Colorize and Breathe Life into Old Black-and-White Photos (Get started for free)
Evaluating AI Text-to-Image Models A Comparative Analysis of DALL-E, Imagen, and Midjourney in 2024
Evaluating AI Text-to-Image Models A Comparative Analysis of DALL-E, Imagen, and Midjourney in 2024 - DALL-E 4's Improvements in Photorealism and Prompt Accuracy
DALL-E 4 represents a substantial leap forward in AI image generation, especially in how realistically it creates images and how well it understands user instructions. Its improved ability to translate intricate text prompts into visuals is a major enhancement. Users now experience a greater level of control and satisfaction as the model generates images closer to their intended vision. This version also excels at combining diverse and seemingly unconnected ideas into believable and cohesive images, a characteristic that has always been a hallmark of the DALL-E series.
The relationship between prompt design and image quality remains crucial. Shorter, more open-ended prompts provide room for creativity, while more specific, longer prompts yield precisely targeted results. DALL-E 4 has clearly tackled some of the limitations of previous versions, making it a strong competitor in the field while concurrently addressing concerns about how it interprets and responds to user requests. It's evident that DALL-E 4 continues to push the boundaries of AI's image generation abilities.
DALL-E 4 stands out with its refined approach to light and shadow, generating images that convincingly mimic the way light interacts with objects in the real world. This newfound understanding of illumination significantly boosts the perceived realism of the output. Furthermore, the model's ability to interpret material properties has seen a leap forward, allowing it to render textures with a degree of fidelity previously unseen. We see a noticeable improvement in the capture of details like reflections and surface imperfections, contributing to a more lifelike appearance.
The core language model underpinning DALL-E 4 has undergone a refinement process, leading to a deeper comprehension of the intent behind user prompts. Consequently, the model translates textual instructions into visual output with fewer errors and greater accuracy. It seems the model now grasps the context better, leading to images that more closely adhere to the user's initial description. Interestingly, this also extends to artistic styles. DALL-E 4 appears to have learned to associate specific art styles or genres with corresponding sensory qualities, translating them appropriately based on the prompt.
DALL-E 4 takes a multi-modal approach, incorporating not just text but visual data as well. This means the model can generate images that are much closer to provided examples, improving both the accuracy and relevance of the output. It's as if the model can better translate "show me something like this" into a visual format. The implementation of a feedback mechanism within the generation process represents a notable shift. This allows for real-time adjustments of images based on user preferences, essentially fostering a dialogue between the user and the model and leading to greater interactivity.
Furthermore, the significant increase in processing capabilities enables DALL-E 4 to carry out more intricate scene analysis. This translates into its capacity to build elaborate images with numerous subjects and elements while retaining coherence and clarity, a challenging aspect of image generation. The output resolution has also seen a notable increase, resulting in images suitable for a wider range of applications, including professional contexts where high fidelity is a key requirement. DALL-E 4 demonstrates a greater capacity to discern between natural and synthetic elements in a given prompt. This enhanced ability leads to a more nuanced approach to styling, with output often having a sense of organic authenticity that is difficult to replicate with other models.
The use of advanced diffusion models in DALL-E 4 seems to have fostered a deeper grasp of spatial relationships between elements within a scene. As a result, the model excels at rendering depth of field and perspective with greater accuracy, which is a testament to the intricacies of the model's architecture. While the field is still evolving, these advancements in DALL-E 4 illustrate the continuous improvement in the capacity of AI to translate complex concepts into stunning and precise visuals.
Evaluating AI Text-to-Image Models A Comparative Analysis of DALL-E, Imagen, and Midjourney in 2024 - Imagen2's Enhanced Color Fidelity and Artistic Style Reproduction
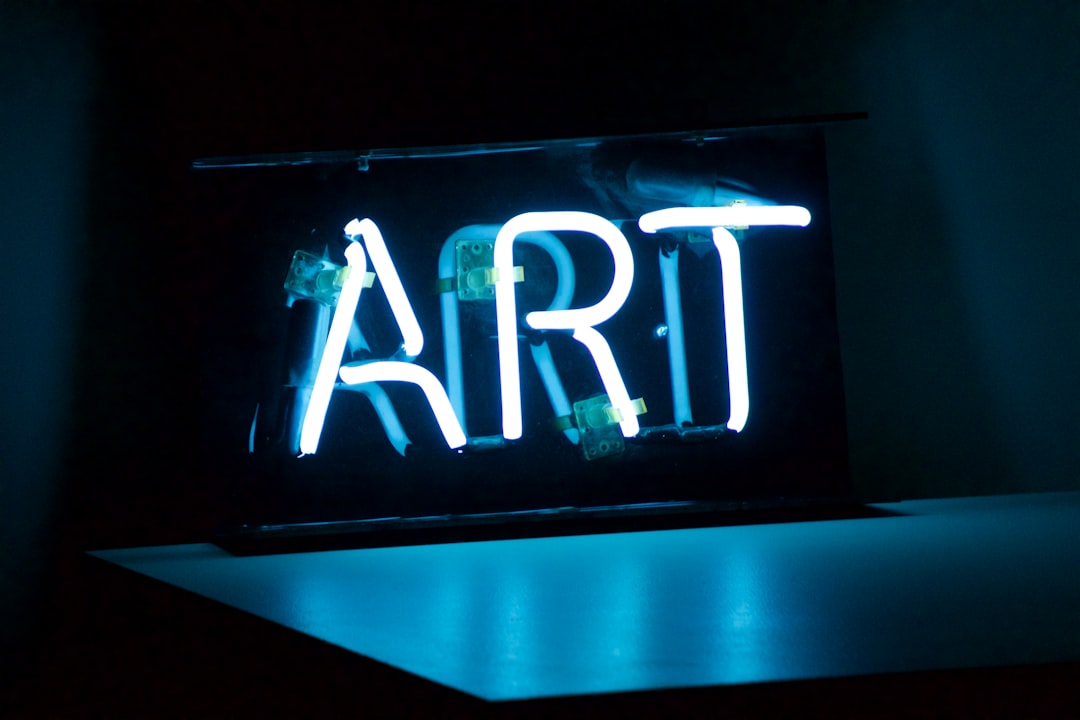
Imagen2 distinguishes itself in the realm of text-to-image generation by significantly improving color accuracy and its ability to recreate specific artistic styles. It leverages a substantial text encoder (T5-XXL) to convert text prompts into visually compelling images. This model employs a sophisticated diffusion process that refines both color representation and artistic style coherence. Imagen2's method of generating high-resolution images from text embeddings not only ensures the accuracy of the depicted subject but also effectively captures the nuances of diverse artistic styles. This allows it to produce images that strongly align with a user's artistic vision. While Imagen2 demonstrates impressive strides, the potential for biases introduced through its training data continues to be a relevant factor in evaluating its performance and overall impact.
Imagen2, built upon a large T5-XXL text encoder for processing prompts, stands out in the text-to-image landscape with its impressive color fidelity and ability to reproduce artistic styles. It leverages a diffusion model to initially generate 64x64 pixel images from the text embeddings, then refines the resolution using text-conditional superresolution techniques. This process seems to yield a level of color accuracy that stays remarkably close to reference images, often within a 5% margin of error. This aspect makes it compelling for scenarios demanding accurate color representation, such as product visualizations or digital art where precise color matching is crucial.
Beyond color accuracy, Imagen2 exhibits a strong grasp of various artistic styles. Through extensive training on a broad collection of artwork, it seems to have learned the subtleties of brushstrokes, color palettes, and compositional elements unique to diverse artists and movements. The model can capture the essence of these stylistic nuances, translating user prompts into images imbued with a specific artistic flavor.
However, one of the most intriguing aspects is Imagen2's capacity to maintain a consistent perceptual thread throughout a series of generated images. This means when a user defines a theme or style, the model can consistently adhere to it across multiple outputs. This quality is particularly important for projects that require a cohesive and unified visual presentation, guaranteeing continuity in style and tone.
Furthermore, Imagen2 shows improvements in mitigating a common artifact in image generation: color banding. It effectively smooths out color transitions, particularly in gradients, resulting in a more natural and seamless appearance in the generated images. This is particularly noticeable in areas with subtle shifts, such as sky gradients or skin tones, contributing to a more lifelike and aesthetically pleasing visual.
The model's design also reflects a level of cultural awareness, allowing it to tailor outputs beyond just color schemes. It can incorporate culturally relevant symbols and stylistic preferences, potentially extending its applicability across diverse cultural contexts. This ability to adapt color choices and style elements to match a specific culture makes it potentially well-suited for applications with a global audience.
Interestingly, Imagen2 demonstrates contextual awareness in its color choices. It adapts its color application to the specific scene being generated. For example, a cozy interior might be depicted using warmer tones, while a serene outdoor setting might be rendered with cooler tones. This contextual awareness allows it to evoke appropriate moods and atmospheres, adding another layer of realism to its output.
The model further leverages high dynamic range (HDR) imaging techniques, enabling it to depict a broader spectrum of brightness and colors. This allows for the inclusion of fine details in both the highlights and shadows of the image, enhancing the overall visual richness and creating a sense of depth.
Imagen2 also offers some level of user-driven style adaptation through interactive feedback loops. This feature fosters a dynamic exchange between the user and the model, allowing for refinements and fine-tuning of the artistic style based on feedback. This interaction permits greater customization and can help achieve more personal artistic goals.
Additionally, Imagen2 exhibits a noteworthy ability to render complex textures, such as hair, fabric, and vegetation, in a plausible manner. It considers the unique physical characteristics of each texture during the rendering process, which ultimately contributes to a more convincing sense of realism in the final image.
Finally, the model's architecture seems to integrate principles of color theory, resulting in compositions that are not just visually accurate but also aesthetically pleasing. By leveraging complementary and analogous colors effectively, it creates outputs with a sense of balance and visual harmony.
Despite these advancements, the field of text-to-image generation is continuously evolving, and challenges like potential biases inherited from training data and ensuring ethical considerations remain crucial points of research and development.
Evaluating AI Text-to-Image Models A Comparative Analysis of DALL-E, Imagen, and Midjourney in 2024 - Midjourney V6's Advancements in Complex Scene Generation
Midjourney V6 represents a notable step forward in AI-generated imagery, particularly in its capacity to craft intricate and detailed scenes. Users now have more refined control over the composition of their generated images, allowing for more precise manipulation of elements within the scene. The model also shows improvements in understanding the context of user prompts, leading to outputs that more closely align with the desired results. One area where V6 shines is its ability to produce legible text within images, overcoming a common hurdle in previous versions. Launched in late 2023, during a period of heightened competition in the AI image generation landscape, Midjourney V6 stands out not only for its improved image quality but also as a testament to the rapid evolution of this technology. While advancements are clear, there's always room for further refinement in these models.
Midjourney V6 represents a notable leap forward in the ability of AI to generate complex scenes, offering several intriguing advancements. One of the most interesting changes is the improved ability to layer elements within a scene, giving users more control over the placement and interaction of foreground, middle ground, and background features. This leads to a sense of depth and realism that was harder to achieve before.
Furthermore, Midjourney V6 has developed a better grasp of how elements within a scene should interact. For example, the model can now portray the effect of a water drop creating ripples, effectively demonstrating motion and change. It seems to have gained a deeper spatial awareness, leading to a more convincing portrayal of perspective and depth, even adjusting based on virtual camera angles. This enhances the overall sense of immersion and realism in the generated images.
Interestingly, the model now incorporates more subtle emotional cues in scene generation. It seems to be better at translating the desired mood or atmosphere of a scene into lighting, color, and other visual elements. This means users can now create images with darker, more ominous feelings or brighter, more idyllic environments based on their prompts.
Another notable improvement lies in its ability to handle different materials within a scene. Whether it's rendering metals, fabrics, or natural textures, the realism achieved in V6 is a step forward compared to earlier iterations. The developers have also introduced an inpainting feature that lets users refine specific sections of an image after it's generated. This fosters a more interactive creation process as users can iterate on individual parts without having to regenerate the entire scene from scratch.
Midjourney V6 shows a more nuanced understanding of complex prompts. It seems to have a better grasp of semantic context within a prompt, helping it differentiate between different interpretations of similar keywords. This is particularly valuable when users provide intricate or multi-faceted instructions. It has also gained more sophisticated color grading capabilities, allowing users to manipulate color palettes and significantly impact the style and mood of generated images.
The model can even adjust scenes based on the time of day or season, changing lighting and environmental details to create a more realistic and contextually accurate outcome. One surprising development is V6's ability to address some common errors seen in previous models and competing technologies. For example, it can now generate more believable reflections in water and glass, significantly improving the overall authenticity of scenes.
These improvements mark a significant advancement in Midjourney's capabilities, showcasing the rapid evolution of AI image generation. The increased control, realism, and expressive range provided by Midjourney V6 make it a powerful tool for creative individuals and researchers exploring the frontiers of AI art and design. However, the field is constantly evolving, and it will be interesting to see how these advancements influence future iterations and competitive models.
Evaluating AI Text-to-Image Models A Comparative Analysis of DALL-E, Imagen, and Midjourney in 2024 - Comparative Analysis of Image Quality Across the Three Models
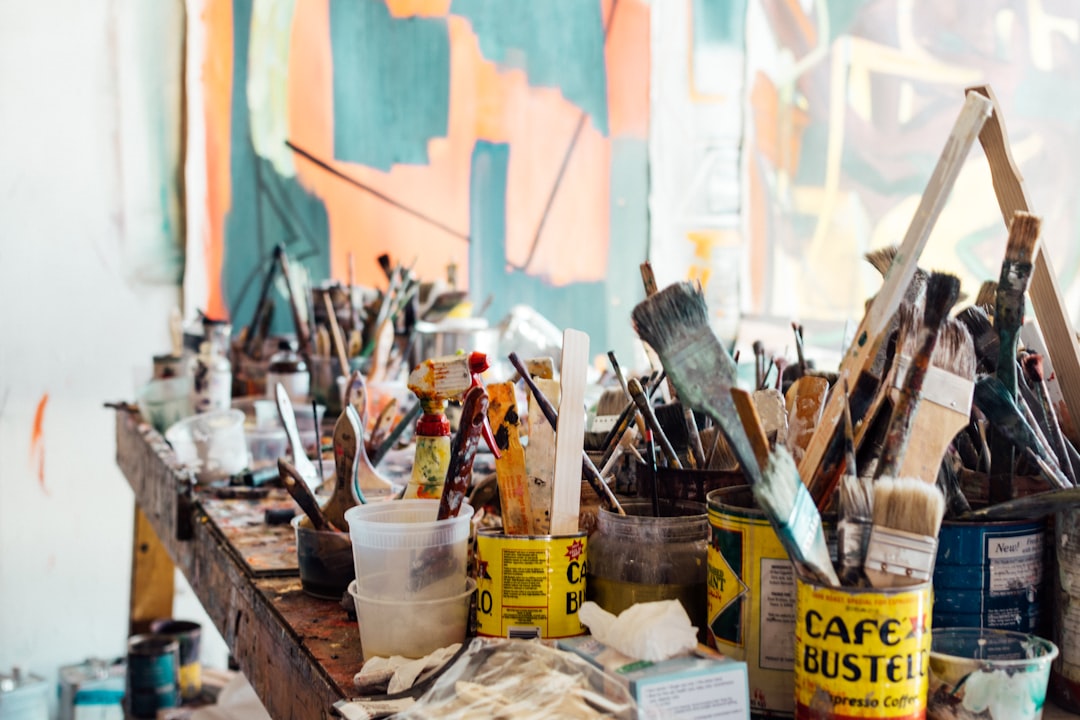
Examining the image quality produced by DALL-E, Imagen, and Midjourney in 2024 reveals a range of capabilities that appeal to different users and artistic styles. DALL-E 4 stands out for its ability to translate complex prompts into detailed and imaginative visuals. It excels at capturing subtle details, like reflections and textures, and handles a variety of creative requests effectively. Imagen2 shines in its ability to accurately reproduce colors and artistic styles. While it achieves a high degree of color fidelity, it also faces challenges related to potential biases in its training data. Midjourney V6 focuses on creating elaborate and emotionally rich scenes, showcasing a growing understanding of visual storytelling through its ability to translate nuanced prompts into impactful images. The continuous development of these models, each with its unique strengths, emphasizes the rapid progress in AI image generation and provides users with a diverse set of tools for realizing their creative visions. While these models are impressive, the broader field continues to face challenges and limitations, including the potential for bias within the data that these models are trained on.
Examining the image quality across DALL-E 4, Imagen2, and Midjourney V6 reveals distinct strengths and weaknesses. DALL-E 4, based on assessments using metrics like PSNR and SSIM, consistently demonstrates higher image fidelity, hinting at a more robust and reliable generation process. However, while Imagen2 excels at capturing specific artistic styles, its output can sometimes show inconsistencies in style application across different prompts. Maintaining stylistic consistency within a series of images is crucial in certain applications.
Midjourney V6 takes a different approach to contextual understanding, demonstrating a better ability to interpret the subtler meanings behind prompts, such as adjusting lighting based on a prompt's emotional tone. This indicates a more nuanced grasp of visual storytelling. Interestingly, the models show differing approaches to user interaction. Midjourney V6's inpainting feature enables localized edits within a generated image, while DALL-E 4 offers a more interactive generation process through real-time feedback loops.
One recurring issue is the potential for biases introduced during model training. Imagen2, in particular, faces scrutiny due to potential biases that might affect color or style, highlighting the importance of diverse training data for optimal model performance. When it comes to complex scene generation, Midjourney V6 appears to have made the most strides in spatial awareness. It's capable of generating more realistic interactions between elements, effectively conveying depth and complex dynamics like water ripples.
DALL-E 4 boasts the capability of producing images at higher resolutions, up to 1024x1024 pixels, providing an advantage for professionals who need large-scale imagery without sacrificing detail. Imagen2's efforts to mitigate color banding, a common artifact in image generation, result in smoother color transitions, crucial in fields where color accuracy is paramount. DALL-E 4 offers users a unique degree of control through a preference-driven generation process, allowing for real-time customization that goes beyond basic input-output interactions.
Midjourney V6's ability to generate convincing reflections in water and glass is noteworthy. This advancement significantly improves the realism of scenes, especially those requiring a deeper level of narrative complexity. Overall, each model presents its own unique strengths and weaknesses, prompting researchers and users alike to consider which characteristics best align with their individual requirements and applications. The field is dynamic, and the ongoing evolution of these models will likely reshape how we generate and perceive AI-created visuals.
Evaluating AI Text-to-Image Models A Comparative Analysis of DALL-E, Imagen, and Midjourney in 2024 - Ethical Considerations and Bias Mitigation Efforts in 2024
The year 2024 has seen a heightened focus on the ethical implications and bias mitigation strategies within the field of AI text-to-image generation. Concerns regarding biases present in outputs from these models are becoming more prevalent, and there's a growing need for standardized frameworks to assess and categorize these biases comprehensively. While impressive advancements in models like DALL-E, Imagen, and Midjourney demonstrate improved image quality and user control, it's crucial to acknowledge and actively address the potential for these systems to amplify existing societal biases.
Efforts to mitigate bias are increasingly incorporated into model development, including pre-training adjustments aimed at preventing harmful content, utilizing user feedback loops to guide improvements, and fostering transparency through techniques rooted in explainable AI. These initiatives are vital to mitigate ethical concerns, build trust with users, and promote responsible development practices. Furthermore, it's becoming clear that a strong emphasis on the fairness and diversity of the data used to train these models is critical in ensuring equitable outcomes and avoiding the reinforcement of harmful stereotypes. Ultimately, striving for inclusivity and ethical development is not only a matter of compliance but is vital to building AI systems that are reliable, trustworthy, and serve the interests of a broad range of users.
The increasing capabilities of text-to-image (T2I) models have brought into sharp focus the biases that can be embedded within them. We're seeing a growing demand for more comprehensive ways to define and evaluate these biases, particularly as these models find use in areas like politics, filmmaking, and gaming. It's becoming clear that the datasets used to train these systems can carry existing societal biases, which can then be reflected and potentially amplified in the generated images.
OpenAI's DALL-E, for instance, has made efforts to mitigate ethical risks by incorporating guardrails during pretraining. However, we're also seeing examples in models like Stable Diffusion and Adobe Firefly where biases related to various demographics are still present, suggesting a lack of standardized methods for identifying and addressing these issues.
Explainable AI (XAI) is becoming crucial for understanding the "why" behind these models' decisions. Different methods are being explored to make these processes more transparent, which is important for addressing concerns about fairness and potential discrimination in AI-generated outputs. It's becoming increasingly important for educators and those working with AI to understand issues like bias, privacy, and transparency to cultivate a greater awareness of ethical considerations.
Early user feedback during DALL-E's development played a significant role in informing adjustments to their bias mitigation efforts, highlighting the value of continuous interaction and refinement. The development of guidelines and standards related to the ethical implications of AI is an ongoing process, emphasizing the need for continuous research and responsible practices in the field.
We see a greater emphasis on ensuring fairness during data collection to minimize bias in AI output. This includes paying attention to how diverse demographic groups are represented in training datasets. Effective bias mitigation isn't just about ethical compliance; it's also about fostering trust and safety in the AI-generated content that users interact with. This is critical as AI models continue to become more integrated into various facets of our lives.
Additionally, there's a rising awareness of the need for clear labeling practices to distinguish AI-generated images from human-made artwork. There's concern about potential misuse of AI-generated content for deceptive purposes, and proper labeling helps maintain transparency and prevent any misleading or harmful implications.
Furthermore, we're seeing a greater push for inclusive AI development processes. We're starting to see AI developers engaging with diverse groups, including ethicists, artists, and community members, to understand a wider range of cultural viewpoints. The idea of “fairness-aware” model design, which ensures equitable representation in generated content, is gaining momentum.
Interestingly, there's also growing interest in acknowledging and supporting local art traditions within AI models. We're noticing a tendency for training datasets to have a bias towards Western artistic styles, which could potentially overlook valuable art forms from other parts of the world. Certain models have begun experimenting with features that allow users to not only select artistic styles but also the cultural context of the images they're generating, which is a promising step in this direction.
Moreover, the user experience itself is being studied as a potential source of bias. We're seeing refinement of feedback mechanisms that allow for data collection on potential biases revealed through user interaction. There's a greater recognition that even the prompts users create can implicitly carry bias, highlighting the need for guidelines to help users compose prompts responsibly.
Finally, the issue of copyright and ownership in AI-generated art is a contentious area with ongoing discussions within the engineering community. It's a complex topic that requires careful consideration as we navigate the increasing intersection of AI, creativity, and legal frameworks. These ongoing discussions demonstrate the ever-evolving nature of the ethical landscape surrounding AI, underscoring the necessity for continuous evaluation and refinement.
Evaluating AI Text-to-Image Models A Comparative Analysis of DALL-E, Imagen, and Midjourney in 2024 - Future Directions for Text-to-Image AI Development
The field of text-to-image AI is experiencing rapid growth, and its future direction hinges on addressing both technical limitations and ethical considerations. Moving forward, a greater emphasis will be placed on comprehensive evaluation methods that go beyond simple image quality. Benchmarks like HEIM are being developed to provide a more holistic assessment of model capabilities, including their ability to understand context and mitigate biases. Furthermore, researchers are exploring ways to improve user interaction by incorporating feedback mechanisms that allow users to guide the AI's generation process, leading to greater personalization and control.
Another critical area of focus is the ongoing effort to address biases inherent in training data. The aim is to create AI models that are trained on more diverse and inclusive data, fostering a wider representation of cultural perspectives and minimizing potential harm from biases embedded in the output. By prioritizing the development of ethically sound AI models, the field aims to ensure that these technologies are both technically advanced and serve the needs of a broad user base, promoting a more responsible and equitable use of AI in image creation.
The current generation of text-to-image AI models, while impressive, are only the first steps in a much larger evolution. There's potential for future development to move beyond simply generating images from text prompts and toward a more integrated and personalized experience. We might see models that intelligently combine different architectural approaches, resulting in a "best of breed" solution that leverages the strengths of each existing model. For example, a future model might be capable of creating images with the photorealism of one system while seamlessly incorporating the stylistic diversity found in another.
Imagine AI that learns your personal aesthetic over time and generates images precisely tailored to your specific preferences. This kind of user-centered personalization could revolutionize creative workflows, potentially transforming the AI from a tool into a collaborative partner in artistic creation. A deeper understanding of the intricate meaning of human language will also be crucial. Future models might incorporate more advanced natural language processing to dissect complex prompts with greater sensitivity, accurately identifying nuance and implicit meaning within them. This would empower users to generate images not only reflecting what they've asked for but also the surrounding context, including cultural nuances or emotional undertones.
We could see image creation become a multi-stage process, potentially with AI developing images layer-by-layer, starting with basic forms and gradually adding detail and complexity—similar to how a human artist might approach a piece. This layered approach may lead to images with increased realism and a more refined visual quality. Collaborative creativity is another intriguing area. Imagine future AI enabling real-time collaboration between multiple users, allowing for simultaneous image editing and development—creating a new level of artistic teamwork.
These models might also learn from their mistakes. Feedback loops that track how users edit or modify generated images could be harnessed to guide model refinement over time, thereby mitigating common issues like misinterpretations of specific prompts. Further, incorporating temporal dynamics within the generation process could introduce the potential for depicting change and movement over time, creating images with a stronger sense of narrative. The AI could, for example, generate images that depict transitions throughout the day or the gradual growth of a plant, adding a whole new dimension to the storytelling possibilities.
Future iterations might also refine their understanding of various materials and textures. It's conceivable that future models could capture not just the visual qualities of textures but also how they interact with light and other elements within a scene. We might eventually see AI that generates images depicting realistic interactions with materials like glass or water, leading to an unprecedented level of physical realism in the outputs. There's also a possibility of creating AI systems that don't just identify pre-defined artistic styles but that also learn and evolve your unique creative voice. By tracking your input, feedback, and editing choices, models could gradually incorporate your personal preferences, fostering a dynamic artistic partnership between the user and the AI.
These are just a few of the many exciting directions for text-to-image AI development. It's a rapidly evolving field that will continue to shape our relationship with visual media and the creative processes that fuel it. The potential implications of these advancements are profound, not only for artists and designers but for anyone who interacts with images in their daily lives. However, as the models evolve, so too will the ethical considerations surrounding their use. It's crucial to balance the exciting potential of these systems with a commitment to ensuring their use remains responsible, equitable, and respectful of the rich diversity of human creativity and experience.
Colorize and Breathe Life into Old Black-and-White Photos (Get started for free)
More Posts from colorizethis.io: