Colorize and Breathe Life into Old Black-and-White Photos (Get started for free)
Legal Paper in Pixels Exploring Digital Dimensions for Image Colorization
Legal Paper in Pixels Exploring Digital Dimensions for Image Colorization - Pixel-Perfect Precision Legal Paper Dimensions in Digital Space
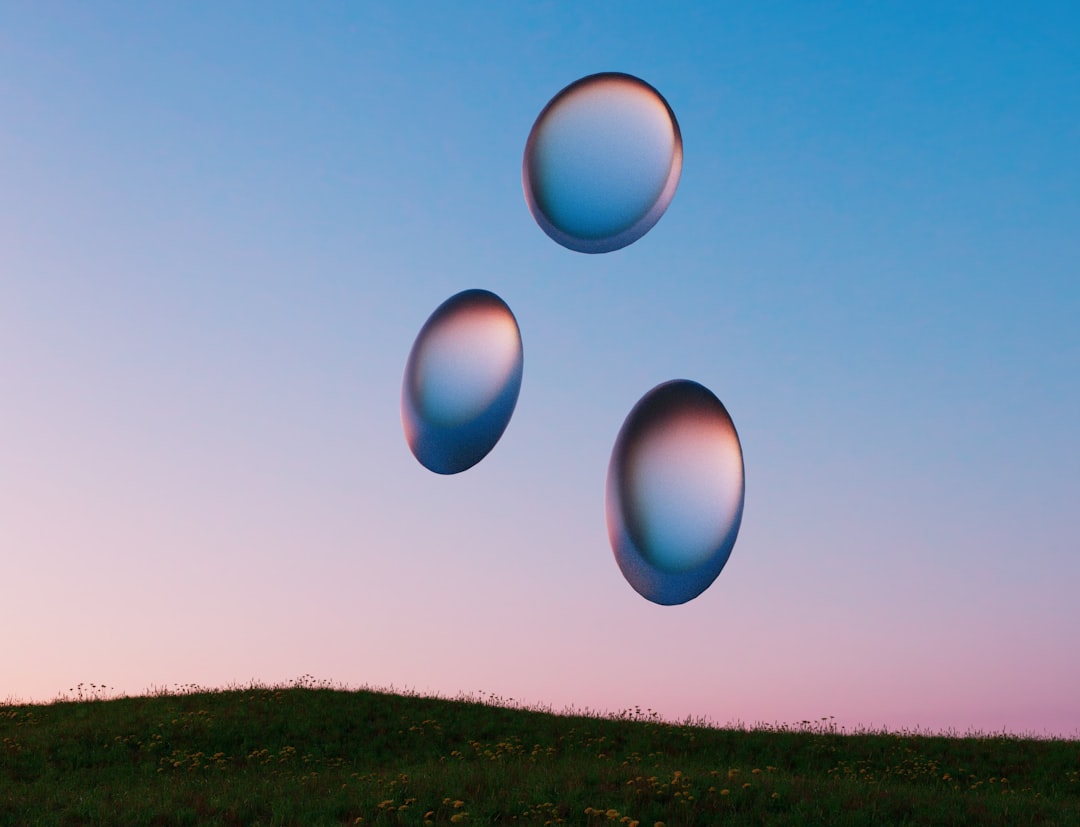
When working with legal documents in the digital realm, getting the pixel dimensions just right is crucial for accurate image colorization. Legal paper, measuring 8.5 inches by 14 inches, translates to 2550 pixels by 4200 pixels at a 300 PPI resolution. This ensures a clear, sharp image, which is important for legal documents that often contain detailed information. The additional length of legal paper, compared to standard letter size, is designed to accommodate the specific needs of the legal field, providing space for lengthy contracts, legal filings, and other complex documents. While this may seem like a small detail, understanding these pixel dimensions is essential for anyone working with legal documents in a digital format. It directly affects the quality of the final output, ensuring that the content is presented clearly and accurately.
The standard dimensions of legal paper, 8.5 inches by 14 inches, translate to a pixel ratio of roughly 2550 by 4200 at a resolution of 300 DPI. But this seemingly simple conversion has some nuanced implications for working with legal documents in the digital realm.
For starters, the unique aspect ratio of legal paper—approximately 1.645:1—poses a unique challenge when shifting between physical and digital formats. This can lead to unexpected issues with text alignment and image placement if not carefully considered.
Furthermore, the color depth of a high-resolution scan can drastically affect the clarity of text on legal documents. Software calibration is crucial to ensure that subtle nuances in line work and text are accurately captured and maintained.
When resizing legal paper images, maintaining the correct aspect ratio is critical. Failure to do so introduces distortions that can compromise the integrity of the document, a potentially serious issue for legal professionals.
The choice of color space—like RGB versus CMYK—impacts how text and images are perceived in the digital realm. This is especially relevant when performing tasks like colorization, where preserving the original tone is critical.
Within the legal field, electronic filing systems often mandate specific pixel standards and resolutions to meet evidentiary guidelines. This means that converting legal paper into digital format requires adherence to specific requirements.
File formats also play a significant role. JPEGs, known for their compression, can result in quality loss, while TIFF files, with their lossless nature, are preferred for archiving legal documents, preserving pixel-perfect detail during digitization.
Scanning legal documents at 600 DPI, while providing higher resolution, can produce exceptionally large file sizes. This can pose challenges for storage and sharing, especially for law firms with large document management needs.
Various software tools employ different algorithms for image processing, including colorization. These variations can significantly influence the final outcome, highlighting the importance of selecting the right tool for legal document needs.
The dimensions of legal paper also play a crucial role in digital signatures. Signature fields must adhere to both legal requirements and digital platform specifications to ensure validity across formats.
Legal Paper in Pixels Exploring Digital Dimensions for Image Colorization - AI-Driven Colorization Techniques Reshaping Grayscale Images
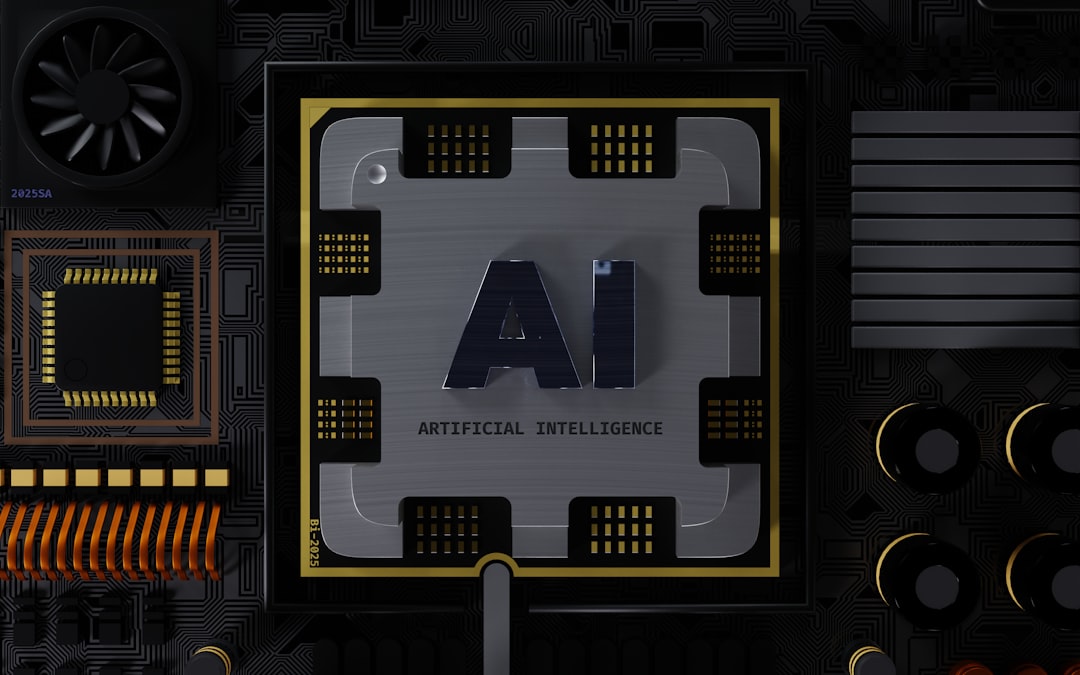
AI-driven colorization techniques are fundamentally altering how we perceive and use grayscale images. By harnessing powerful deep learning models like Convolutional Neural Networks (CNNs) and Generative Adversarial Networks (GANs), these techniques are producing more accurate and lifelike colorized images. This advancement significantly impacts areas such as media and legal documentation, leading to a greater depth of visual engagement.
While automation is enhancing efficiency and precision in colorization, the legal field presents unique challenges. Maintaining pixel-perfect dimensions and preserving the integrity of documents in the digital realm are paramount. The intersection of AI colorization with legal documents requires careful attention to detail to ensure that crucial information remains uncompromised.
Ultimately, the evolving field of AI colorization plays a critical role in enhancing modern media while simultaneously preserving the significance of historical and cultural artifacts. This technology is becoming increasingly impactful, offering a bridge between the past and present, and impacting various aspects of our visual world.
The realm of image colorization is undergoing a fascinating transformation with the advent of AI-driven techniques. These methods leverage convolutional neural networks (CNNs), which essentially learn the intricate relationships between grayscale pixels and color values by processing vast quantities of color images. While this approach significantly surpasses traditional methods relying on manual input, its effectiveness is heavily dependent on the quality and diversity of training datasets. Insufficient variety can lead to biased outcomes, where certain colors are disproportionately favored or specific subjects misrepresented.
One notable aspect of AI colorization involves the use of generative adversarial networks (GANs). These networks pit two neural networks against each other, refining the colorization process and resulting in a more realistic color application to grayscale images. However, a critical eye remains necessary when using these techniques, as they can sometimes introduce artifacts or incorrect colors, especially in areas of complex texture or shading. This is particularly relevant in contexts such as historical document preservation where accuracy is paramount.
Some advanced algorithms go further by incorporating semantic segmentation, which identifies objects within grayscale images. This enhances the accuracy of color placement by taking into account the context and spatial relationships within the image, a crucial aspect for legal and archival documents. Furthermore, deep learning strategies can be used to prioritize certain elements of the image—like text or critical details—ensuring these features remain clear and aren't obscured by color.
To improve the user experience, engineers have introduced feedback mechanisms, allowing for "teaching" of the colorization algorithm. This enables it to adapt based on preferences or historical accuracy, especially valuable when working with legal or ancestral documents.
The balance between adding color and preserving contrast remains a challenge in colorization. Algorithms need to strike a balance that prevents the original details from being washed out. This is especially important when digitizing legal documents, as clarity in text and signatures is paramount.
Interestingly, AI colorization not only enhances aesthetics but can also improve the legibility of scanned archival texts. The introduction of color can guide the eye and provide visual cues that assist in deciphering difficult handwriting or faded print.
The future of AI-driven colorization holds immense promise. One exciting prospect lies in utilizing multi-spectral imaging data, enabling the transformation of grayscale images not just into color, but into a representation that incorporates additional information invisible to the naked eye. This opens up new possibilities for understanding and interpreting historical artifacts.
Legal Paper in Pixels Exploring Digital Dimensions for Image Colorization - Deep Learning Advancements in Image Restoration Since 2014
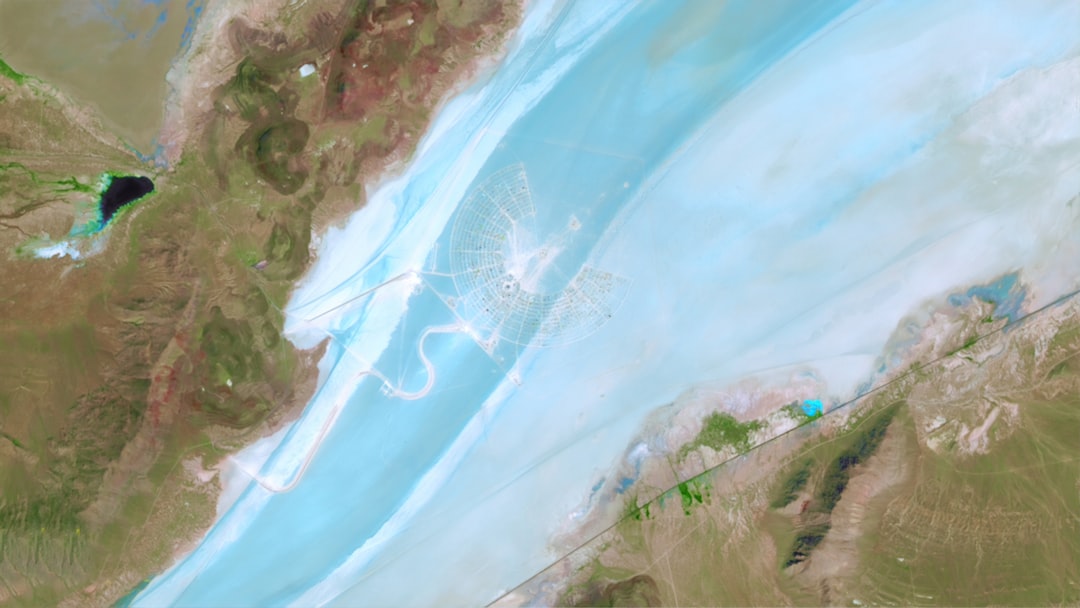
Deep learning has dramatically changed image restoration since 2014. Convolutional neural networks (CNNs) are especially influential in this field. They are particularly adept at recreating details and generating images that more closely resemble the original scene. This has made the restoration process much quicker and more effective. However, even with these improvements, challenges remain. In situations demanding exceptional accuracy, such as legal documents, balancing the enhancements with preserving essential details remains crucial. Preserving clear text and accurate signatures is a key concern. As these AI techniques advance, it is important to remain aware of potential inaccuracies, as they could affect the integrity of the restored image.
Deep learning has completely changed the field of image restoration since 2014. We're not talking about simple noise reduction, but real advancements that can handle complex distortions and artifacts. Convolutional Neural Networks (CNNs) are now commonly used to correct blurred, noisy, or distorted images, which is critical for improving the legibility of legal documents, especially old ones. It's really amazing how effective these techniques are getting.
One of the most interesting advancements is the use of Generative Adversarial Networks (GANs). Instead of just applying a simple filter, GANs train two neural networks against each other, essentially pushing each other to become better. The "generator" tries to create realistic images, while the "discriminator" attempts to spot fake ones. This ongoing competition leads to incredible results, restoring images with a level of detail and fidelity that was previously unimaginable.
Another exciting development is the use of attention mechanisms. These allow the algorithm to focus on specific areas of an image that need the most correction, essentially "paying attention" to the most important parts. This results in more accurate and natural-looking restoration, which is especially important for legal documents, where maintaining the integrity of subtle details like faded signatures is crucial.
Image super-resolution is another area seeing remarkable progress. By interpolating additional data, deep learning techniques can upscale low-resolution images without losing quality, which is a huge boon for digitizing old legal documents that might have been scanned at lower resolutions. The resulting images are clearer and easier to read, and the accuracy of these techniques is improving all the time.
Researchers are even exploring ways to use synthetic data generation to improve training sets. This means creating artificial images based on real samples, which allows models to be more robust and less susceptible to overfitting, especially important for the wide variety of documents found in legal settings.
While we've seen dramatic improvements in deep learning image restoration, the journey continues. One of the current areas of focus is on contextual information. By incorporating the surrounding elements of an image, algorithms can ensure that corrections are made in a way that is consistent with the context. This is important when dealing with complex layouts or documents with multiple textual elements, which is common in legal documents.
Another exciting development is the introduction of variational autoencoders (VAEs). These offer a probabilistic approach to image reconstruction, which can lead to more nuanced restorations by taking into account the inherent uncertainties in the data. This helps create more natural-looking images, which is important for a wide range of applications.
Multi-scale approaches are another area gaining traction. By analyzing images at different resolutions simultaneously, models can extract richer features and produce even more detailed restorations. This is especially helpful when dealing with the diverse quality of digitized legal documents, ensuring that all types of documents can be restored effectively.
Finally, there's increasing focus on explainability in deep learning. This means developing models that can explain their decision-making process, allowing engineers to understand why an image is being restored in a certain way. This transparency is important for building trust in these systems, especially when working with legal documents where accuracy is paramount.
The field of deep learning image restoration is moving at a rapid pace, and we're sure to see many more advancements in the future. These techniques are already making a significant impact on various industries, including law, where they're helping to improve the accessibility and accuracy of critical information.
Legal Paper in Pixels Exploring Digital Dimensions for Image Colorization - Regression vs Classification Approaches in Machine Learning Colorization
The choice between regression and classification methods in image colorization using machine learning significantly influences the final outcome. Regression aims to predict precise RGB values, generating a more nuanced and visually consistent colorization. In contrast, classification categorizes image features into predetermined color classes, often leading to less variation in color application. Both techniques, when powered by deep learning, have achieved significant progress. Yet, they each come with their own strengths and weaknesses: regression potentially offers more detail but necessitates extensive training data, while classification might oversimplify complex images. As these techniques advance, their impact on preserving the integrity of legal documents or historical artifacts becomes increasingly significant. This raises questions about how to balance automation with the careful preservation of detail in crucial contexts. Ultimately, a thorough understanding of these methods is essential to optimize image colorization algorithms, ensuring they meet the specific technical and historical requirements of legal documentation.
In the realm of image colorization, we can use machine learning to achieve the desired results through two main approaches: classification and regression. These approaches differ in their fundamental objectives and the resulting granularity of color prediction.
Classification models categorize data into distinct labels. In the context of image colorization, this might involve assigning broad color categories like "blue," "red," or "green." However, regression models are designed to predict continuous variables, like specific RGB values for each pixel, resulting in a more nuanced and visually rich output.
The use of regression requires more training data and computational resources compared to classification models, as the complexity of representing colors at a fine level demands more intricate neural network architectures.
Evaluating the performance of these methods involves different metrics. For classification, accuracy measures the proportion of correctly labeled pixels. However, regression tasks utilize metrics like Mean Squared Error (MSE) to determine how closely predicted colors match the actual colors in the original image, a crucial aspect for preserving detail.
The choice of approach impacts the potential for introducing artifacts. Classification methods can lead to generalized artifacts, whereas regression methods, while sensitive to noise from pixel-level predictions, can potentially deliver greater detail if adequately trained.
The integration of semantic segmentation with regression models can incorporate contextual understanding, recognizing the relationships between objects in an image, which is less prevalent in classification models. This can result in more realistic color applications.
Furthermore, regression models can adjust to variations in lighting and textures, showcasing greater adaptability than classification models which rely on predefined categories. In archival work, the precision required for detail restoration makes regression more suitable than classification, where broad color themes might suffice.
Ultimately, both approaches heavily depend on the quality of training data. Classification models require a balanced representation of colors, while regression benefits from diverse color gradations to mitigate bias.
The future of image colorization research lies in exploring hybrid techniques that combine the precision of regression with the efficiency of classification, aiming for a balance between detail and computational cost. This could prove particularly valuable in legal contexts where accuracy is paramount.
Legal Paper in Pixels Exploring Digital Dimensions for Image Colorization - The Need for Standardized Benchmarking in Colorization Research
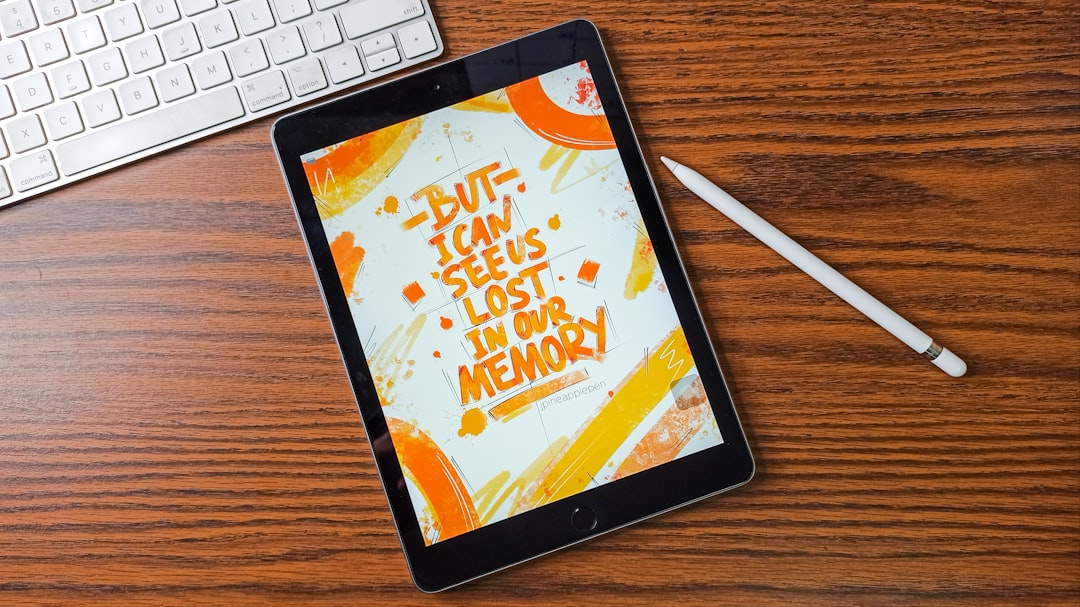
In the ever-evolving field of image colorization, the importance of establishing standardized benchmarking practices is becoming more crucial. The rapid advancements in deep learning have brought about a wide array of different methods, but the lack of a uniform system for assessing these methods can hinder progress and innovative research. Having consistent benchmarks would enable researchers to effectively evaluate the performance of different algorithms, fostering meaningful comparisons and collaborations. As models grow more intricate, clear and consistent evaluation metrics are vital to ensure that results are reproducible and can be built upon. Ultimately, adopting standardized benchmarking practices will elevate the quality of colorization research, promoting accountability and driving improvements in both aesthetics and accuracy, particularly in sensitive areas like legal documentation.
The lack of standardized benchmarking in colorization research is a significant roadblock to progress. It's hard to know if new algorithms are truly better because researchers often use different datasets and metrics, making direct comparison impossible. This creates an environment where misleading claims about effectiveness are commonplace.
It's essential to have standardized datasets with controlled variables so that we can compare new techniques against existing ones. Using well-known datasets like ImageNet or COCO could provide a common ground for evaluating algorithms. But even here, the benchmark can sometimes lead to bias – algorithms might excel on a particular benchmark but be ineffective in real-world scenarios, such as legal documents. We need to use benchmarks that are diverse and relevant to the specific application, exposing any limitations or biases in the models.
Metrics like Intersection over Union (IoU) and pixel-wise accuracy are crucial for quantifying the qualitative aspects of colorization. This is especially important in legal contexts where text clarity is paramount. However, the inconsistency of metrics used in current research makes it difficult to compare models and understand their real-world capabilities.
Community-driven benchmarks can encourage collaboration and accelerate innovation. Challenges in other areas of research have led to significant advancements. We need a benchmark that includes a wide range of images, particularly those relevant to legal documents, so that we can fully understand the qualities of the colorization techniques being developed. Without a strong foundation of rigorous, standardized benchmarking, creating robust models that meet the exacting requirements of legal documentation will remain a challenge.
Legal Paper in Pixels Exploring Digital Dimensions for Image Colorization - Addressing Artifacts and Information Loss in Digital Colorization
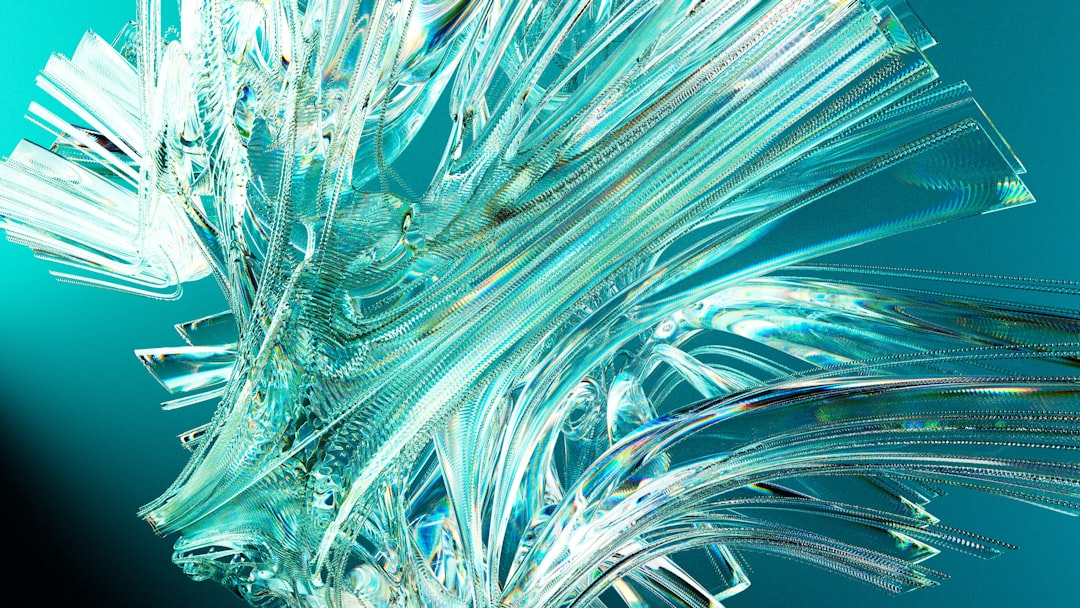
Addressing artifacts and information loss in digital colorization is a critical issue, especially when working with sensitive materials like legal documents. While AI-powered techniques like convolutional neural networks (CNNs) and generative adversarial networks (GANs) have significantly advanced colorization, they still present challenges. These challenges include artifacts, which are unwanted distortions, and the loss of detail information during the colorization process. These issues can compromise the integrity of the original image and hinder its usability in legal settings, where clarity and accuracy are paramount. To address these concerns, researchers are developing strategies for artifact reduction and detail preservation, aiming to achieve a balance between visually appealing colorization and the fidelity of the original image. Ultimately, understanding the complexities of these methods is essential for optimizing colorization processes and ensuring the preservation of vital information in legal documents.
The pursuit of accurate digital image colorization is rife with challenges, especially when dealing with the sensitive nature of legal documents. While recent advancements in deep learning have significantly improved colorization techniques, ensuring the fidelity of legal documents presents unique hurdles. One critical concern is the unintentional introduction of artifacts during the process. These artifacts, such as banding or color inconsistencies, can drastically alter the visual representation of the document, compromising its integrity, especially when it comes to text clarity and subtle detail.
Another major hurdle is the potential loss of vital information due to compression techniques employed in various file formats. As legal documents often contain crucial textual and visual details, high levels of compression can lead to significant data loss, potentially rendering them illegible or even inaccurate.
The choice of color space is another vital consideration. RGB, widely used for digital displays, might not always accurately represent the colors intended in legal documents, especially when converting from traditional printed formats.
While deep learning models have revolutionized image colorization, relying heavily on their accuracy comes with its own set of challenges. The quality and diversity of the training dataset can directly impact the model's effectiveness. Insufficient or biased datasets can lead to inaccurate color applications or unintended color distortions.
Furthermore, a delicate balance must be struck between adding color and preserving the original text's clarity. Certain colorization techniques can inadvertently obscure text elements, which is unacceptable in legal contexts.
Regression models, despite their potential for producing more nuanced and detailed colorization, demand extensive training data, a significant obstacle given the diverse nature of legal documents.
To combat these challenges, advanced techniques like semantic segmentation are being employed. This approach, incorporating contextual understanding of the image, helps ensure that colors are accurately placed based on the relationship between objects within the document. This, in turn, minimizes the risk of misrepresenting critical legal information.
However, the lack of standardized benchmarking methods remains a significant hurdle in objectively evaluating the effectiveness of different colorization algorithms. Without reliable benchmarks, it's difficult to determine whether new techniques truly offer improvements or are merely variations on existing methods.
Generative Adversarial Networks (GANs) offer a powerful tool for creating incredibly realistic colorized images, but their application in the legal domain requires careful consideration. There is always the risk of these networks introducing artificial features or colors that might conflict with the historical context of the document.
The varied quality of legal documents themselves presents another challenge. Documents from different eras and preservation states require unique processing techniques, as lower-quality scans inherently make it more difficult to accurately retain detail after colorization.
Ultimately, navigating the complexities of digital image colorization in the legal sphere necessitates a meticulous approach. The need for accurate and reliable colorization solutions that respect the integrity and accuracy of legal documents is paramount.
Colorize and Breathe Life into Old Black-and-White Photos (Get started for free)
More Posts from colorizethis.io: