. It's not just about removing backgrounds; it's about giving users the power to transform images with ease and control. Tools like LightXEditor and Clipdrop are taking this technology a step further, letting users seamlessly swap out backgrounds while retaining the natural look of the subject. These AI tools are designed to be user-friendly, offering a solution that’s accessible to everyone, from casual photographers to seasoned professionals. This level of accuracy and user-friendliness is a testament to how far machine learning has come in image editing. And with ongoing advancements, we can expect even more sophisticated and versatile background replacement tools to emerge in the future.
It's fascinating how AI is tackling the challenge of automatically replacing backgrounds in images. The foundation lies in convolutional neural networks (CNNs), which excel at segmenting and identifying objects within an image. This allows for precise isolation of the subject from its background, paving the way for a seamless replacement.
However, one of the hurdles is achieving accurate edge detection. The AI needs to pinpoint the precise boundary between the subject and background to avoid unnatural transitions and artifacts when swapping backgrounds. That's where sophisticated algorithms come into play, with some leveraging generative adversarial networks (GANs). These networks, in a way, compete with each other, pushing the boundaries of realism in generated imagery, which translates to convincingly replaced backgrounds.
The applications extend beyond still images; real-time background replacement in video frames is becoming increasingly possible. This has implications for everything from live broadcasts to virtual meetings. Texture synthesis techniques, where AI analyzes patterns from existing images, are essential for generating backgrounds that blend naturally with the subject, adding to the visual coherence.
While the training data for these models involves millions of labeled images, the quality and diversity of this data directly impact performance. Recently, depth estimation technologies have been incorporated into background replacement algorithms. This allows the model to comprehend spatial relationships, making the newly inserted backgrounds even more realistic.
Additionally, AI systems can now address lighting discrepancies between the subject and the background. They utilize learned data to adjust color tones and shadows, resulting in a more natural appearance. It's also interesting to note the connection between background replacement and watermark removal. While watermark removal technology is another area where AI is making significant progress, there are ethical considerations regarding copyright and the authenticity of the original content.
Finally, the availability of cloud-based AI solutions for background replacement is a game-changer. This eliminates the need for powerful local hardware, making it possible for even mobile devices to perform complex image processing tasks through lightweight applications.
AI Photo Editing in 2024 How Machine Learning Revolutionizes Image Enhancement - Machine Learning for Advanced Color Correction
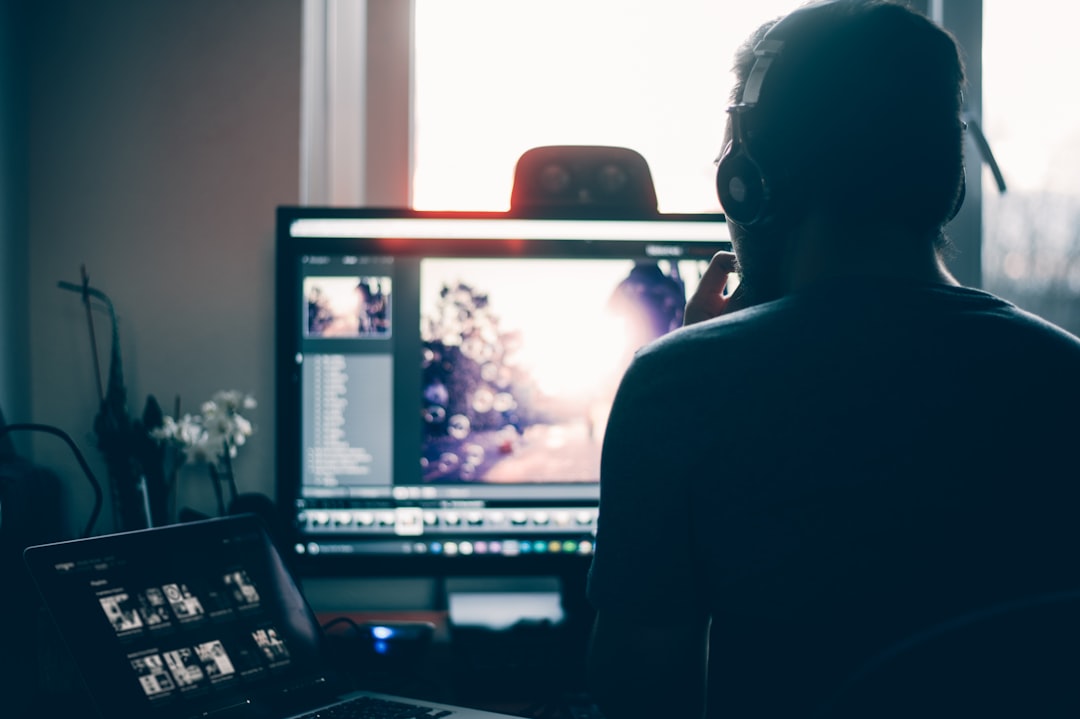
Machine learning is bringing about a revolution in color correction within photography. Advanced algorithms are now able to analyze color variations, enhance saturation, and fix lighting issues automatically. This means that tedious manual adjustments can be done effortlessly. These AI-powered tools are making advanced editing accessible to everyone, even those new to photography, allowing them to vastly improve their images. But, as technology advances, it is important to be cautious of becoming overly dependent on automated solutions, which could potentially diminish the unique artistic flair that comes with individual photo editing. Looking forward, machine learning is expected to continue its evolution, opening up new creative possibilities and further enhancing the editing experience.
Machine learning is taking color correction to a whole new level. We're seeing algorithms that analyze images with pixel-level precision, making adjustments that were previously impossible for humans. They can even learn from existing datasets and adapt to specific images, delivering high-quality results even with limited data. This is changing the way we think about color grading, moving beyond basic adjustments to more nuanced improvements.
One exciting development is the ability to intelligently manage the dynamic range of images. These algorithms understand the context of a photo and make changes to highlights and shadows, creating richer images without losing details in the brightest or darkest areas. They can even go further by enhancing textures in an image based on their analysis of local and global patterns. This gives photos a more natural and realistic look.
Another interesting area is real-time processing, where edits are applied instantly, allowing photographers to experiment with different color adjustments in real time. This is a huge improvement over the traditional workflow where you have to wait for complex calculations to complete.
But we're not just focusing on technical advancements. There's a growing interest in the psychological effects of color. Can machine learning models produce images that evoke specific emotions by understanding how different color combinations impact viewers? This raises interesting possibilities for using color as a storytelling tool in photography.
Of course, there are ethical questions to consider. As AI becomes more sophisticated at color correction, how do we ensure that images aren't being altered in a way that misrepresents reality? This is a complex issue, but one that the field must address as AI plays an increasingly prominent role in image editing.
And as we look to the future, we can expect even more exciting developments in color correction. AI may even integrate with augmented and virtual reality to create dynamic viewing experiences, where colors can shift and adapt to the environment. This could lead to truly immersive photographic experiences, blurring the lines between the physical and digital worlds.
AI Photo Editing in 2024 How Machine Learning Revolutionizes Image Enhancement - Neural Networks Enhancing Image Resolution
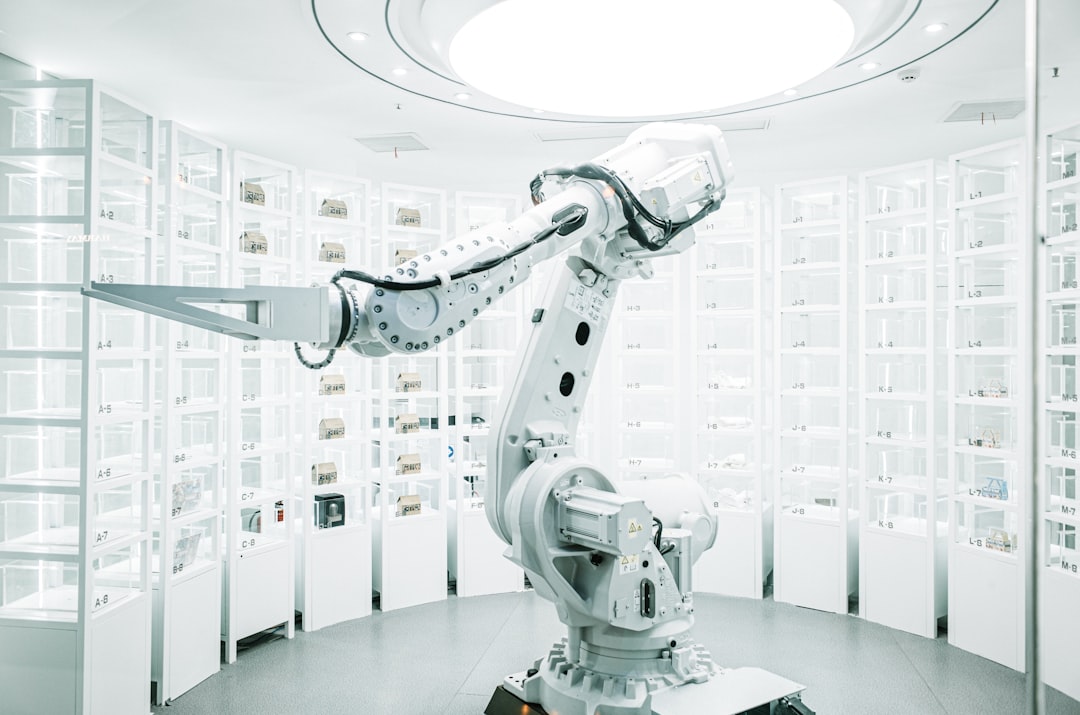
Neural networks are revolutionizing image resolution. They can make even low-resolution pictures look sharper and more detailed. Generative Adversarial Networks (GANs) and Super-Resolution Convolutional Neural Networks (SRCNNs) are two key technologies driving this progress. GANs essentially compete with each other to create realistic images, while SRCNNs use deep learning to learn how to enhance resolution from massive datasets. This is exciting but not without challenges. For instance, sometimes the results can be a little blurry, and getting edges just right is still tricky. As technology improves, we can expect to see even more sophisticated ways to enhance image resolution in the future.
Neural networks are playing a significant role in image resolution enhancement. It's a fascinating field where AI learns to reconstruct high-resolution images from lower-resolution versions. The core of this process lies in "super-resolution" techniques, which use deep learning architectures to infer the missing details in images. These networks excel at analyzing patterns in data, making them far more effective than traditional methods that simply fill in gaps.
One notable player in this field is Generative Adversarial Networks (GANs). These networks feature a constant competition between two parts - the "generator" that tries to create realistic high-resolution images, and the "discriminator" that evaluates those creations. This adversarial training pushes the generator to constantly improve, creating remarkably realistic results.
Traditionally, image quality was measured by how closely the output matched the original pixels. However, neural networks are using "perceptual loss functions" that assess how similar images look to the human eye, leading to enhancements that are more visually appealing and cater to our preferences.
To train these networks, researchers need access to massive datasets containing millions of images. While this approach allows the AI to generalize across diverse settings, it also means that performance can be inconsistent on underrepresented types of images.
However, the field is moving beyond static images. Neural networks are now being used to upscale video content frame-by-frame, a complex process that involves maintaining temporal coherence to prevent jarring inconsistencies. And it's not limited to photographs – these techniques have even been applied to medical and satellite imagery, showcasing their versatility and impact beyond traditional photography.
We’re also seeing real-time image resolution enhancement, a key feature for live broadcasting and instant social media sharing. However, the potential for misuse should not be ignored. While neural networks can remove watermarks, this raises ethical questions about copyright integrity.
Overall, neural networks are opening exciting possibilities for enhancing image resolution. However, these models aren't perfect. They can sometimes introduce unrealistic details, particularly when the network overfits to training data. As with any AI technology, responsible application is crucial to prevent unintended consequences. The future holds great promise, but we must tread carefully as AI continues to evolve.
AI Photo Editing in 2024 How Machine Learning Revolutionizes Image Enhancement - AI Algorithms Streamlining Watermark Removal
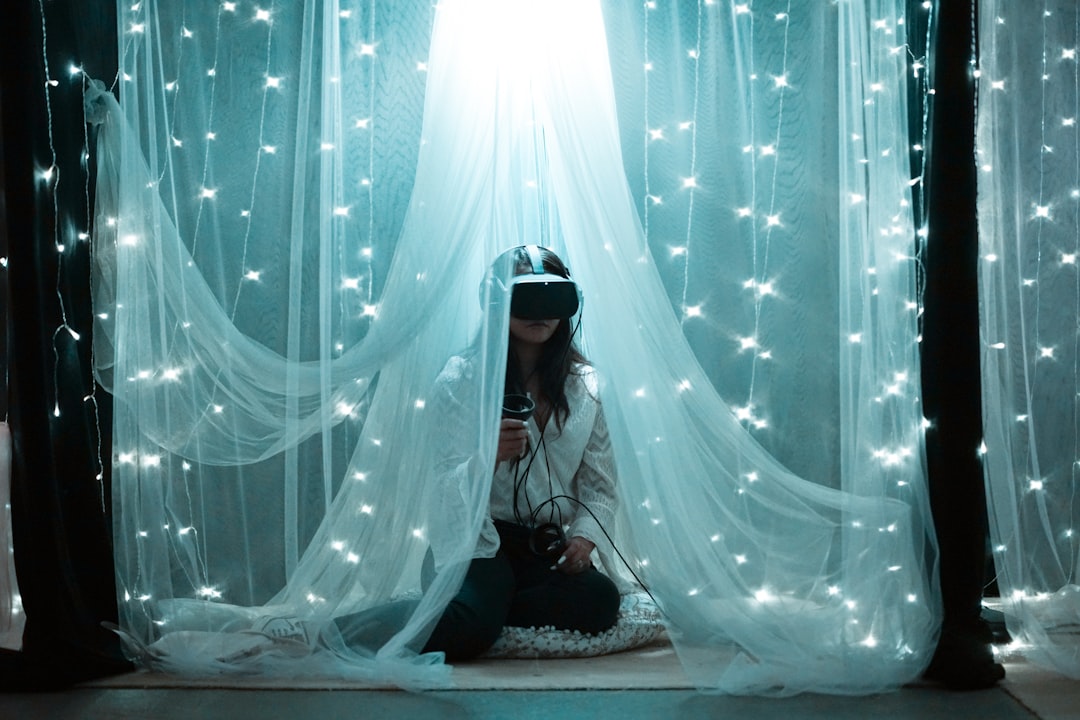
In 2024, AI algorithms are taking watermark removal to a new level. This is a welcome change for anyone who has ever struggled to remove a pesky watermark from an image. Tools like AniEraser and advanced deep learning models have arrived, making it easy to erase unwanted marks while aiming to keep the photo looking natural. These programs rely on impressive techniques like generative adversarial networks and deep image priors, creating results that used to take hours of manual editing. However, the efficiency and quality of these AI-powered solutions also spark questions about copyright integrity and the genuineness of edited content. As image editing evolves, the balancing act between technological progress and ethical considerations will become increasingly important.
AI algorithms are rapidly refining the process of watermark removal. Deep learning techniques, especially convolutional neural networks (CNNs), play a crucial role in recognizing the watermark and the surrounding image, enabling a more accurate removal without disrupting the original image's integrity. Generative Adversarial Networks (GANs), which essentially have two components competing with each other, have become a leading force in watermark removal. One part of the GAN creates the output image, while the other judges its realism. This competition pushes the GAN to create increasingly realistic results, making the removed watermark seamlessly integrate with the rest of the image.
However, the challenge remains in managing any artifacts that may arise due to inadequate training data. These artifacts could be unnatural textures or jarring boundaries. A robust dataset is critical for high-quality results. Inpainting algorithms, which go beyond simply removing pixels and predict the most appropriate content for the watermark’s area by analyzing the image’s context, are at the forefront of this technology.
The application of these algorithms to videos presents a unique set of challenges. Maintaining temporal consistency across the frames is essential; mismatched frames could disrupt the viewing experience.
However, the ethical implications are significant. Using AI for watermark removal without permission raises serious concerns about copyright infringement.
Excitingly, real-time watermark removal is becoming a reality, with benefits for live streaming and instant social media postings.
The diversity of the training data is critical for accurate results. If the model only encounters a narrow range of images, it might struggle with more complex watermark patterns. This limitation needs to be addressed.
Encouraging user control is emerging, giving users the ability to steer the AI's actions during the process. This allows them to customize how forcefully the watermark is removed and the adjustments made to surrounding pixels.
There is also a growing understanding of how the absence of a watermark can have a powerful impact on viewers' emotional responses. This is a fascinating area of research that reveals the intricate connection between visual perception and human emotions.
AI Photo Editing in 2024 How Machine Learning Revolutionizes Image Enhancement - Deep Learning Techniques for Noise Reduction
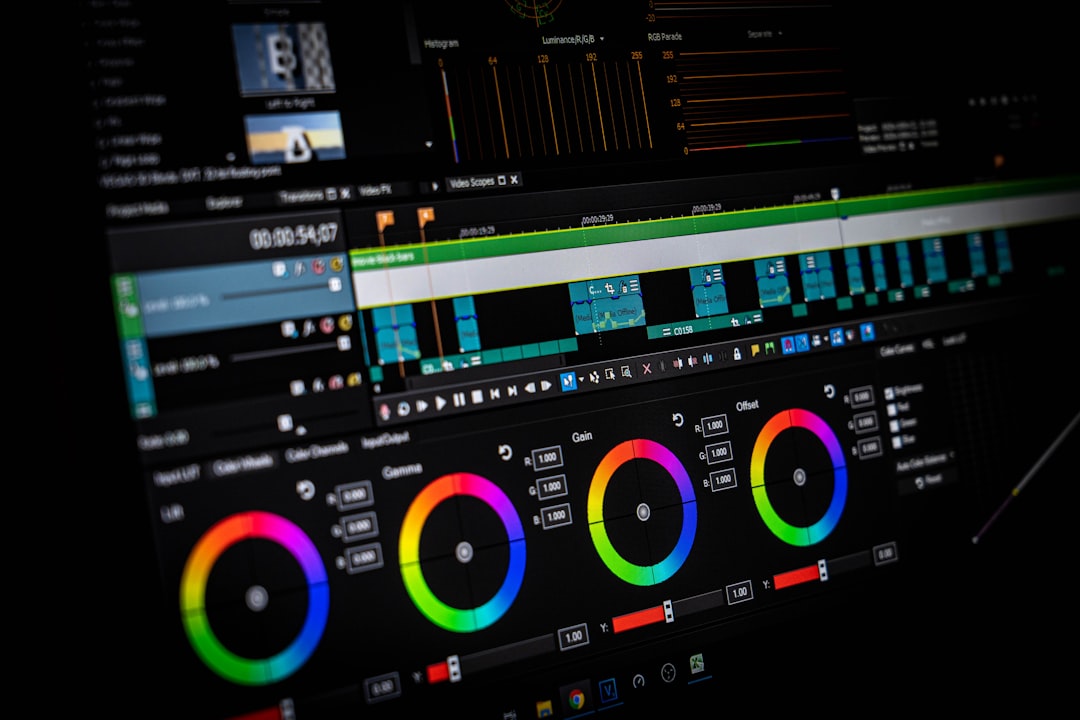
Deep learning is transforming the way we deal with noise in images. It's no longer about simply smoothing things out; these techniques are now capable of understanding the nature of noise and targeting specific types effectively. The ability of deep neural networks to learn complex patterns within images is what allows them to go beyond simple filters and deliver results that feel more natural and realistic.
One area of focus is creating lightweight networks that can perform just as well as larger, more complex models, but with less computational power. This is important for practical applications, especially in real-time processing for live streams or mobile editing. Another trend is the emergence of hybrid approaches, combining different deep learning techniques to optimize noise reduction across a wider range of image types and noise patterns.
However, there's a cautionary note. Sometimes deep networks are made excessively complex, which can actually hinder performance rather than improve it. Striking a balance between network depth and efficiency is crucial for getting the best results. The research community is also still working on establishing clear standards for evaluating the effectiveness of these different techniques. We need a better understanding of which methods work best for which types of noise and image content.
Deep learning is changing the game for noise reduction in images, opening doors to more sophisticated and effective techniques. It's fascinating to see how these models are learning to recognize and address the diverse types of noise that can plague our photos, whether it's from the camera sensor, environmental factors, or even compression artifacts. But the advancements go beyond simply removing noise; they are now tackling the complex task of enhancing the overall visual quality.
One of the most intriguing developments is the use of "perceptual loss functions" in deep learning models. This approach goes beyond the traditional methods that simply analyze pixels, instead focusing on how humans actually perceive images. This leads to results that look more natural, better reflecting our own visual preferences.
Conditional Generative Adversarial Networks (cGANs) are another exciting development. These models are basically trained to "imagine" what a noise-free image would look like, based on the noisy input they receive. This conditioning process allows them to focus on restoring specific features while effectively eliminating noise.
But the future of noise reduction goes beyond still images. Deep learning is now being applied to video sequences, enabling temporal denoising. This means that instead of just working on a single image at a time, these models are learning to maintain consistency across different frames, ensuring a smooth, flicker-free video experience.
The applications extend beyond aesthetics. Techniques like denoising autoencoders are being explored for applications in medical and satellite imagery, revealing the profound potential of deep learning for a wide range of fields.
We're also seeing real-time noise reduction, making it possible to clean up images instantly, with applications for everything from live video streaming to instant social media sharing.
And of course, the exciting field of transfer learning is making waves in denoising. Pre-trained models are now being adapted to new types of noise, significantly reducing the need for extensive data and training time. This opens the door to faster and more efficient noise reduction across diverse contexts.
But as we delve deeper into this world of AI-powered noise reduction, ethical questions arise. The ability to manipulate images so effectively raises concerns about authenticity and the potential for visual information to be misrepresented. It's important to tread carefully and ensure that these powerful tools are used responsibly.
AI Photo Editing in 2024 How Machine Learning Revolutionizes Image Enhancement - Automated Portrait Retouching Using AI Models
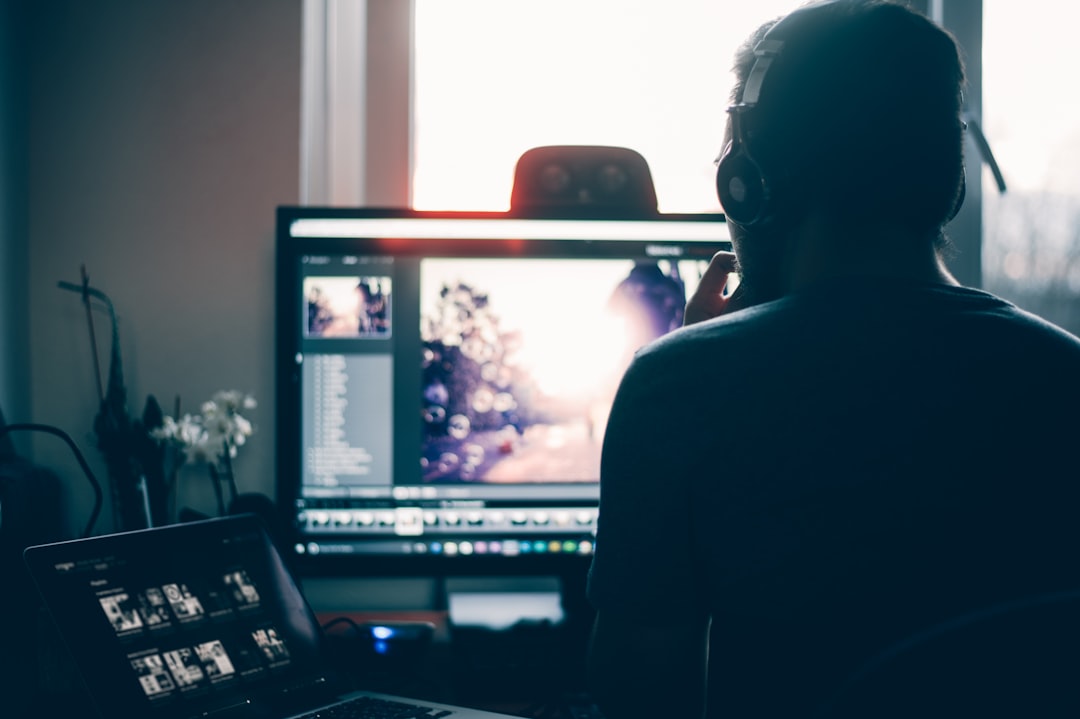
Automated portrait retouching with AI is transforming the way we edit photos in 2024. AI can analyze faces with incredible accuracy, automatically smoothing skin, removing blemishes, and even subtly contouring features. This is a huge leap forward for photographers, making high-quality portrait editing accessible to everyone, regardless of their editing skills. It's incredibly efficient, but raises questions about how far we should go in manipulating images and whether it diminishes the unique artistic vision of the photographer. As AI evolves, we'll have to carefully consider the balance between using these powerful tools and preserving the integrity of our photographic art.
AI portrait retouching is an intriguing field that is rapidly evolving, thanks to the advancements in machine learning. These automated tools can analyze and enhance portraits with unprecedented precision, streamlining a process that used to be incredibly time-consuming. But while the results can be impressive, there's also a lot to think about beyond the technical aspects.
For instance, many of these AI models are capable of real-time processing. This means that photographers can see the retouched image as they are shooting, leading to much more efficient workflows. This opens the door to entirely new approaches to portrait photography. Another interesting aspect is the ability for AI to learn an individual photographer's style and preferences. It's like having a personal assistant who knows your artistic vision and helps you achieve it with each click. These AI models are becoming increasingly sophisticated, recognizing facial features like eyes, lips, and skin texture. This allows for very specific enhancements that maintain the natural look of the subject while still improving their appearance.
However, the emergence of these powerful tools also raises a number of ethical concerns. It becomes even more important to address the line between subtle enhancement and unrealistic manipulation. We need to be mindful of the potential for these AI models to be used to create images that don't reflect the actual subject, particularly as these systems become more adept at generating images on their own. The training data for these AI models also plays a crucial role in their effectiveness. It's essential to use datasets that reflect a diverse range of skin tones, facial structures, and backgrounds to avoid biases and ensure that the retouching results are fair and inclusive.
Generative Adversarial Networks (GANs), which were initially used primarily for image generation, are also being incorporated into retouching processes. These networks involve two competing components - one that generates the retouched image and another that evaluates the quality of the output. This adversarial training helps achieve highly realistic and aesthetically pleasing results. AI-powered retouching tools are also becoming much more sensitive when it comes to skin retouching. Instead of over-smoothing the skin, these new methods retain the subtle texture and details that create a more natural look.
These AI retouching technologies are finding their way into various fields, from filmmaking to medical imaging. While portrait photography is the most visible application, it is only the tip of the iceberg. We're also seeing a move towards user-driven control in AI retouching. Many new tools offer the ability to specify the type and intensity of enhancements, empowering photographers to refine the AI-generated results according to their own vision.
Looking ahead, AI portrait retouching may even be integrated into augmented reality (AR) applications. This could lead to an entirely new way of experiencing and capturing portraits, as users are able to see and adjust their appearance in real time before taking a photo. While the possibilities for AI in portrait retouching are exciting, we must ensure that these advancements are used responsibly. Striking the right balance between AI-assisted enhancement and authentic portrayal will be crucial as we navigate this rapidly changing landscape.
More Posts from colorizethis.io: