Colorize and Breathe Life into Old Black-and-White Photos (Get started for free)
AI-Powered Photo Restoration Reviving Damaged Images in 2024
AI-Powered Photo Restoration Reviving Damaged Images in 2024 - Neural networks advancing photo reconstruction techniques
Neural networks are revolutionizing how we reconstruct damaged photos, using deep learning to tackle the intricate challenges of image restoration. The move from CNNs towards Transformer-based networks demonstrates a clear advancement, as these newer models excel at understanding the relationships between pixels across larger areas within an image, ultimately leading to more refined restorations. This is particularly important in fields like art restoration, where traditional methods often struggle to capture the nuanced details and spirit of the original piece when dealing with faded or physically damaged works. Moreover, modern neural networks show promise in their ability to seamlessly fill in missing parts of images and even selectively remove unwanted elements, hinting at a future where we can better preserve and restore both artistic creations and everyday visual data. However, the need for extensive training datasets continues to be a significant hurdle, implying that this field of research must constantly push forward to find solutions that can be more widely applied.
AI's foray into photo reconstruction has seen significant progress through the use of neural networks. Convolutional Neural Networks (CNNs), in particular, have been instrumental in this advancement, allowing algorithms to predict pixel values based on surrounding information and thereby fill in missing parts of an image. It's fascinating how they can learn to anticipate what's missing, essentially reconstructing a lost visual puzzle piece by piece.
While traditional methods often fall short when trying to restore the original essence of images, especially when dealing with extensive damage, neural network approaches can often surpass them in accuracy. Certain studies have shown promising results with neural networks achieving remarkably better outcomes in restoring intricate details from severely deteriorated photographs. This speaks to the potential of neural networks to overcome the limitations of older techniques.
The development of super-resolution techniques, which leverage the power of neural networks to upscale low-resolution images, further showcases the capabilities of these systems in image restoration. This is a very useful aspect, especially in situations with images that were never taken with high resolution to begin with.
It's worth noting, though, that the effectiveness of these neural networks relies heavily on the quality and quantity of the training data they are provided. Larger datasets with examples of various damage types enable these networks to learn a wide range of patterns, which ultimately leads to better results. This can also mean that, in certain scenarios, if the data to train the system does not appropriately represent the problem it may lead to less desirable results.
A notable aspect of these systems is their ability to learn from user interactions. This suggests an evolving field of AI-driven restoration that could adapt to users' feedback and preferences, moving beyond a simple one-size-fits-all approach. This capability could eventually lead to personalized and more accurate restoration outputs, and that's a pretty remarkable possibility.
However, these neural networks aren't without their limitations. The introduction of artificial details (artifacts) in the final image is a recurring issue that researchers are actively tackling. Refining these approaches to minimize such undesirable elements remains a crucial area for further research. I believe that finding ways to better understand the nature of these artifacts will help us to create systems with even more desirable outputs.
AI-Powered Photo Restoration Reviving Damaged Images in 2024 - Automated scratch and crease removal algorithms
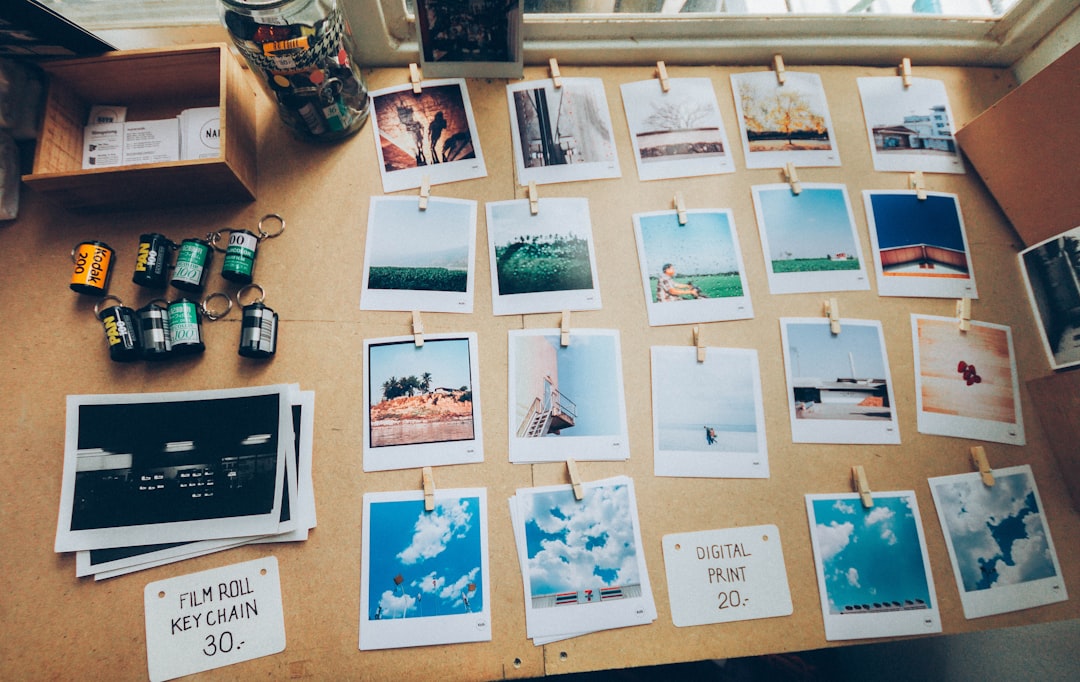
Automated scratch and crease removal algorithms are becoming increasingly important within the field of AI-powered photo restoration. These algorithms are designed to efficiently identify and repair various types of damage found in old or damaged photos, including scratches, creases, and tears. Their ability to automate tasks previously done manually makes the process of restoring images faster and potentially more accessible.
While these algorithms represent a significant step forward in photo restoration, it's important to acknowledge that they are not without limitations. There's always the possibility that the automated repairs introduce new problems, like unwanted artifacts or alterations that change the original feel of the photo. It's a challenge to balance the speed and ease of use provided by these algorithms with ensuring the restored image remains faithful to the original.
Researchers are continuing to improve these algorithms, aiming to develop more sophisticated methods that can better differentiate between real details and imperfections, leading to more accurate and aesthetically pleasing restoration outcomes. This ongoing work suggests that the future of AI-powered photo restoration will see further advancements in the ability to delicately handle the delicate task of image restoration without introducing unwanted distortions.
Automated scratch and crease removal algorithms are becoming increasingly integrated into AI-powered photo restoration tools, offering a more efficient way to revive damaged photos compared to traditional methods. These algorithms often rely on sophisticated edge detection techniques to pinpoint imperfections within an image, distinguishing them from simple enhancement techniques that might impact the entire picture. This targeted approach helps ensure that the removal of scratches doesn't unintentionally affect the underlying image quality, which is crucial for preserving the authenticity of the original photograph.
Many of these algorithms employ a technique called frequency domain analysis, a clever approach that transforms the image into a different mathematical representation. In this new space, scratches and creases stand out as distinct frequencies, which can then be selectively filtered out. This filtering process often leads to more effective restoration outcomes compared to simply manipulating the pixel values directly.
Interestingly, machine learning models are being trained to recognize various kinds of damage by analyzing pixel patterns and textures. This ability allows the algorithms to make subtle distinctions between deliberate features in the image (like brush strokes in a painting) and unwanted artifacts. This nuanced understanding is essential for achieving high-fidelity restoration.
We've also seen a remarkable improvement in the computational efficiency of these algorithms. Many systems can now process high-resolution images in real time, making the entire restoration process significantly faster. This acceleration not only streamlines the restoration workflow but also opens up new possibilities for more interactive user experiences during the restoration process.
Some cutting-edge algorithms are even able to predict the original appearance of damaged areas by intelligently analyzing surrounding pixel information. This prediction capability, drawing inspiration from how neural networks learn from patterns, can greatly reduce the need for manual adjustments, thereby speeding up the process further.
It's important to recognize that not all algorithms are created equal, and the effectiveness of a given algorithm can vary significantly depending on the nature of the damage. For example, high-frequency scratches may require a different approach compared to low-frequency creases, showcasing the intricate challenges involved in optimizing image restoration.
Another interesting aspect is the capacity for these automated algorithms to learn from user feedback. Many systems use a feedback loop to incorporate user corrections, allowing them to continually improve their restoration capabilities. By integrating user input, these AI systems can refine their understanding of what constitutes a successful restoration, leading to more personalized and tailored outputs over time.
The application of deep learning, especially convolutional neural networks (CNNs), has shown promise in reducing the occurrence of artificial elements or artifacts in the restored images, compared to older restoration techniques. This is due to the ability of these neural networks to consider the context of each pixel in relation to its neighbors, rather than just treating them as isolated entities.
However, there's a lingering challenge: finding a balance between automated restoration and artistic intent. While these algorithms excel at technically restoring images, the task of differentiating between genuine imperfections and deliberate stylistic choices (like visible brush strokes in a painting) remains a domain where human judgment is still essential.
Despite the advancements made in automated scratch and crease removal, these algorithms still encounter limitations when faced with extremely damaged photos, particularly those with significant color fading or deep textural changes. This limitation highlights that while automated methods are very powerful, they serve as valuable tools to enhance traditional restoration techniques rather than replace them entirely. This signifies that the best restoration outcomes are often achieved when combining the capabilities of AI and the experience of a skilled human restorer.
AI-Powered Photo Restoration Reviving Damaged Images in 2024 - Color restoration breakthroughs for black and white images
The ability of AI to restore color to black and white photos has seen remarkable advancements. Algorithms are becoming increasingly sophisticated in their capacity to analyze images and predict the most likely original colors. These methods use deep learning to examine the content of a photo and then intelligently apply colors, breathing new life into old photographs. Several user-friendly tools have emerged that streamline this process, allowing anyone to restore old images with relative ease. However, it's crucial to acknowledge that these advancements come with their own set of challenges. While providing a quick and easy way to colorize old photos, some methods can unfortunately introduce unintended changes to the image, including artificial-looking elements that can detract from the desired result. The ongoing challenge is to create systems that balance speed and convenience with the need to maintain a high level of accuracy and authenticity. Finding the optimal balance between fully automated color restoration and some degree of human involvement is vital to ensure that these valuable images are restored with respect for the original.
The field of black and white image restoration has witnessed exciting advancements in colorization thanks to AI. Researchers are exploring ways to leverage historical information like common color palettes of a specific time period and cultural artifacts to guide the algorithms in selecting appropriate colors, creating restorations that feel more genuine. For example, understanding the dyes and pigments prevalent during a certain era allows for a more accurate representation of the past, bridging a visual gap between then and now.
One promising method involves intricate analysis of how pixels are connected within an image. Algorithms are designed to predict likely colors based on the relationships between neighboring pixels, making use of statistical models to boost accuracy. This approach essentially extrapolates what color might have been present in a faded or missing region.
Another interesting technique is texture recognition. By training machines to understand different textures like skin, fabrics, or natural elements, algorithms can make better-informed decisions about what colors are appropriate. This is crucial for creating depth and believable results, rather than just applying colors in a flat, unrealistic manner.
Some researchers have begun using multi-spectral data for restoration. This involves taking images using wavelengths of light beyond what humans normally see. This hidden data can reveal details not visible in standard photos and offer a wider range of color possibilities, resulting in a more faithful restoration.
More recently, generative models have come into play. These powerful algorithms learn the underlying patterns within images and generate new pixel information based on those patterns. This helps generate more plausible colors, moving beyond the more basic approaches of simply filling in gaps with a guessed color.
There's also a growing emphasis on user interaction. AI systems are increasingly designed to respond to users' color choices, learning from their feedback and making adjustments accordingly. This personalization enables users to fine-tune the results to match their desired aesthetic or understanding of the image's original context.
Image segmentation is also becoming a key technique. By dividing an image into its different components – objects, backgrounds, and so on – the algorithm can apply color in a more context-aware manner. Rather than just coloring the whole image uniformly, it can tailor the color selection to the specific attributes of each region.
It's important to be mindful of the potential for introducing artificial elements. Therefore, methods are being developed to specifically detect and manage these visual artifacts during the restoration process. This focus on artifact management is aimed at ensuring the restored image retains a sense of authenticity while still being visually appealing.
Another fascinating aspect is maintaining temporal consistency across multiple images from the same time period. Algorithms are beginning to be designed to keep the colors harmonious, especially when dealing with a series of photos that tell a story together.
Finally, restoring an image involves considering the historical lighting conditions under which the original photo was taken. Different light sources would have affected the appearance of colors, so understanding these factors helps produce more realistic and authentic restorations.
It's exciting to see how AI-driven color restoration methods are continually being refined. While the technology still faces challenges and isn't perfect, there's clearly a push to improve the accuracy and realism of results. This continuous development of more intelligent and sensitive restoration methods suggests that the future of colorizing historical black-and-white images is very bright.
AI-Powered Photo Restoration Reviving Damaged Images in 2024 - High-resolution upscaling of low-quality photographs
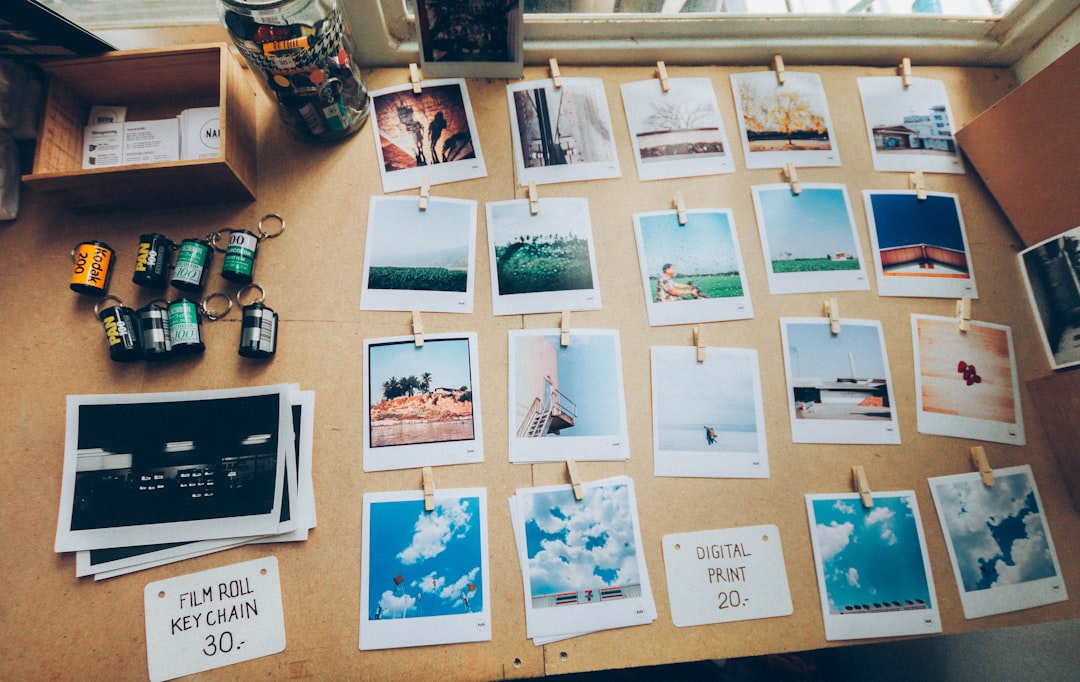
AI-powered photo restoration has seen a rise in the capability to upscale low-resolution photographs to a significantly higher resolution. These systems employ intelligent algorithms that effectively add more pixels to an image, enhancing its clarity and revealing finer details, sometimes reaching resolutions as high as 8K. This ability to transform a blurry, pixelated image into a sharper, more defined one is a key feature of modern restoration tools. Users are able to easily submit images in many common file formats for automated processing.
While these upscaling methods are incredibly fast compared to manual image editing, relying on deep learning introduces a persistent need for large and diverse training datasets to ensure that the algorithms can effectively handle a wide variety of image imperfections. This is crucial for maintaining the integrity of the image and preventing the introduction of undesirable artifacts that can diminish the value of the restoration. While the results can be remarkable, achieving a perfect upscale requires the AI model to have been properly trained on a broad enough set of images. There is still room for improvement in these systems, particularly in refining the process to minimize the introduction of unrealistic or distorted details.
AI-powered image upscaling is transforming how we handle low-quality photographs, effectively increasing their resolution by cleverly adding new pixel information. We can now upload a variety of image formats, like JPG, PNG, and even HEIF, to services that can upscale them to impressive resolutions, even up to 8K. This capability builds upon existing AI restoration methods, expanding their utility beyond simply repairing damage.
It's interesting how these upscalers work. They employ sophisticated techniques like pixel synthesis, where the algorithm learns from surrounding pixels to intelligently guess what new pixel data should be added. However, it's not as simple as just filling in the gaps. The initial resolution of the photo plays a surprisingly important role – images with a bit more detail to begin with tend to yield better upscaled results.
Generative Adversarial Networks (GANs) are becoming essential in improving upscaling. GANs pit two neural networks against each other, with one creating the upscaled image and the other judging its quality. This constant competition leads to the generation of ever more realistic-looking images, significantly improving the restoration process.
One challenge is that upscaling very complex images, like those with detailed fabrics or intricate natural textures, is tougher. This underscores the need to develop specialized algorithms that can understand and enhance these more challenging aspects of images. Unfortunately, upscaling is not a perfect science. Even the best algorithms sometimes introduce visual artifacts, which are essentially distortions or unwanted patterns that emerge during the upscaling process. This highlights the ongoing need to refine how we train and evaluate these models.
It's fascinating how some techniques learn from images at other resolutions, using insights from high-resolution photos to guide the upscaling of low-resolution ones. This cross-resolution learning approach can significantly boost the quality of results. And for those instances where we have a series of photos, time-series algorithms can ensure that the upscaled images maintain a consistent look in terms of color and lighting.
Many of these upscaling methods incorporate sophisticated edge-preserving techniques, essentially prioritizing the sharpness and clarity of lines and transitions between colors and textures. This helps create a more natural appearance in the final image. Furthermore, we are starting to see systems that allow users to provide feedback on the upscaled image, creating a sort of iterative process where the algorithm learns from user preferences. This user-driven improvement makes upscaling even more personalized.
Despite the progress, we still encounter difficulties with severely distorted or very low-quality photos. The path forward for researchers likely involves developing even more advanced model architectures and improving training strategies to handle these tough cases. It's a journey of continually refining these amazing AI tools for restoring and enhancing the visual legacy we capture in our photographs.
AI-Powered Photo Restoration Reviving Damaged Images in 2024 - Machine learning models tackling complex image artifacts
Machine learning models are playing a growing role in tackling the complex issues that arise when restoring damaged images. They offer a more powerful approach compared to older methods, effectively addressing a range of artifacts like noise, blur, and distortions commonly found in deteriorated photos. These models, particularly those based on convolutional neural networks (CNNs) and generative adversarial networks (GANs), are trained on vast datasets, enabling them to differentiate between genuine image features and undesirable artifacts. This, in turn, leads to a greater level of fidelity in the restoration process. While progress has been made, the risk of introducing artificial details remains a concern. Balancing the drive for accurate restoration with the need to preserve the original visual integrity is an area where continued research and development are crucial. Despite the challenges, the use of machine learning in image restoration is progressing rapidly, leading to more sophisticated techniques that can intelligently adapt to the context of each image and better preserve the original visual characteristics.
Machine learning models are making significant strides in tackling complex image artifacts, a crucial aspect of AI-powered photo restoration. These models are becoming increasingly adept at identifying specific artifacts like noise or compression errors, while simultaneously preserving genuine details. This ability to distinguish between actual image features and imperfections is key to accurate restorations.
Several cutting-edge models now leverage generative techniques to create missing pixel data. Instead of simply filling in gaps with estimates, they predict and generate new pixel values, leading to more natural and realistic restorations that maintain a high level of visual fidelity.
Another interesting development is the growing emphasis on context in image restoration. By analyzing the pixels around a damaged area, models can now make more informed decisions about how to fill in missing information, ultimately resulting in restorations that feel more authentic.
Many of the latest models also employ multi-scale processing, a technique that allows them to analyze images at multiple resolutions simultaneously. This multi-faceted approach helps them capture both fine details and larger structures, leading to more thorough artifact removal without compromising image quality.
Interestingly, there's a growing trend towards ensuring temporal consistency in restoring series of images, like film frames or sequential photos. Models are being trained to remove artifacts uniformly across multiple images, which helps maintain the narrative flow and prevents jarring inconsistencies.
Due to the need for vast amounts of training data, researchers are turning to innovative techniques like data augmentation. By artificially expanding datasets through transformations such as cropping, flipping, or adjusting colors, they aim to improve model generalizability and create more robust restoration outcomes.
Some models are even exploring self-supervised learning, a method that allows them to create their own training labels based on the inherent patterns within images. This process helps streamline the training process and can result in models that are better at identifying complex artifacts.
Furthermore, researchers are making a concerted effort to design models that adapt based on user feedback. This means the models can learn from user corrections and adjustments, thereby personalizing the restoration process to better align with individual expectations and aesthetic preferences.
There is also a growing recognition that different artifacts like banding or blurring might require different approaches. To address this, researchers are exploring the development of hybrid models that can intelligently switch between multiple restoration techniques based on the specific type of artifact detected, which can ultimately produce optimized restorations for a broader range of image issues.
However, as these AI-powered tools become more sophisticated, there's a growing discussion surrounding ethical considerations related to authenticity. Restoring historical images with AI inevitably raises questions about the balance between accuracy and embellishment. There is a concern that overly aggressive restoration could inadvertently misrepresent the original image, leading to debates about the role and responsibilities of developers in ensuring truthful representation in historical records. This conversation is likely to continue as the field of AI-driven image restoration continues to advance.
AI-Powered Photo Restoration Reviving Damaged Images in 2024 - Privacy-focused AI restoration tools for personal archives
The field of AI-powered photo restoration is evolving beyond mere image enhancement, with a growing emphasis on user privacy, especially for personal archives. Tools are now emerging that prioritize keeping personal information private while still offering robust restoration capabilities. This means individuals can now revitalize old, damaged images without needing to create accounts or share any personal details. This shift in focus is crucial as concerns about data security continue to grow. These privacy-focused tools are a welcome development, allowing users to confidently restore and preserve their treasured memories without worrying about compromising their personal information. This balance between high-quality image restoration and a respect for individual privacy is a positive indicator of the thoughtful development of AI technologies. It signals a growing awareness that advanced technological capabilities should be built alongside a strong emphasis on ethical considerations, ensuring that our personal histories are safeguarded while we explore the potential of AI for enriching our visual heritage.
The field of AI-powered photo restoration is evolving alongside growing concerns about data privacy. Regulations like GDPR and CCPA are pushing developers to build tools that not only restore photos but also protect the privacy of individuals within them. This has led to a focus on designing algorithms that minimize the exposure of sensitive data during processing.
Some developers are shifting towards processing images directly on user devices, rather than sending them to cloud servers. This "on-device" approach ensures that the original image never leaves the user's control, significantly reducing the risk of data breaches. We're also seeing a change in how user data is used for training. Newer models are offering users more control over what data they share, allowing them to opt-in to specific training programs while retaining control over their own information.
Interestingly, the use of synthetic datasets is gaining traction. These artificially created datasets mimic the characteristics of damaged images, allowing developers to train AI models without compromising actual user data. This approach offers a potential solution to the challenge of training these models without needing huge amounts of real-world examples.
Federated learning is another strategy being explored. This technique lets AI models train on data across multiple devices without the data ever leaving the users' control. It's a promising approach for increasing privacy and minimizing the risks associated with centralized data storage.
Alongside these developments, there's an increasing focus on transparency. Some platforms now offer real-time privacy audits that let users see how their data is being used during restoration. This provides a layer of reassurance and promotes user trust.
Adaptive learning algorithms are also gaining popularity. They can learn from user adjustments without needing to retain the original images, enhancing both restoration quality and privacy.
However, as these tools become more sophisticated, we face challenging questions around the ethical use of AI in historical archives. Restoring personal images raises questions about the balance between enhancing historical accuracy and respecting the original context. Overly invasive restoration techniques could inadvertently distort the historical record, raising ethical concerns.
One complex aspect of this is the use of facial recognition in restoration tools. It highlights the need for thoughtful consideration of consent and user rights when it comes to using images of individuals, further complicating the privacy debate in this area.
Ultimately, the development of privacy-focused AI photo restoration is driving a demand for standards that guide ethical practices within the industry. The goal is to foster a culture of trust where restoration practices are aligned with user expectations regarding their personal information and rights. This is an evolving area, with more questions being raised as technology continues to advance and its applications within personal archives become more widespread.
Colorize and Breathe Life into Old Black-and-White Photos (Get started for free)
More Posts from colorizethis.io: