Colorize and Breathe Life into Old Black-and-White Photos (Get started for free)
Pascal VOC Dataset in 2024 Analyzing Its Continued Relevance in Modern Computer Vision
Pascal VOC Dataset in 2024 Analyzing Its Continued Relevance in Modern Computer Vision - Legacy and Impact of Pascal VOC in Computer Vision Research
The Pascal VOC dataset's influence on computer vision research is undeniable. Its role in establishing standardized datasets and evaluation protocols for object recognition tasks has been pivotal. The annual challenges, running from 2005 to 2012, acted as a catalyst for progress by enabling researchers to train and assess their algorithms on a common set of data encompassing a wide range of everyday objects. This created a competitive arena that pushed the boundaries of object recognition and detection, leading to significant breakthroughs in the field. The metrics used to judge these competitions have become integral to modern computer vision research, used as benchmarks for new techniques. Although influential, the dataset's limitations with respect to the diversity and complexity of real-world scenarios should not be overlooked. The continued use of VOC serves as a testament to its impact while simultaneously highlighting the need for ongoing development of datasets capable of addressing current challenges in the field.
The Pascal VOC dataset's enduring legacy stems from its role in establishing a common ground for object recognition research. Its introduction of standardized image datasets and evaluation procedures, starting with the Pascal VOC challenge in 2005, provided a consistent platform for researchers to compare and contrast their algorithms. This structured approach, which spanned a period until 2012, helped elevate the field by fostering a spirit of competition and shared progress.
The impact of VOC extended beyond object detection. It spawned challenges in image segmentation, action classification, and other visual understanding tasks, showcasing the breadth of its applicability. These annual challenges, coupled with the dataset's accessibility and a focus on a curated set of real-world objects, spurred a wealth of research, resulting in a significant body of publications that leverage the dataset.
The VOC challenges, in essence, created a benchmark for model performance. Models trained on VOC and performing well often exhibit good generalization to real-world scenarios, highlighting its importance as a stepping stone in building practical computer vision solutions. Researchers still regularly utilize the dataset to assess their models against established benchmarks.
The dataset’s innovation wasn't limited to providing images. It introduced detailed annotations like object bounding boxes and segmentation masks, which were instrumental in training increasingly complex and precise object recognition models. The design maintained a balance between manageable size and task difficulty, enabling researchers, even those new to the field, to tackle meaningful challenges.
While larger and more complex datasets are now common, the simplicity of VOC continues to hold value. Researchers appreciate its straightforward nature as it allows them to isolate algorithmic improvements without the noise of overly-large or diverse datasets. The influence of VOC is even seen in modern deep learning through transfer learning techniques, where models initially trained on VOC are fine-tuned for different applications.
However, some criticisms linger regarding the limited variability of scenes within VOC. This can potentially lead to model overfitting on a specific set of characteristics. Nevertheless, despite its limitations, the dataset still plays a critical role as a foundational benchmark against which we evaluate the generalization ability of more contemporary computer vision algorithms. Its influence remains tangible in ongoing research, solidifying its importance as a historical stepping stone in the advancement of the field.
Pascal VOC Dataset in 2024 Analyzing Its Continued Relevance in Modern Computer Vision - Evolution from VOC2007 to VOC2012 Key Differences and Applications
The progression from PASCAL VOC2007 to VOC2012 showcases a notable evolution in dataset design, specifically geared towards enhancing its suitability for training machine learning models. VOC2012 brought about improvements like expanded image collections and more detailed annotations, offering a richer environment for assessing object detection and segmentation techniques. This refinement wasn't just about adding more data; it reflected a growing awareness of the intricate challenges involved in visual understanding, necessitating annotations that captured finer details within varied scenarios. The refinements incorporated in VOC2012 have left a lasting imprint on later developments within computer vision, setting the stage for subsequent datasets and methodologies. While newer datasets have naturally emerged to address more modern concerns, the foundational framework offered by VOC2012, particularly in defining the core challenges of object recognition, remains relevant and insightful. It provides a crucial point of comparison, underscoring the continuous journey of research and development within computer vision.
The evolution from VOC2007 to VOC2012 brought about some notable changes and enhancements, impacting how researchers approached object recognition and related tasks. One of the key improvements was the expansion of the object categories from the original 10 to 20 in VOC2012. This broader set of objects allowed for the training and evaluation of models across a wider range of visual understanding problems.
Beyond just quantity, VOC2012 also focused on refining the quality of the annotations. The addition of more detailed object segmentations proved to be valuable for tackling more intricate challenges, especially in image segmentation, a field that saw increased attention because of this change. It's intriguing how the addition of these detailed annotations shifted the emphasis towards pixel-level understanding, stimulating the development of segmentation-specific algorithms.
A notable difference introduced in VOC2012 is the inclusion of a validation set, absent in its predecessor. This enabled researchers to fine-tune hyperparameters more effectively, which wasn't explicitly addressed in the earlier dataset. This feature seems to have been vital in ensuring more reliable evaluations of model performance.
VOC2012 also significantly increased the number of images compared to VOC2007. The initial dataset had about 9,000 images, while VOC2012 pushed this number closer to 11,000. This increase addressed previous criticisms surrounding the dataset's size and its ability to represent real-world variability. Larger, more varied datasets were seen as a way to make models more robust.
While VOC2007 primarily emphasized detection tasks, VOC2012 expanded its scope to include a strong emphasis on segmentation tasks. The addition of annotated segmentation masks made it a highly valuable tool for those focusing on both object recognition and more comprehensive scene understanding, pushing the field towards a deeper understanding of visual information.
The evaluation methods were also standardized further in VOC2012. The introduction of mean average precision (mAP) calculated over various intersection-over-union (IoU) thresholds provided a more refined and nuanced assessment of model performance compared to the single IoU threshold used in VOC2007. This change seems like a step toward a more realistic representation of a model's capability in real-world applications.
Community engagement seems to have grown as well in the VOC2012 competition. The addition of new tasks like object detection in videos and action recognition helped expand the range of visual understanding challenges tackled. The crossover between these different subfields resulted in more cross-disciplinary research within the computer vision community, encouraging researchers to bridge various aspects of the field.
Furthermore, the images within VOC2012 tended to show more complex scenes compared to those in VOC2007. This shift towards more challenging environments helped drive the development of more advanced and robust algorithms capable of tackling the intricacies of real-world situations, where various factors and conditions impact object recognition.
A crucial shift occurred with the rise of deep learning models during the VOC2012 competition. It played a critical role in validating and pushing the adoption of convolutional neural networks (CNNs) as a dominant approach for tackling object recognition and other related visual tasks. It appears that VOC2012 served as a key turning point in the broader shift towards deep learning within the field.
Despite all these advances, the VOC2012 dataset maintained a relatively focused scope compared to newer large-scale datasets like MS COCO. This has sparked debate regarding the importance of domain-specific datasets versus the need for highly diverse, large datasets to achieve substantial progress in computer vision. It's fascinating how the debate continues even today about the ideal characteristics of a training dataset for various tasks.
Pascal VOC Dataset in 2024 Analyzing Its Continued Relevance in Modern Computer Vision - Pascal VOC Challenge 2005-2012 Contributions to AI Development
The Pascal VOC Challenge, active from 2005 to 2012, significantly impacted the progress of AI, especially within the field of computer vision. Its open-source dataset, along with standardized evaluation tools and a common framework, allowed for systematic assessment of object recognition algorithms. The annual challenge motivated researchers to develop more precise models, pushing the boundaries of what was possible in areas like object detection and segmentation. Notably, the metrics established during this period remain important benchmarks in computer vision research today. Though the formal competitions ended, the dataset remains relevant for current computer vision research and applications, serving as a core point of reference. However, its limited representation of real-world environments highlights a persistent need for datasets that are more diverse and complex, ultimately pushing computer vision models to achieve greater accuracy and robustness.
The Pascal VOC Challenge, active from 2005 to 2012, significantly influenced the development of common evaluation practices in computer vision, with the mean Average Precision (mAP) becoming a widely adopted metric for judging object detection models. Notably, the competitions were open to both academic and industry teams, creating a vibrant and collaborative environment that spurred innovation in the field.
The shift from VOC2007 to VOC2012 brought about more than just an increase in the number of images. The enhanced annotation quality, particularly in segmentation tasks, compelled researchers to develop more sophisticated algorithms capable of handling intricate object shapes and boundaries. This period also witnessed the rise of Convolutional Neural Networks (CNNs), with VOC2012 proving to be a critical proving ground that helped establish CNNs as a dominant force in computer vision.
The expanding range of object categories, increasing from 10 in VOC2007 to 20 in VOC2012, highlighted the importance of training models on more diverse datasets to improve their ability to generalize across different real-world scenarios. Researchers value the VOC dataset for its thoughtful balance, as its relatively manageable size permits focused experimentation, a trait that stands in contrast to more recent datasets that can sometimes introduce excessive complexity and hinder focused investigation.
The inclusion of validation sets in VOC2012 proved to be a pivotal step forward. It facilitated more precise tuning of model parameters, leading to more reliable evaluations of performance – an aspect of model development that had previously received less attention. The expanded scope of challenges in VOC2012, beyond basic object detection, encouraged research spanning action recognition and video analysis, promoting cross-disciplinary work within the computer vision community.
It's important to acknowledge that while the VOC challenges were instrumental in establishing foundations for object recognition, they also illuminated a critical need for datasets capable of capturing a broader array of visual contexts. This was to help mitigate the potential for models to become overly specialized to the specific characteristics found within the VOC dataset.
Despite the emergence of newer and larger datasets, the rigorous structure and evaluation standards established by the Pascal VOC series remain influential. The lessons learned about robust model evaluation methods continue to inform best practices within the field, encouraging a more standardized and comparative approach to benchmarking computer vision technologies.
Pascal VOC Dataset in 2024 Analyzing Its Continued Relevance in Modern Computer Vision - Current Role of Pascal VOC in Training Modern AI Models
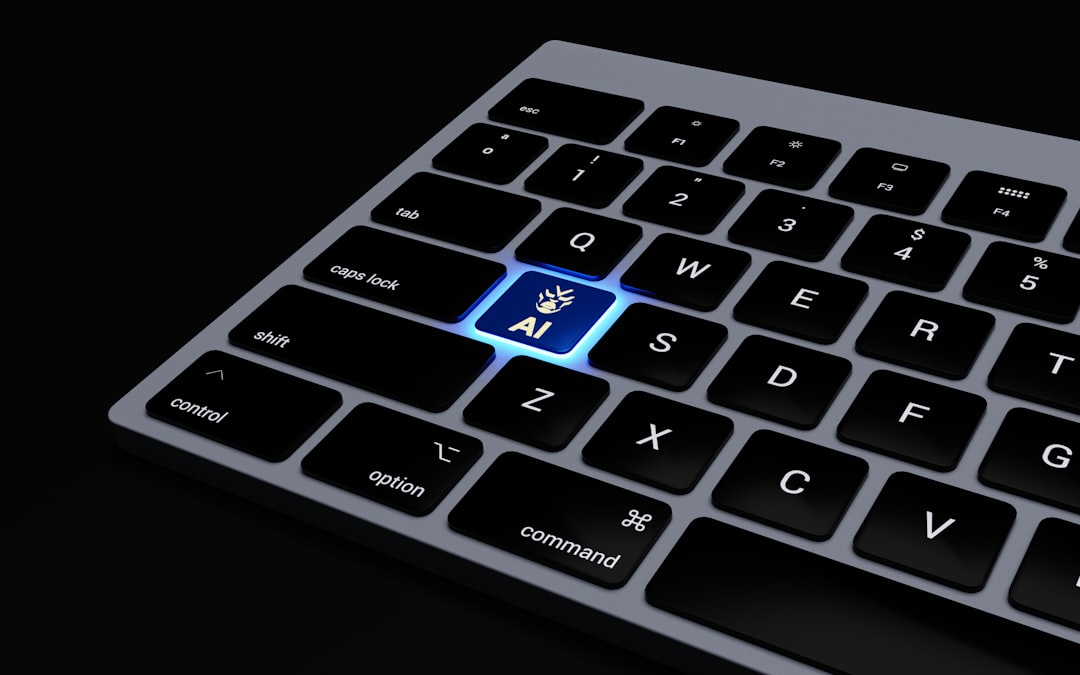
The Pascal VOC dataset continues to play a role in training modern AI models, primarily within computer vision. It serves as a valuable benchmark, particularly for object detection and segmentation tasks, thanks to its standardized annotations that help researchers evaluate the performance of different algorithms. While the dataset's scene variety is limited, it remains a foundational resource that supports experiments and comparisons among modern AI models. Researchers find its simplicity and manageable size appealing, allowing them to concentrate on specific improvements in algorithms without the overwhelming scale and complexity often seen in newer, larger datasets. The lasting impact of Pascal VOC stems from its contribution to the development of reliable evaluation metrics, consequently shaping the overall progress of AI within the field of computer vision. However, its limitations push researchers to explore more complex datasets that better reflect real-world scenarios.
The Pascal VOC dataset, while not the largest or most complex, continues to play a surprising role in the training of modern AI models, particularly in computer vision. Its enduring relevance stems from several factors. First, the evaluation metrics established during the VOC challenges, particularly mean Average Precision (mAP), remain standard benchmarks across various tasks like object detection, segmentation, and image retrieval. This consistency offers a valuable way to compare the performance of different models and approaches.
Second, many pre-trained models used today are initially trained on VOC. This serves as a foundation, allowing newer models to leverage the features learned from VOC, leading to quicker training and improved performance when tackling more complex tasks with limited labelled data – a technique known as transfer learning.
Third, the focused structure and carefully annotated data within VOC have influenced the design of newer datasets. While datasets like MS COCO have gained popularity, VOC's effectiveness in specific tasks, especially those needing high-quality annotations, serves as a benchmark.
Fourth, researchers continue to find VOC useful for isolating improvements in specific algorithms. Its manageable size and well-defined tasks create a controlled environment for experiments, a valuable contrast to larger datasets where diverse data can mask individual improvements.
VOC's impact extends to segmentation advancements as well. The improvements in segmentation capabilities in VOC2012 drove the development of deep learning architectures that can handle intricate object boundaries and overlapping objects, pushing the field towards a deeper understanding of pixel-level information within images.
Despite limitations like a less-diverse set of scenes, VOC remains a crucial point of reference for evaluating new computer vision methods. This is due to its standardized evaluation practices, often cited in current research.
The gradual incorporation of more intricate scenes in VOC2012 helped researchers develop models capable of more accurate recognition tasks found in the real world, particularly in handling situations with occlusions and multiple objects.
The inclusive nature of the VOC challenges led to collaboration between academia and industry, resulting in numerous publicly accessible solutions that have directly influenced practical applications and commercial computer vision products.
Further, VOC's clear structure and comprehensive documentation make it an excellent educational resource for new researchers. It provides a solid starting point for understanding core computer vision principles before venturing into larger, more intricate datasets.
Finally, even with newer datasets, ongoing research using VOC continues to generate new insights and methodologies, reaffirming its enduring value. It's a remarkable bridge between classical and modern computer vision approaches, demonstrating that foundational datasets can continue to influence cutting-edge research.
Pascal VOC Dataset in 2024 Analyzing Its Continued Relevance in Modern Computer Vision - Pascal VOC vs COCO Dataset Comparative Analysis in 2024
In 2024, when comparing the Pascal VOC and COCO datasets, we see clear distinctions in their design philosophies and the kinds of tasks they excel at. Pascal VOC, with its simpler XML format, is straightforward to use and focuses on well-defined object detection and segmentation tasks. COCO, in contrast, has a more complex JSON structure, capable of handling a greater variety of visual tasks and permitting richer annotations. Both, however, struggle with issues like labeling errors, which disproportionately affect how well they handle small object detection. This is a shared weakness that future dataset developments should aim to improve. Although COCO has become very popular due to its scale and diverse content, Pascal VOC maintains a strong position as a benchmark, particularly because of its role in shaping the way we measure and evaluate progress in object recognition. Its lasting influence highlights the importance of both datasets in driving progress in the field of computer vision.
The Pascal VOC and COCO datasets, while both influential in computer vision, differ significantly in scale, annotation complexity, and scope. Pascal VOC, with its roughly 11,000 images, offers a more manageable dataset for researchers, particularly for initial model training and comparisons. In contrast, COCO boasts over 330,000 images and a wider array of real-world scenarios, aiming to create more robust models but at the cost of increased complexity.
VOC annotations primarily concentrate on bounding boxes and segmentation, providing a good foundation for object recognition. COCO expands this by including detailed annotations like keypoints for human pose estimation, allowing for more nuanced understanding of human interactions. This added complexity could lead to more insightful models, particularly for tasks requiring intricate human interaction recognition.
VOC encompasses 20 object categories, while COCO extends this to 80, offering a broader spectrum of object recognition training. This wider range in COCO gives models a better chance at generalizing to unseen objects, lessening the risk of becoming overly specialized to the objects found within VOC.
Historically, Pascal VOC challenges focused on particular computer vision tasks like object detection and segmentation. COCO, on the other hand, has incorporated numerous challenges including caption generation and multiple object detection in a single image, showing a greater breadth of artificial intelligence applications.
The evolution of these datasets also reflects shifts in deep learning. VOC, notably VOC2012, played a pivotal role in validating CNNs. COCO has emerged as the benchmark for newer models that utilize attention mechanisms and transformer architectures, highlighting the continuous drive for improvement in computer vision algorithms.
Both datasets use mAP as a performance metric, yet COCO takes a more refined approach with multiple IoU thresholds. This refinement fosters a more in-depth evaluation of model performance across various overlap levels, encouraging a more thorough evaluation methodology.
Despite COCO's popularity, a large number of modern model architectures still utilize Pascal VOC for initial pre-training. The simpler structure of VOC serves as a beneficial starting point before models are further refined on more complex datasets like COCO. This highlights the value of simpler datasets in establishing a strong foundation.
Scenes in VOC tend to be relatively straightforward, while COCO’s scenes are more complex, incorporating diverse objects, occlusions, and intersections. This increased complexity challenges models to handle more realistic scenarios that might not be adequately represented in VOC.
The initial Pascal VOC challenges fostered a significant community of researchers and industry professionals, resulting in a vast pool of shared resources. COCO, while promoting collaboration, doesn't seem to carry the same competitive spirit that drove the VOC community forward.
Researchers frequently leverage VOC as a standard for benchmarking new computer vision methodologies even in the presence of larger datasets like COCO. This speaks to the enduring significance of VOC in providing a reliable foundation for testing new ideas and algorithms in the ever-evolving field of computer vision.
While both datasets have their strengths, understanding the differences between VOC and COCO can help researchers make informed decisions regarding the most appropriate choice for their specific research needs. Each has its place within the evolution of computer vision, from foundational building blocks (VOC) to more expansive, complex benchmarks (COCO).
Pascal VOC Dataset in 2024 Analyzing Its Continued Relevance in Modern Computer Vision - Future Prospects of Pascal VOC in Emerging Computer Vision Tasks
The Pascal VOC dataset's future in the realm of emerging computer vision tasks presents a mixed outlook. While its historical role in establishing object detection and segmentation benchmarks continues to be valuable for research, its inherent simplicity and narrow focus pose questions regarding its adaptability to newer, more intricate tasks. Tasks demanding more complex scenes and nuanced annotations may not be ideally suited to VOC's current design. The dataset remains a valuable tool for honing algorithms and providing a controlled setting for experimentation, thus contributing to algorithmic advancements. However, as the field pushes boundaries into increasingly complex applications, the suitability and relevance of the VOC dataset will require continuous reevaluation and potential adaptation to ensure it stays a relevant resource for the modern computer vision researcher.
The Pascal VOC dataset continues to be a valuable resource, especially in areas like autonomous driving and robotics where object recognition is crucial. Its simple, structured format minimizes data preprocessing steps, enabling engineers to focus on refining algorithms.
Even with the rise of more extensive datasets like MS COCO, the original Pascal VOC challenges remain essential for developing new architectures. Researchers frequently use VOC as a benchmark to gauge the effectiveness of innovative methods in various object detection tasks, evaluating the improvements in accuracy.
The relatively small number of categories in VOC (20 classes) fosters a concentrated approach to model training. This focused training is particularly beneficial for tasks needing high precision and reliability, like medical image analysis and facial recognition, where models must generalize well with limited classes and avoid overfitting.
As computer vision tackles increasingly complex tasks, the detailed annotations offered by Pascal VOC, including pixel-level segmentation, remain pertinent. Researchers working on fine-grained segmentation, like instance segmentation in images, still rely on VOC for its meticulous object boundary annotations.
The structured evaluation framework established by the VOC challenges has set a standard for newer datasets. Many emerging datasets borrow from VOC's methodology to ensure rigorous performance evaluation, illustrating the importance of clear, quantitative metrics.
Researchers still use transfer learning with models initially trained on Pascal VOC before applying them to more challenging tasks. This approach leads to quicker model convergence and reduces the need for massive amounts of data, highlighting the dataset's foundational role in modern AI development.
Breakthroughs in deep learning, especially during the VOC challenge years, were influenced by innovations emerging from the Pascal VOC dataset. Many influential architectures were initially validated using VOC data, underscoring its ongoing relevance in shaping contemporary AI strategies.
The adaptability of Pascal VOC's annotations has led to its use in evolving visual tasks, such as zero-shot learning and generative modeling. These areas leverage the existing structure while pushing AI towards understanding unseen classes, showcasing VOC's educational role in advancing computer vision.
Many new algorithms developed for intricate visual tasks often revisit Pascal VOC for ablation studies, where individual algorithmic changes can be assessed empirically. This helps researchers isolate variables and understand how innovations impact performance, confirming VOC's role as a testing ground.
Despite its limited scene diversity, the controlled environments of Pascal VOC offer a unique advantage for debugging and troubleshooting emerging computer vision models. Researchers can more readily identify issues in machine learning workflows without the distractions of overly complex environments found in larger, more diverse datasets.
Colorize and Breathe Life into Old Black-and-White Photos (Get started for free)
More Posts from colorizethis.io: