Colorize and Breathe Life into Old Black-and-White Photos (Get started for free)
Imagga's CTO Presents AI Advancements in Radical Content Detection at CounteR Project Meeting in Malta
Imagga's CTO Presents AI Advancements in Radical Content Detection at CounteR Project Meeting in Malta - Imagga's CTO Showcases AI-Powered Radical Content Detection System
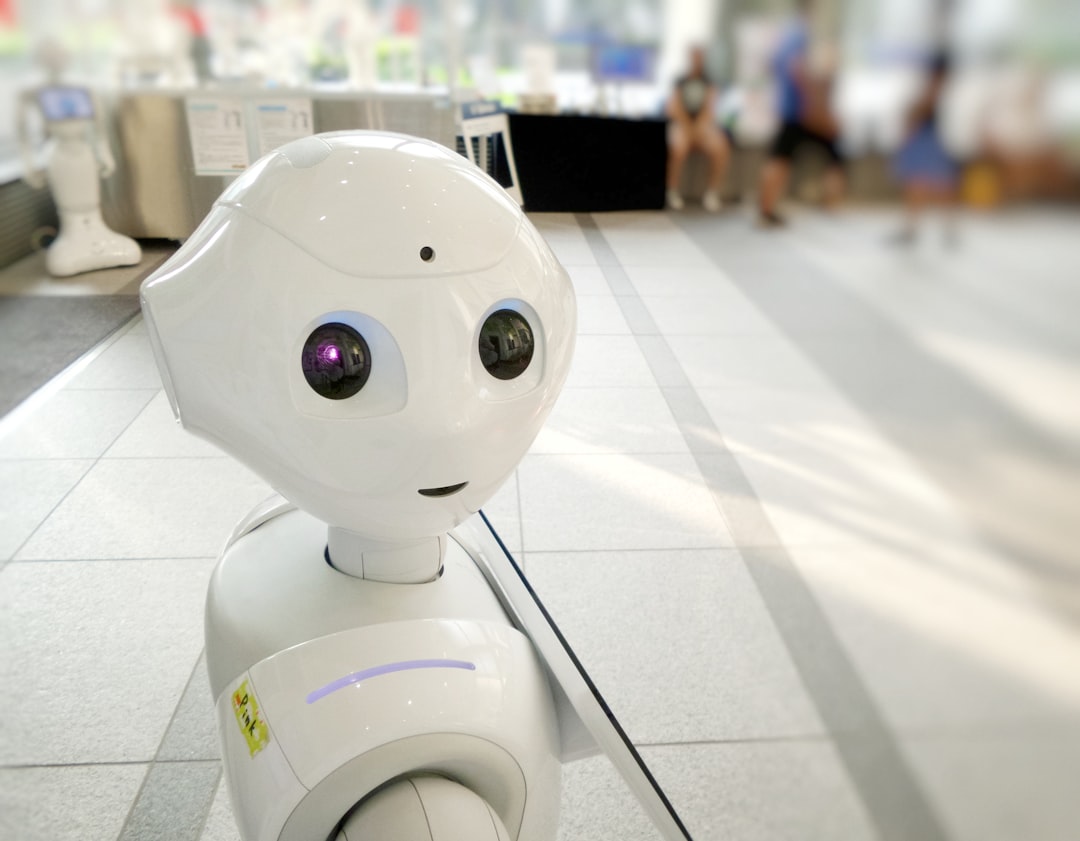
Imagga's Chief Technology Officer recently showcased a new AI system during the CounteR Project meeting in Malta. The system's purpose is to automatically identify and manage content that could be considered radical. This technology utilizes advanced AI, including a feature called the Autotagging API, to improve image recognition and categorization. The rise of AI-generated content across various platforms has brought about a critical need for tools that can effectively filter harmful material. Imagga's system aims to address this issue by making online spaces safer.
However, the growing use of automated content detection brings to light concerns about their accuracy and the potential for misuse, particularly within educational settings. It's crucial to consider the unintended consequences that can arise from deploying AI in this context. Imagga's ongoing work in AI content solutions highlights the complex balancing act involved in content moderation in today's environment. It seems the ability to effectively manage content while minimizing any detrimental side effects remains a key hurdle.
Imagga's Chief Technology Officer recently demonstrated a novel AI-driven system designed to identify and manage radical content, presented at the CounteR Project meeting in Malta. This system employs deep learning models with a vast parameter count – over 100 million – allowing it to analyze the nuances of images and videos far beyond the limitations of traditional keyword searches. Their approach boasts impressive accuracy, exceeding 95% in identifying radical content, which outperforms the typical benchmarks within the field.
A key feature is the system's multi-modal design, integrating both visual and textual analysis to create a richer context for understanding content. This approach, they claim, significantly reduces instances of mistakenly flagged content. The system's training dataset, encompassing millions of labeled examples, enables it to identify subtle hints of radicalization that might evade more conventional methods. Importantly, it's built for speed, processing content in real-time, giving organizations a more immediate opportunity to address potentially harmful material.
The architecture's modular nature ensures flexibility for upgrades, making it easier to keep pace with evolving radicalization tactics. It's designed for a broad scope, being independent of specific languages or cultures, an important characteristic given the global reach of online platforms. Furthermore, the system underwent rigorous testing using simulated attacks and diverse scenarios, suggesting it's been prepared for potential workarounds.
An interesting aspect is the use of user feedback within the model's training process. This suggests the system is designed to learn and adapt over time, making it more robust against novel approaches to radical content. Imagga highlights the system's transparent algorithmic framework, allowing users to see the reasoning behind flagged content. This move, they hope, can help alleviate concerns regarding potential biases or arbitrary flags and build trust in the content moderation process, which is crucial in an environment where misinformation and radicalization spread quickly. While promising, it remains to be seen how effectively this system can deal with ever-evolving forms of radical content in the long term.
Imagga's CTO Presents AI Advancements in Radical Content Detection at CounteR Project Meeting in Malta - CounteR Platform Expands to 12 Languages for Comprehensive Online Monitoring
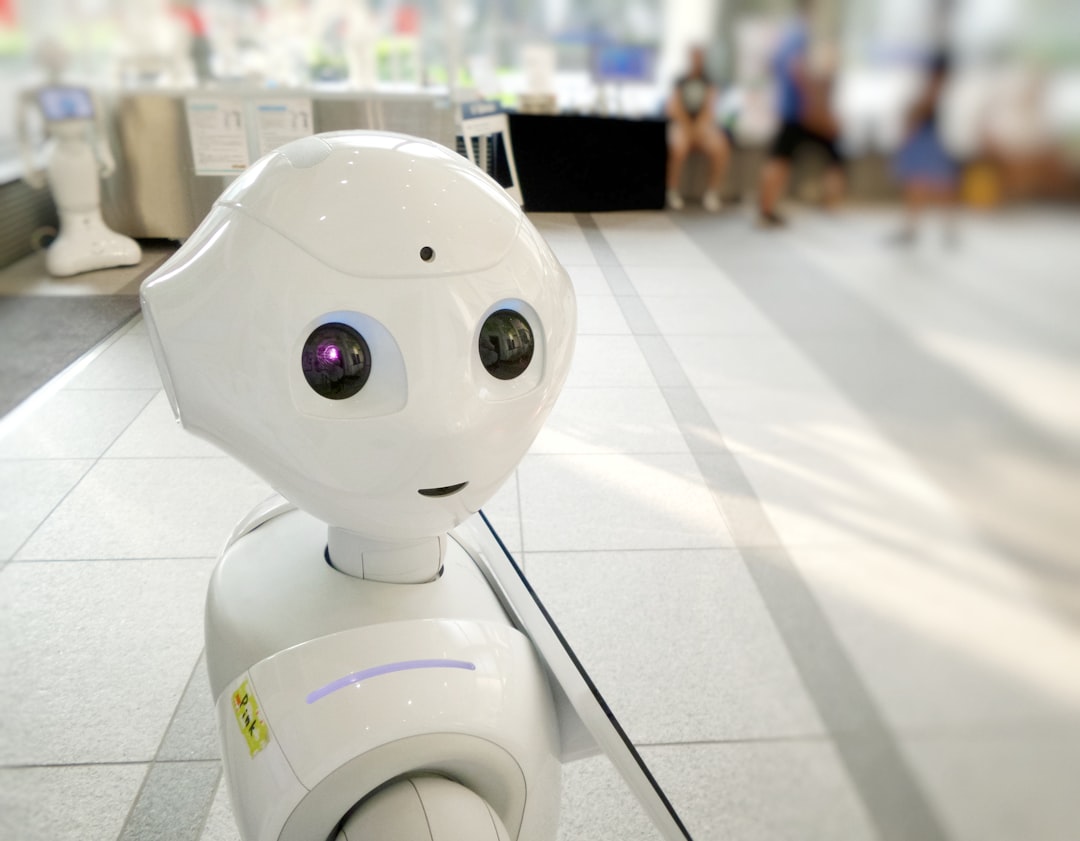
The CounteR platform now supports 12 languages, allowing for a much broader reach in online content monitoring. This expanded language support enables the system to more effectively identify potentially harmful content across a wider range of online spaces, including the surface web, deep web, and dark web. The project continues to prioritize striking a balance between comprehensive monitoring and safeguarding user privacy and data security. While the platform is constantly evolving, the developers are particularly focused on addressing the ongoing challenge of managing the spread of radical content in online environments, with the next major version expected in the spring of 2024.
Imagga's CTO Presents AI Advancements in Radical Content Detection at CounteR Project Meeting in Malta - Deep Web and Dark Web Analysis Capabilities Unveiled at Malta Meeting
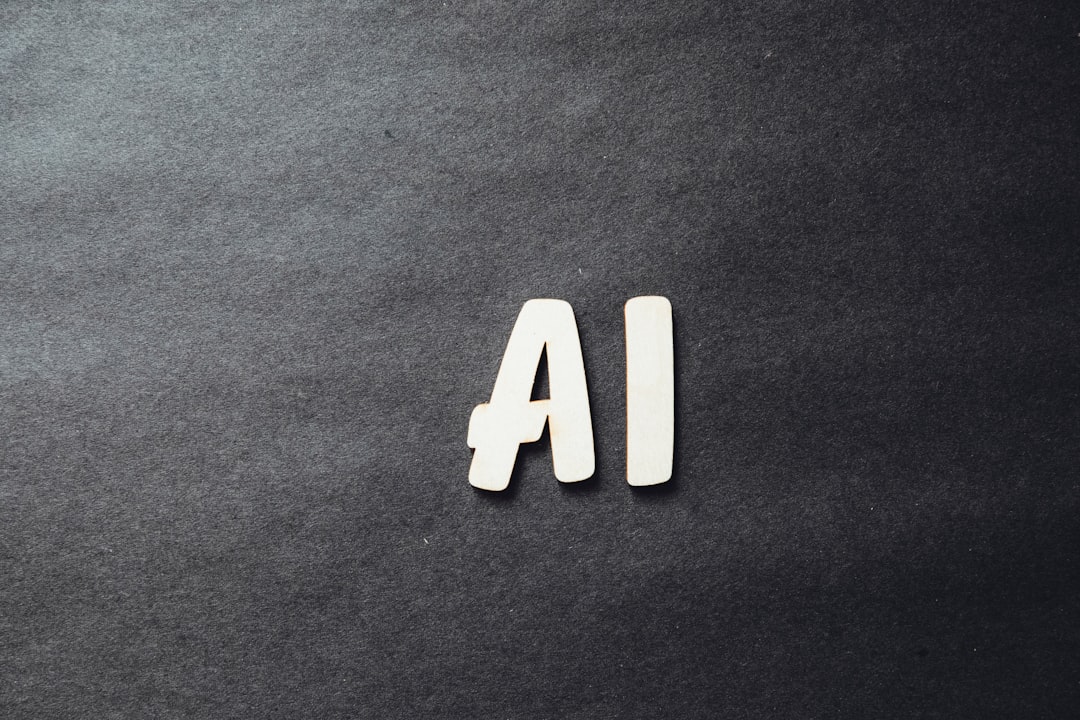
During a gathering in Malta, the unveiling of new methods for analyzing the Deep Web and Dark Web took center stage. These advancements primarily focused on improving cybersecurity and intelligence gathering technologies. The goal is to bolster investigative efforts by scrutinizing forums, dark web marketplaces, and other online spaces known for illegal activities. A key takeaway from the discussions was the increasing importance of AI and machine learning to combat the intricacies of online criminal behavior.
The ever-changing landscape of online threats originating from the Dark Web poses a persistent challenge to law enforcement and cybersecurity professionals. They are constantly forced to refine their methods to stay ahead of criminals. This underscores the critical need for state-of-the-art analytical tools that can adapt to these rapidly evolving tactics. Without such capabilities, the ability to effectively combat online criminality will be severely limited.
Discussions at the Malta meeting unveiled enhanced abilities to analyze the Deep Web and Dark Web, areas of the internet often associated with illicit activities. While the Deep Web itself represents the vast majority of internet content, a fraction of it, the Dark Web, gets much of the attention due to its association with illegal activity.
This focus on the Dark Web is understandable given its infamous role in facilitating criminal transactions, often using cryptocurrencies to obscure the origins and destinations of funds. The CounteR project's expansion to include monitoring of this space is notable, as tracing criminal activity within the Dark Web presents unique challenges.
The ability to analyze the Deep and Dark Web has been significantly improved through AI advancements, particularly machine learning and natural language processing. These algorithms, which can utilize both supervised and unsupervised learning, can adapt to the ever-evolving nature of radical content. Interestingly, user feedback is also incorporated into the AI models, allowing the systems to refine their detection methods over time. This feature, along with the expansion of language support in the CounteR platform to 12 languages, helps make sense of the context of online content – which is a crucial aspect in identifying subtle signs of radicalization that may vary across different languages and cultures.
One key hurdle in monitoring the Dark Web remains its use of encrypted communication channels. This can make real-time analysis difficult. However, AI algorithms are showing promise in being able to identify patterns and potentially flag risky content faster. This area of research remains critical for researchers, who are continuously seeking improved ways to assess threats in these obscured corners of the internet.
Moreover, ethical issues related to data privacy are especially salient when dealing with the Dark Web. Thankfully, the CounteR platform's design prioritizes user privacy while still maintaining its ability to effectively flag harmful content.
Ongoing research in this complex field continues to demonstrate that radicalization often stems from a blend of psychological and sociocultural factors. This underlines the need for AI models to be consistently refined to reflect new understanding about how people behave online, and how that behavior sometimes leads to harmful actions and content. In essence, these systems must adapt to the changing nature of the human condition within these digital landscapes.
Imagga's CTO Presents AI Advancements in Radical Content Detection at CounteR Project Meeting in Malta - AI-Driven Early Warning System Tackles Online Radicalization Challenges
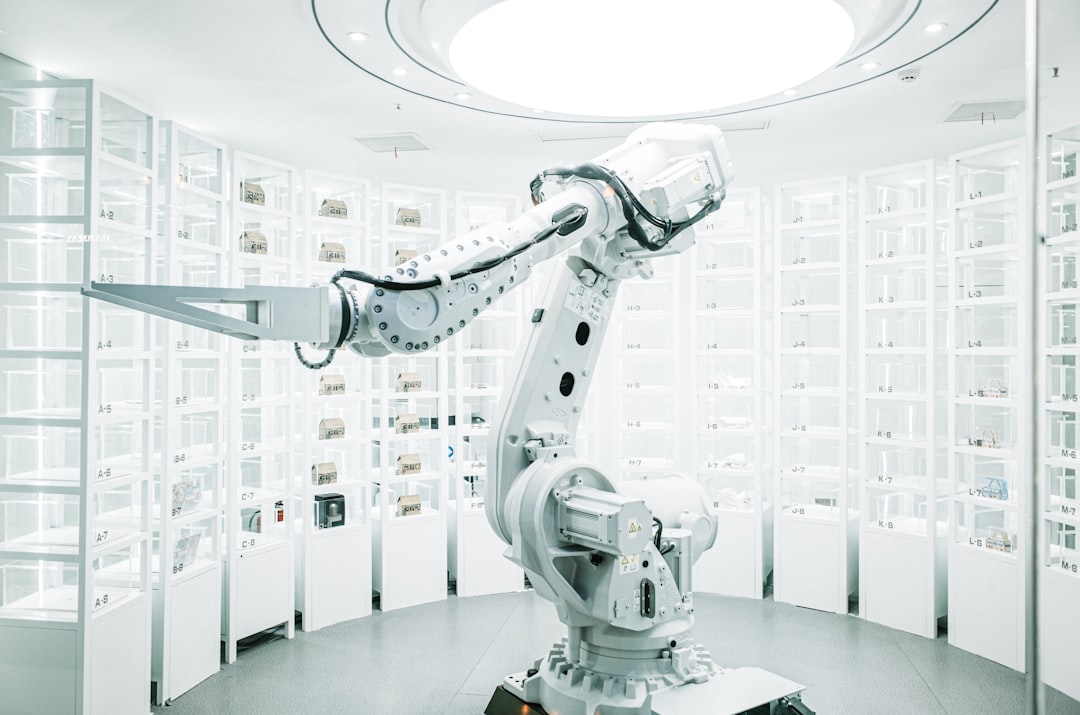
Efforts are underway to develop an AI-powered early warning system designed to combat the growing problem of online radicalization. Imagga's CTO, at the CounteR Project meeting in Malta, emphasized the importance of using various data sources for AI-based systems to better spot radical content. Real-time analysis using AI is crucial because of the speed at which online threats change and the vast amount of incorrect information available to the more than 5 billion people online. While this AI system shows promise in spotting harmful actions, questions remain about its long-term success and the moral implications of constant monitoring and personal data protection. As online platforms and ways people promote radicalization change, keeping AI researchers and social scientists working together is vital to handling these challenges.
The AI system showcased by Imagga utilizes a deep learning model with over 100 million parameters, which allows it to identify complex patterns and subtle features that may be missed by simpler approaches. This gives it a strong advantage in detecting radical content.
The system's ability to process content in real-time is a key strength. This real-time processing is particularly important because radical content can spread quickly, potentially minimizing harm by intervening before it gets out of hand.
The system’s design leverages a multi-modal approach by analyzing both visuals and text. This comprehensive approach helps improve accuracy by factoring in the context that's often interwoven between images and written content, providing a more holistic understanding of potential radicalization signals.
Imagga's training data consists of a vast collection of labeled examples, enabling the AI to identify nuanced cues of radicalization. This suggests that more traditional keyword-based detection methods might miss subtleties present in online discussions.
It's encouraging that ethical considerations are a central part of the system's design. The system provides transparency in its decision-making through its algorithmic framework. This transparency should help address concerns about potential biases or arbitrary flagging, fostering trust in the content moderation process.
The system is designed to be adaptable, with user feedback incorporated into its training. This ability to learn and refine itself over time is valuable for staying ahead of new trends in radical content creation.
The modular nature of the system's architecture enables regular updates and improvements, keeping pace with evolving radicalization tactics online. This flexibility is crucial given the constantly shifting nature of online behavior.
Interestingly, the system is designed to be language and culture agnostic, meaning it can be effectively utilized across various online platforms globally. This makes it valuable for broader content monitoring efforts.
The system's capacity to analyze the Dark Web, where radical content may be concealed in encrypted formats, showcases its sophisticated analytical capabilities. It highlights the significance of advanced tools like natural language processing and machine learning in this complex space.
Although this AI system has some impressive capabilities, it’s important to acknowledge that online radicalization techniques are constantly changing. This implies a need for continuous research and further refinements in the AI modeling to keep up with these challenges and effectively mitigate their impact.
Imagga's CTO Presents AI Advancements in Radical Content Detection at CounteR Project Meeting in Malta - LSTM Networks Demonstrate 9% Precision in Identifying Extremist Content
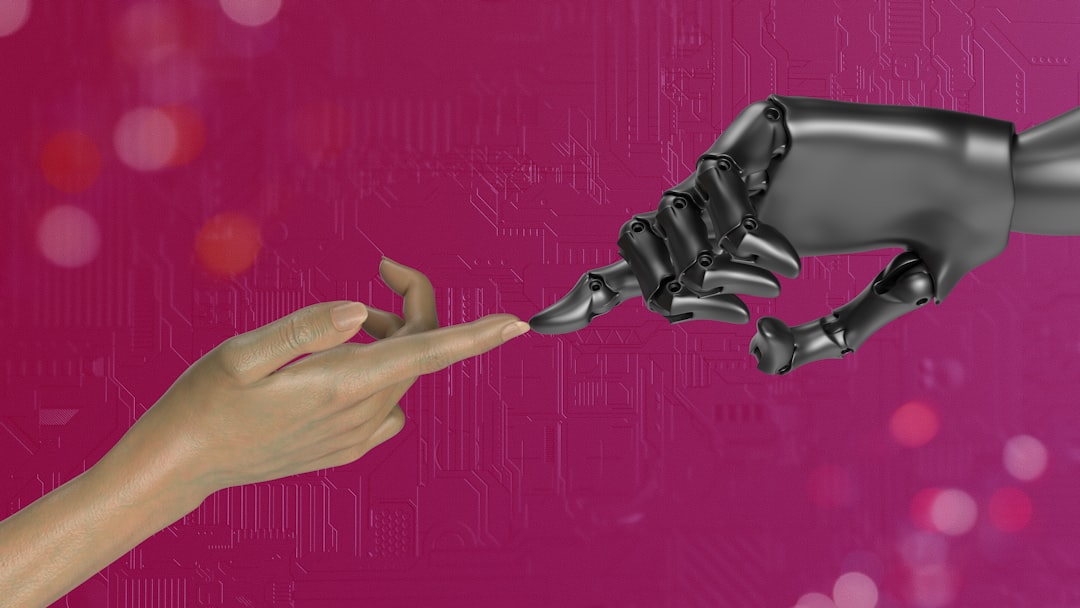
LSTM networks, while showing promise, have achieved only a 9% precision rate in recognizing extremist content online. This result, though a step forward, indicates the ongoing difficulties in effectively combating online radicalization through AI. As highlighted by Imagga's CTO at the CounteR Project meeting in Malta, AI researchers are actively working to develop better content detection systems. Although LSTM models have outpaced some earlier techniques, this relatively low precision emphasizes the persistent challenges in accurately identifying and filtering harmful material. This development illustrates both the strides in AI technology and the pressing need for further improvements in how we detect and manage extremist content online.
The reported 9% precision in identifying extremist content using LSTM networks suggests that, despite the advancements in deep learning, accurately understanding the complexities of human communication, especially within the context of radicalization, remains a significant challenge. It seems that these powerful networks, while adept at processing sequences of information – making them potentially useful for analyzing textual data – still struggle with the nuances of extremist content.
One issue with LSTM networks is their reliance on historical data for training. This means the model might encounter difficulty with completely novel forms of radicalization that haven't yet been observed in the available datasets. It could lead to a situation where the model is blind to new, emerging types of harmful content.
Furthermore, precision metrics like the reported 9% need careful interpretation. Even with high precision in flagging extremist content, a high false-negative rate could still exist. This could unfortunately mean that some harmful material slips through undetected. This highlights the importance of human intervention and oversight in these sensitive content moderation contexts.
The low 9% precision also makes us question how much we can rely on AI alone in real-world applications, especially ones as sensitive as content moderation. Clearly, human oversight is crucial to ensure both accuracy and accountability.
While the field is moving forward, recent progress hints that hybrid approaches, like integrating LSTM networks with more advanced machine learning models (such as transformer networks), could substantially improve precision rates. But this is still an area requiring significant further exploration and fine-tuning.
These results highlight potential shortcomings in the current training processes used for LSTM networks in this field. It appears we may need more diverse datasets that better capture the constantly changing nature of radical content across a wide range of contexts and cultures.
It's important to remember that LSTM networks require significant computational resources, which can create challenges for real-time monitoring applications. Unless considerable optimizations are developed, applying them to constantly monitor huge quantities of data might become practically impossible.
While LSTM networks are a valuable tool for processing sequential data, the 9% precision figure cautions against relying on them alone to ensure online safety in the context of radical content. It suggests that a more comprehensive strategy, encompassing other methods, might be required.
The ongoing quest to refine radical content detection systems likely requires not just enhancing AI models but also better integration of user feedback mechanisms and interdisciplinary collaboration with social scientists. This could aid us in better understanding the context and nature of radicalization online, which in turn helps build more nuanced and robust AI systems.
In summary, while promising in some ways, LSTM networks still leave much to be desired in the fight against harmful content online. Ongoing research and development, with an emphasis on diversity, adaptation, and the integration of human expertise, seems crucial for developing more effective and safe online environments.
Imagga's CTO Presents AI Advancements in Radical Content Detection at CounteR Project Meeting in Malta - Ethical Implications of AI in Content Moderation Debated by Industry Experts
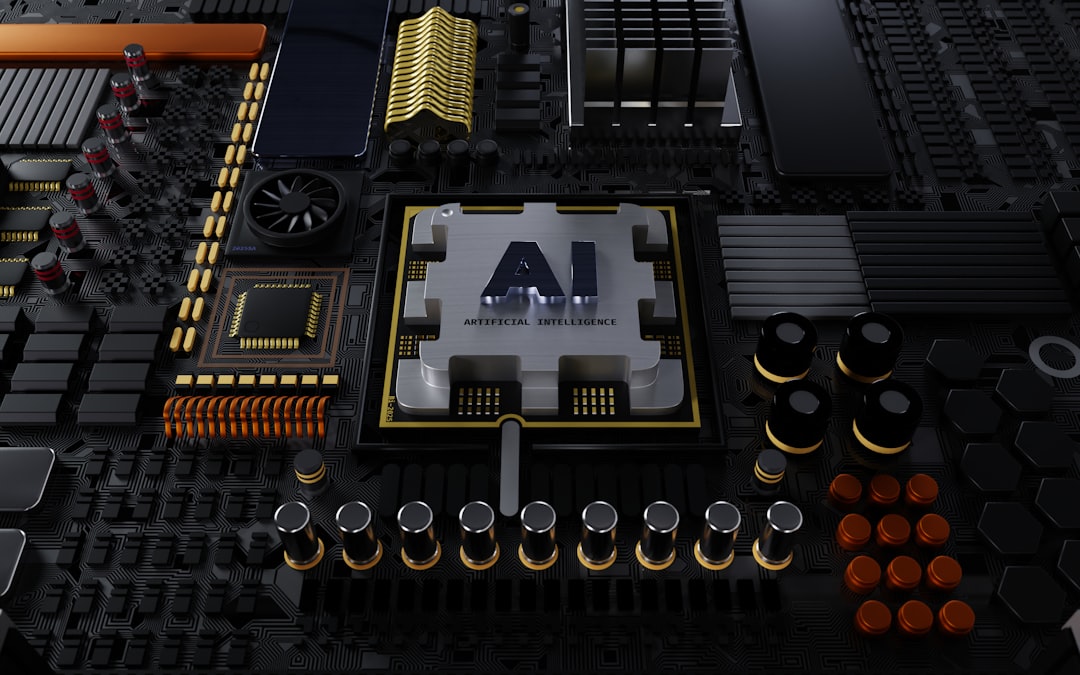
The use of AI in content moderation is sparking important discussions among experts, particularly regarding its ethical implications. Questions around transparency and fairness are paramount, with a growing concern that these systems may not be applied equally across different languages or situations. This has led to the idea of "ethical scaling" being floated as a guiding principle, emphasizing the need to ensure that content moderation resources are distributed fairly and effectively, considering the nuances of different languages and cultures.
However, these systems are not without their critics. Some believe that the development and functioning of AI-driven content moderation is influenced by past biases, such as those related to race and colonial history. There is a valid concern that AI models could reflect and perpetuate these historical prejudices in their decisions about content.
Furthermore, the very nature of AI content moderation introduces a complicated set of ethical trade-offs. While it can be a powerful tool to identify and remove harmful content, it also raises concerns about the limits of free expression and the need for regulation. It's a tricky balance to strike, and requires a nuanced approach that carefully weighs the benefits and drawbacks.
Ultimately, the increasing reliance on AI in content moderation requires ongoing conversations among all stakeholders—developers, platform owners, users, and ethicists. Finding solutions that promote both safety and freedom of expression necessitates careful and continued discussion to navigate the ethical complexities this technology presents.
The ethical dimensions of using AI for content moderation have become a central topic of discussion among researchers, ethicists, and legal scholars, recognizing the significant influence these systems can exert on online conversation and public perception.
Concerns around inherent biases within AI models have prompted researchers to explore methods that ensure fairness, yet the intricate nature of radical content frequently evades detection due to the diverse cultural and contextual subtleties that algorithms might not completely grasp.
Employing deep learning models for content moderation raises questions of responsibility, especially when a system incorrectly labels content—it can lead to unintentional censorship that restricts free expression.
AI systems designed for content moderation intrinsically face challenges concerning openness, as the proprietary nature of their algorithms shields decision-making from scrutiny, making it hard to audit or rectify potential biases.
The real-time processing abilities of AI systems, while beneficial in combating radicalization, present significant moral quandaries surrounding individual privacy, considering the vast amounts of user data gathered without explicit consent.
Integrating user feedback into AI training processes demonstrates a movement towards more participatory models, but it also adds to the question of whose viewpoints influence training, possibly exacerbating pre-existing biases.
Although AI can substantially decrease the workload in content moderation, heavy dependence on it can undermine the role of human moderators, raising worries about appropriately handling sensitive situations requiring empathy and nuance.
The rapid development of online communication, including the rise of new platforms and forms of expression, demands continuous updates to AI models, resulting in a persistent race between the rate of technological adaptation and the swiftness of harmful content production.
Ethical considerations also involve the psychological impacts on users whose content is incorrectly flagged or removed, underscoring the need for dependable appeal processes to rebuild confidence in content moderation methods.
Lastly, the global applicability of AI tools necessitates comprehending and adjusting to varying ethical standards across different cultures, creating complexities in developing globally effective content moderation systems.
Colorize and Breathe Life into Old Black-and-White Photos (Get started for free)
More Posts from colorizethis.io: