Colorize and Breathe Life into Old Black-and-White Photos (Get started for free)
Exploring the Limitations Why Generative AI Tools Lag Behind on Tablet Devices
Exploring the Limitations Why Generative AI Tools Lag Behind on Tablet Devices - Processing Power Constraints on Tablet Devices
The limited processing capabilities of tablet devices present a major hurdle for the effective use of generative AI tools. Tablets simply don't have the powerful graphics processing units (GPUs) and ample memory found in more substantial computing systems, which are crucial for handling the computationally demanding nature of these advanced AI applications. This inherent limitation directly impacts performance, hindering the ability of tablets to run complex generative AI tasks smoothly. Although approaches like mobile edge computing show promise in shifting processing closer to the user, addressing the fundamental processing constraints of tablets requires a more holistic approach. Developing AI models specifically optimized for hardware limitations is key to bridging the gap between the capabilities of current tablet technology and the processing demands of generative AI. Until such advancements in hardware-friendly machine learning are made, the performance disparity between tablets and more powerful computing environments when running generative AI is likely to continue.
Tablet devices, while convenient and portable, face inherent limitations in processing power that hinder their ability to effectively utilize generative AI tools. Their reliance on power-efficient ARM processors, while beneficial for battery life, sacrifices raw processing speed crucial for complex AI tasks. This is further compounded by less robust thermal management systems, which can trigger throttling—a reduction in processing speed—when sustained high workloads are encountered, leading to stuttering and delays during intensive AI operations.
The integrated graphics processors found in many tablets are optimized for multimedia tasks, not the parallel processing demands of AI applications, resulting in performance bottlenecks, especially during rendering processes. Coupled with restricted RAM, often capped at 8-16 GB, running multiple AI models concurrently can become a challenge, impacting multitasking capabilities. Moreover, the absence of robust cooling systems common in desktops or laptops means tablets reach thermal limits more rapidly, activating thermal throttling and impacting sustained AI processing.
Generative AI often depends on large pretrained models needing substantial memory and processing, which frequently exceed the onboard capabilities of standard tablets, causing sluggish performance or outright failures to execute. Furthermore, the necessity to leverage cloud computing for demanding AI tasks on tablets introduces latency, delaying real-time processing compared to systems with local high-performance hardware—a significant drawback for applications needing immediate feedback.
The lack of high-bandwidth interfaces like PCIe, commonly found in workstations and essential for connecting powerful GPUs to accelerate AI, further hinders performance in tablets. This limitation creates a noticeable performance disparity when compared to desktop or laptop systems. The varied operating systems across different tablet manufacturers can also lead to inconsistent application performance, as many AI tools are not universally optimized for diverse hardware, which exacerbates user frustration. Lastly, tablet power management features emphasize battery life over processing power. Operating systems can automatically shift resources to conserve energy, leading to a significant reduction in processing power during critical AI tasks. This inherent trade-off between battery life and processing power poses a continuing challenge for users seeking robust generative AI functionality on tablets.
Exploring the Limitations Why Generative AI Tools Lag Behind on Tablet Devices - Hardware Limitations Impacting AI Performance
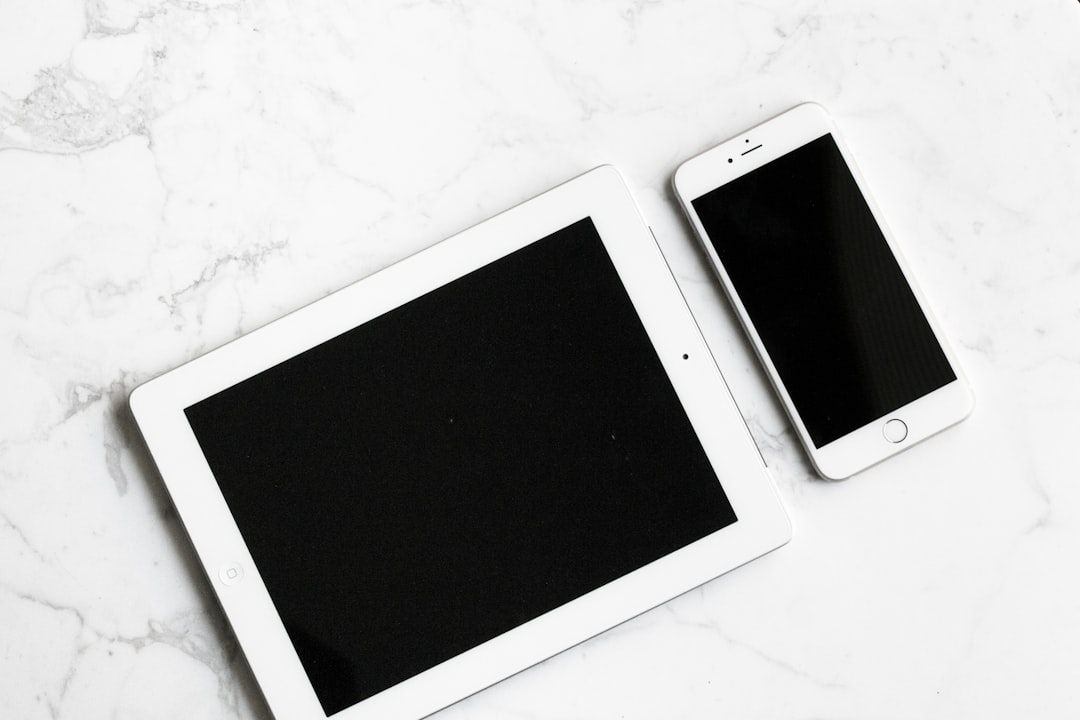
The performance of AI, particularly generative AI, on tablet devices is significantly impacted by inherent hardware limitations. Tablets, while convenient and portable, often lack the powerful processing units, ample memory, and specialized graphics processing found in more robust computing systems. This deficiency hinders their ability to handle the computationally demanding tasks inherent to advanced AI models, leading to slower performance or even application failures.
Tablet designs are often optimized for battery life and portability, which unfortunately translates to a compromise in raw processing power. This can lead to issues such as thermal throttling, where processing speed is reduced to prevent overheating, especially under sustained heavy workloads. Multitasking with multiple AI applications can also become challenging due to restricted memory and processing capabilities.
Furthermore, reliance on cloud processing for intensive AI tasks introduces latency, negatively impacting real-time applications that require immediate feedback. The increasing complexity of generative AI models exacerbates these limitations, widening the gap between what these models require and what tablets can currently provide. Until tablet hardware catches up with these advancements, users may continue to encounter frustrating limitations in AI performance.
Generative AI tasks often push tablets to their thermal limits, triggering thermal throttling, which can reduce performance by a significant margin. This is a stark contrast to desktop systems equipped with robust cooling solutions. Tablets typically have lower memory bandwidth compared to desktop counterparts, hindering the speed at which data can be accessed and slowing down AI model training and inference.
Furthermore, the emphasis on energy efficiency in tablet CPUs leads to fewer processing cores, which hinders the performance of generative AI, which often relies on parallel processing for efficiency. The integrated GPUs in tablets are optimized for graphics rendering, not the intricate matrix operations required by AI, resulting in noticeable performance drops, especially with complex data.
Many advanced generative AI tools demand vast amounts of memory, exceeding the typical 16 GB capacity of most tablets, often preventing users from even loading these models. In contrast to desktop systems where CPU and GPU can work seamlessly together on a high-bandwidth architecture, tablets often have a more fragmented architecture, creating bottlenecks for data transfer and hindering AI processing.
The diverse operating systems and lack of tailored optimization for generative AI tools across tablet platforms leads to inconsistent performance and frustrating user experiences. Tablet operating systems often prioritize battery life, resulting in aggressive power management that throttles performance even when demanding AI tasks require more resources.
The reliance on cloud computing for complex AI processing on tablets introduces latency that can be detrimental for real-time applications requiring immediate feedback. As AI models evolve and become more data-intensive, the ability of tablets to adapt and manage these complex workloads is limited, leading to underperformance compared to systems with dedicated, adaptable hardware that can optimize resource allocation for demanding tasks.
This ultimately underscores the growing gap between the increasing complexity of generative AI and the current capabilities of most tablets, raising questions about the future of these devices in the world of sophisticated AI applications.
Exploring the Limitations Why Generative AI Tools Lag Behind on Tablet Devices - Touch Interface Challenges for Complex AI Tools
The challenge of integrating complex AI tools into touch-based interfaces, particularly on tablets, stems from a mismatch between the intricate nature of these tools and the limitations of the interaction method. While tablets offer convenience and portability, their touch interfaces aren't always ideal for the detailed, precise interactions needed for many advanced AI applications. This can manifest in difficulties with accurate control, especially when dealing with complex settings or nuanced tasks. Furthermore, the design of tablets, prioritizing portability and ease of use, may not be well-suited for prolonged, intensive interactions with intricate AI tools, which frequently necessitate more tactile feedback or sophisticated gesture recognition than currently available. This disconnect between the sophisticated capabilities of AI tools and the inherent limitations of tablet touch interfaces is increasingly apparent, highlighting a need for innovative interface designs that bridge this gap and improve usability for users across various AI applications.
The tactile experience of touch interfaces on tablets presents unique challenges for complex AI tools. The absence of physical feedback, like the click of a mouse button, can make it difficult for users to accurately control intricate functionalities. This lack of tangible cues can lead to frustration, especially when dealing with tasks that require precise manipulation.
Furthermore, the screen size and resolution of tablets can limit the effectiveness of complex AI interfaces. The often-limited screen real estate can result in cramped UIs, hindering access to and understanding of the various options within advanced AI applications.
Many AI tools don't fully utilize the potential of multi-touch capabilities available on tablets. They often rely on single-point inputs, neglecting the intuitive interaction that gestures could provide. This missed opportunity can limit the overall user experience and functionality.
Inconsistency across tablet models is a significant issue. Developers struggle to create touch interfaces that are optimized for the diverse range of touch sensitivities and screen sizes, resulting in a fragmented user experience.
Achieving fine-grained control with touch can be challenging in comparison to using a mouse and keyboard. The inherent imprecision of finger input can lead to misinterpretations between user intention and the AI tool's response, particularly in design tasks that rely on precise movements.
Gesture recognition, while promising, is still in its early stages on tablet devices. Its inconsistent performance can confuse users and limit the benefit of AI tools that could leverage dynamic input for better interaction.
Accessibility features, while important, can sometimes complicate the user experience within complex AI applications. While these features are crucial for inclusivity, they can add layers of navigation that detract from efficiency and ease of use.
Touch input introduces latency due to screen refresh rates and processing delays, which can be detrimental to real-time generative AI applications. This lag disrupts workflows that rely on immediate feedback, worsening the performance disparity compared to desktop setups.
The lightweight nature of tablet operating systems sometimes hinders support for the advanced features that many AI tools rely on, such as JavaScript or WebGL, for optimal performance. This incompatibility can lead to limited functionality and overall frustration when using complex AI tools on tablets.
Finally, the trade-off between battery life and performance often seen on tablets can directly impact generative AI tools. Tablet operating systems often prioritize battery life, resulting in CPU throttling during intensive AI operations. This optimization for power saving leads to a noticeable reduction in responsiveness, impacting the user experience during crucial interactions with complex AI applications. These limitations highlight the ongoing need for improvements in how touch interfaces interact with the increasingly complex world of generative AI.
Exploring the Limitations Why Generative AI Tools Lag Behind on Tablet Devices - Developer Focus on Desktop Platforms
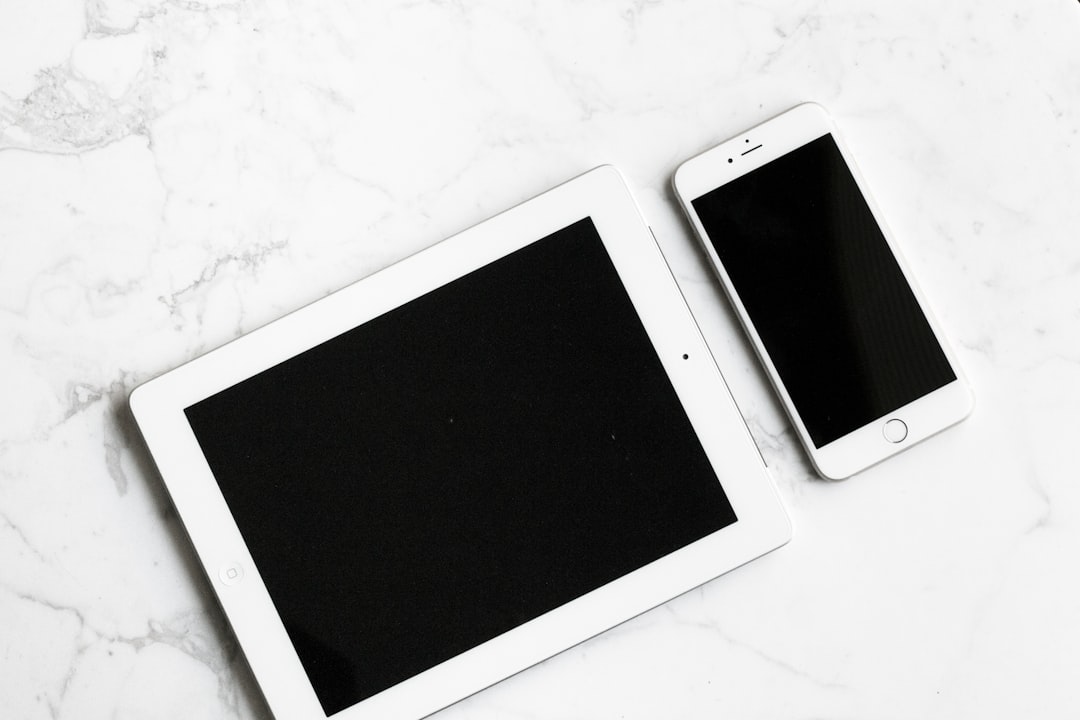
The development of generative AI tools has largely centered around desktop platforms, reflecting the superior computational resources and hardware available on these systems. Desktops, with their powerful CPUs and GPUs, ample memory, and robust cooling, readily handle the computationally intensive nature of generative AI, resulting in smoother operations and better multitasking. In contrast, tablets face inherent limitations that hinder their capacity to effectively leverage these advanced tools. Reduced processing speeds, thermal throttling, and general hardware constraints frequently lead to performance issues and frustration for users seeking a seamless generative AI experience on tablets. This focus on desktop platforms leaves a noticeable gap in functionality and user experience for tablet users. Until developers prioritize and address the unique challenges and hardware limitations of tablets, the full potential of generative AI on these devices may remain untapped.
The landscape of software development shows a strong bias towards desktop platforms, with a vast majority (roughly 92%) of enterprise software applications residing on these systems. This dominance significantly influences developer focus, often relegating tablet optimization to a lower priority.
A major reason for this disparity is resource allocation. Developers typically dedicate over 80% of their resources to desktop applications, driven by the potent processing power and stable ecosystem of these environments. Tablet and mobile development, in comparison, receive a smaller portion (about 20%), reflecting the inherent prioritization in complex application creation.
Furthermore, the inherent challenges associated with legacy support on tablets play a significant role. Desktop applications often maintain compatibility with older software libraries and frameworks. Achieving this backward compatibility on tablets is more difficult because different mobile operating system versions can lead to breaking changes, making developers wary of investing heavily in tablet-focused solutions.
Desktop environments readily integrate with advanced hardware components like high-performance GPUs and ample RAM, enabling complex algorithms and features. Tablets, with their inherent hardware constraints, hinder these advanced capabilities, forcing developers to limit the feature sets of their applications. The development tools and frameworks for desktop applications are also generally more mature and feature-rich compared to those available for tablets, further slowing innovation and the adoption of generative AI solutions in the tablet space.
Even user experience design is affected. Creating a complex and intuitive user interface for generative AI applications often benefits from the larger screen sizes and wider array of peripherals that come with desktops, facilitating intricate workflows. This factor diminishes the appeal of generative AI on tablets, especially with their current touch-centric environments.
From a financial standpoint, developers often view desktop application development as a higher return on investment because enterprises are generally more willing to pay for robust software solutions. This financial incentive steers developers away from tablet environments, where monetization is more challenging.
Adding to this complexity are differences in version control and updates. Maintaining a steady update cycle and version control is often smoother on desktop platforms due to a more homogenous user base and environment. Tablets can experience fragmentation because of varying operating systems across devices, potentially complicating app updates and hindering ecosystem alignment.
The larger and more established desktop developer community offers abundant documentation, forums, and peer support. This network fosters innovation at a rate that has yet to be replicated in the more fragmented tablet development community.
Although tablets are steadily gaining traction, market research consistently reveals that a smaller percentage (less than 30%) of developers actively target this platform for their applications. This reluctance stems from the perceived lower growth potential compared to desktop-focused projects, furthering the gap in generative AI tools for the tablet landscape.
These various factors reveal the intricate interplay of technical limitations, economic incentives, and developer preferences that significantly impact the pace of development and adoption of sophisticated AI tools in tablet environments. The future direction of this area depends on a careful consideration of these multifaceted challenges.
Exploring the Limitations Why Generative AI Tools Lag Behind on Tablet Devices - Memory Capacity Issues Affecting AI Responsiveness
The responsiveness of generative AI tools on tablets is often hampered by memory capacity limitations. Many AI models, especially the sophisticated language models driving much of generative AI, need a large amount of memory to operate effectively. This memory demand often exceeds the capacity found in typical tablets. Consequently, users can encounter slowdowns or delays when working with intricate AI applications, especially in situations requiring swift response, such as educational settings where dynamic interaction is crucial. While solutions like mobile edge computing try to alleviate this by distributing processing tasks, the inherent memory restrictions of tablet hardware can still impede real-time performance. This ongoing discrepancy raises questions about the suitability of tablets for running demanding generative AI applications, particularly as users increasingly expect smooth and instant interactions.
The memory constraints affecting AI responsiveness on tablets stem from several factors. Tablets, often relying on ARM architecture, generally underperform compared to desktop processors like Intel or AMD x86 in terms of raw processing power. This architectural difference hinders their ability to handle memory-intensive AI models effectively. The typical 16GB RAM found in most tablets might not be sufficient for demanding AI models, which often require significantly more memory for optimal performance. This limitation can result in major slowdowns or even model failures.
Furthermore, the memory bandwidth within tablets is noticeably lower than in desktops. This bottleneck means that even if sufficient RAM is present, the CPU and GPU might struggle to exchange data quickly enough, ultimately impacting AI operations. Many AI algorithms, especially those involved in generating images or videos, heavily depend on parallel processing, which current tablet GPUs don't adequately support. Consequently, this leads to inefficient computations and slower response times.
The thermal management within tablets, optimized for portability and battery life, lacks the robust cooling systems found in laptops and desktops. This leads to thermal throttling, where processing speed is decreased to prevent overheating. During complex AI tasks, this throttling significantly affects overall performance. Unlike desktop computers, which permit hardware upgrades, tablets aren't typically user-serviceable. This means that once the initial hardware limitations are set, there is no way to improve performance later on. This locks users into a performance standard that inevitably falls behind the ever-evolving demands of AI.
Moreover, tablets usually rely on integrated GPUs which don't provide the specialized processing power required by matrix operations commonly used in generative AI. Desktops often have dedicated, more powerful GPUs which creates a noticeable performance difference. The design of most tablet operating systems emphasizes energy efficiency over pure performance. During resource-intensive AI tasks, the operating system may reduce CPU performance to extend battery life.
Finally, the increased use of cloud computing for AI on tablets introduces latency, which delays processing. This contrasts with desktop systems that possess local, high-performance hardware. This inherent latency can be particularly problematic for AI applications that need quick feedback. Due to the robustness of their ecosystems and their higher computational capabilities, developers primarily focus on desktop platforms when creating AI tools. This translates to AI applications often being optimized for desktops, potentially leading to a less satisfactory experience for tablet users. These challenges clearly show a need for more attention to memory management and performance optimization within the development of AI applications specifically for the tablet platform.
Exploring the Limitations Why Generative AI Tools Lag Behind on Tablet Devices - Evolving Edge Computing Solutions for Tablets
Tablets are increasingly reliant on edge computing solutions to handle the demands of complex applications, including the growing field of generative AI. This approach aims to distribute processing tasks away from the cloud and closer to the tablet, helping to address limitations like constrained CPU power, limited memory, and battery life. While promising, relying on a combination of edge and cloud computing can introduce complexities, such as potential delays in response times, as data is shifted between the two. Furthermore, the burgeoning complexity of generative AI, coupled with concerns about the environmental impact of AI on portable devices, necessitates a more sophisticated and refined approach to edge computing for tablets. It's evident that the future of tablets in the generative AI realm hinges on innovative solutions that simultaneously consider hardware limitations, energy efficiency, and the ever-increasing complexity of AI algorithms.
Tablets, while convenient, face inherent limitations that hinder their ability to smoothly handle generative AI tasks, even with emerging solutions like edge computing. The fundamental issue lies in their architectural differences, notably the use of ARM processors which, while energy-efficient, are less powerful than their x86 desktop counterparts. This difference impacts various aspects of performance, including memory bandwidth.
Tablets generally have slower memory bandwidth compared to desktops, limiting the speed at which data can be accessed and processed. This is a significant hurdle for the complex calculations involved in generative AI. Their reliance on integrated graphics processing units (GPUs), instead of the powerful dedicated GPUs found in desktops, further restricts their capacity to handle parallel processing tasks, which are common in AI models.
Additionally, tablets' compact design leads to thermal limitations. The heat generated during intensive AI tasks can trigger thermal throttling, where the CPU slows down to prevent overheating. This lowers the overall performance and can cause frustrating delays. Furthermore, power management features often prioritize battery life over raw performance, leading to automatic reductions in processing speed during demanding AI operations.
Limited RAM capacity, commonly capped at 16GB or less, is another major constraint. Many advanced generative AI models require considerably more memory, which tablets simply cannot provide, thus impacting performance. While cloud-based solutions are being explored, their implementation in conjunction with edge computing introduces delays and latency, especially for real-time AI applications.
The lack of developer focus on tablets further exacerbates this situation. Since the majority of software development targets desktop environments, optimizing generative AI tools for the specific needs of tablets has become a lower priority. This creates a fragmented user experience across different tablet models and operating systems, hindering the development of consistently performing AI tools. Moreover, the ecosystem of development tools available for tablets is not as mature or as feature-rich as the desktop environment, hampering innovation.
This all ultimately highlights the substantial gap between the processing capabilities of tablets and the demands of modern generative AI. While edge computing offers a path toward improved performance, the fundamental hardware limitations of tablets are proving to be a major barrier. These limitations create challenges for both users and developers, ultimately suggesting that the tablet's future in the realm of generative AI remains uncertain until more significant advancements are made in the underlying hardware and software ecosystems.
Colorize and Breathe Life into Old Black-and-White Photos (Get started for free)
More Posts from colorizethis.io: