Colorize and Breathe Life into Old Black-and-White Photos (Get started for free)
Precision Blurring A Deep Dive into Selective Image Obfuscation Techniques for 2024
Precision Blurring A Deep Dive into Selective Image Obfuscation Techniques for 2024 - DeepBlur Revolutionizes Latent Space Blurring for Image Privacy
DeepBlur offers a fresh approach to obscuring images by blurring them within the latent space of pre-trained AI models. This technique focuses on safeguarding privacy by making faces harder to recognize, both by machines and people. It stands out from traditional methods, like pixelation or blurring with a Gaussian filter, because it manages to keep images looking good while still altering the information that identifies individuals. The need for better privacy solutions is increasingly important given how widespread social media and surveillance have become, along with the advancements in facial recognition. DeepBlur tackles a key limitation of older privacy methods—they often produce lower-quality images and are prone to being reversed. It potentially establishes a more robust system against future attacks that exploit AI, providing a strong tool for preserving the privacy of sensitive visual data.
DeepBlur operates by intricately manipulating the latent space of a pre-trained generative model, leading to significant image distortion while remarkably preserving the core elements of the image. This is a vital aspect for maintaining the image's functionality, for instance, in scenarios where the image's overall context matters.
It's not just about protecting sensitive features but also handles diverse image complexities quite effectively, hinting at its potential application in a wide array of situations, from everyday street scenes to more specific contexts like private documents.
Rather than the blurry, indistinct outputs often seen with traditional methods, DeepBlur takes a more intelligent approach to blurring by selectively focusing on areas based on their significance within the scene.
The core of DeepBlur's operation lies within deep learning frameworks, utilizing convolutional neural networks to dynamically assess the content and adjust the blur level accordingly. It's a very adaptive method.
DeepBlur is poised to reshape image privacy solutions across areas like law enforcement and healthcare, where the need for secure data management is rapidly growing, presenting an innovative solution in these vital fields.
Intriguingly, the level of blur can be tailored based on the distance of the viewer from the image. This creates a flexible privacy approach that can be adapted to the perceived risk within a given environment.
In contrast to pixelation techniques that tend to create a rather artificial appearance, DeepBlur generates more natural-looking blurred outputs. This can help to avoid unwanted attention or suspicion that severely distorted images might cause.
The method is thoughtfully designed to optimize performance, which can be challenging in real-time applications where image processing needs to be swift. Its efficient nature ensures that the blurring process doesn't impact the overall system performance.
Furthermore, DeepBlur incorporates feedback loops to learn from user interactions and choices. This continuous learning process refines its blurring accuracy and enhances its target sensitivity over time.
The strength of DeepBlur stems from its multidisciplinary foundation, combining elements of computer vision, machine learning, and human perception. By drawing on these diverse fields, it aims to effectively address the complex interplay of technical and ethical aspects inherent in image obfuscation.
Precision Blurring A Deep Dive into Selective Image Obfuscation Techniques for 2024 - Balancing Perceptual Quality and Facial Recognition Prevention
In our increasingly interconnected world, the need to balance the perceptual quality of images with the prevention of facial recognition is becoming more critical. The rise of surveillance technologies and the vast proliferation of visual data online have heightened privacy concerns, pushing the development of more sophisticated image obfuscation methods. Techniques like DeepBlur highlight a promising approach, capable of effectively obscuring faces while maintaining a high level of visual quality. This is crucial for preserving the natural appearance of images within real-world applications.
However, striking this balance is not without its difficulties. Traditional methods like blurring or pixelation, while simple, often significantly degrade image quality, making them vulnerable to advanced recognition algorithms. Therefore, the development of new techniques must carefully navigate the tension between sufficient obfuscation and the preservation of visual fidelity. As researchers continue to explore the landscape of image obfuscation, a central challenge will be ensuring that the methods employed offer a strong level of privacy protection without compromising the usefulness of the visual data itself. Ultimately, the success of any solution will be judged on its ability to effectively reconcile these seemingly conflicting goals.
Balancing the preservation of image quality with the prevention of facial recognition presents a fascinating challenge. Improving the blur while retaining the core essence of an image, like its overall context, requires a careful balancing act. Simply increasing blur isn't always the answer, as it can lead to a point of diminishing returns for visual fidelity. We need to understand the trade-offs involved and where visual quality starts to suffer.
The context of an image plays a huge role in how effectively an obfuscation technique like DeepBlur works. This means we can't apply a simple, one-size-fits-all blurring approach. Understanding which parts of an image are most important for both humans and facial recognition algorithms is key to developing a nuanced approach. This ties into how humans perceive images—our visual system relies on recognizing edges, colors, and textures. We must understand how to manipulate these features to obscure faces without causing a severe drop in overall image quality.
DeepBlur utilizes adaptive learning to hone its blurring techniques. These advanced algorithms try to figure out which visual features are most important for facial recognition and how to selectively obscure them. This continuous learning aspect helps ensure the balance between privacy and visual perceptibility remains optimal over time. However, implementing this in real-time scenarios, like video surveillance, presents significant challenges. The system must make rapid decisions about where to apply blur and how strongly without creating lags or hindering the performance of the entire system.
The constant improvement of facial recognition algorithms necessitates a concurrent evolution of image obfuscation methods. If recognition systems become more sophisticated at picking out faces, blurring techniques need to become smarter, too. We also need to keep in mind how our own cognitive biases can inadvertently influence the design of blur techniques. If we're not careful, we might create a blurring pattern that's more easily recognized by the human brain because it caters to our inherent bias to quickly identify faces. This highlights a critical aspect—it's not just about computers but also about how people perceive images. We need to maintain enough key elements for users to comprehend an image but avoid giving away identifying information.
The ethics of applying obfuscation techniques to images in various situations raises fundamental questions. We need a more comprehensive framework to address who has a right to obfuscate images and in what contexts. Blurring faces might raise privacy concerns, but depending on the images, it could also raise questions about transparency and freedom of expression.
Furthermore, we need to ensure that the drive to increase security doesn't accidentally degrade the usability of an application or platform. Users might find overly-obfuscated images hard to use, thus leading to poor user experiences and diminished system functionality. The balance between performance and security needs careful consideration.
To build truly effective obfuscation techniques, we need to combine elements from different fields. Computer science plays a vital role in developing the algorithms, but psychology offers insights into human perception, while design principles help guide the implementation so that it's user-friendly. Ultimately, a collaborative effort across these areas is critical for establishing a truly universally effective and ethical image obfuscation solution.
Precision Blurring A Deep Dive into Selective Image Obfuscation Techniques for 2024 - Comparative Analysis of Traditional vs.
Advanced Obfuscation Methods
The field of image obfuscation has seen a shift towards more sophisticated methods, particularly with the advent of advanced techniques like DeepBlur. Traditional methods, including pixelation and simple blurring, often result in a noticeable drop in image quality and have proven vulnerable to increasingly powerful facial recognition systems. These limitations highlight a need for a more comprehensive approach to image obfuscation. Advanced methods like DeepBlur leverage deep learning to achieve a more refined level of obfuscation, specifically targeting facial features while preserving the overall structure and context of the image. This nuanced approach suggests that a new generation of obfuscation tools is needed – ones that can both counteract advanced recognition techniques and remain adaptable to different scenarios, prioritizing both privacy and usability. This comparative analysis emphasizes the critical need to find a balance between maintaining high perceptual image quality and ensuring strong privacy protection. The ongoing development and assessment of various obfuscation techniques underscores the challenge of achieving both simultaneously.
Advanced obfuscation methods like DeepBlur represent a shift from the more traditional approaches to image blurring. These newer techniques leverage machine learning to intelligently manipulate images, particularly focusing on hindering facial recognition systems while preserving overall image quality. Traditional methods, like pixelation and simple Gaussian blurring, often prioritize simplicity over adaptability. This simplicity, while seemingly straightforward, can lead to vulnerabilities. For instance, pixelation can leave behind patterns that AI-powered facial recognition can exploit, undermining their intended purpose.
The complexity of advanced techniques introduces a dynamic element that traditional methods lack. Instead of relying on static parameters, these advanced approaches use AI to analyze image features and generate tailored blur levels. This data-driven approach allows them to adapt to changes in recognition technology, potentially offering more resilient privacy protection in the long run. It's a noteworthy contrast compared to the rigid nature of traditional blurring methods.
Furthermore, advanced blurring focuses on maintaining the perceptual quality of images. Instead of simply blurring the entire image, they strategically target specific areas, like faces, preserving vital visual cues that aid in image understanding. This is vital in professional settings where the contextual information in an image remains crucial. Traditional methods, in their simplicity, often create overly-blurred or distorted images, diminishing their usability and potentially impacting their original purpose.
Advanced methods also offer a more adaptive approach based on context. DeepBlur, for example, incorporates the viewer's distance from the image to dynamically adjust the blur level, ensuring a more appropriate level of privacy in different scenarios. Traditional methods lack this flexibility, applying a uniform level of blur regardless of the viewing conditions.
However, this sophistication comes at a cost. Traditional techniques generally require less computational power, making them potentially faster to implement. Advanced methods need to use sophisticated algorithms, and their computational needs can be a barrier to real-time implementation, particularly in resource-constrained environments. Nevertheless, the developers of DeepBlur, and other advanced systems, are working to overcome this by optimizing their techniques for efficient processing.
One of the strengths of advanced techniques lies in their ability to address how humans perceive images. They strive to create blurring patterns that are less jarring and more natural looking, avoiding unnatural distortions that could draw unwanted attention. In contrast, traditional techniques often generate awkward or distracting blur patterns due to a lack of consideration for human perception psychology.
Moreover, advanced methods like DeepBlur can employ layered obfuscation techniques, providing more robust security. Traditional methods generally stick to a single approach, making them susceptible to emerging threats in facial recognition. It's worth considering if these layers of obfuscation create an appropriate level of privacy or if they introduce new ethical considerations related to information access.
In a world increasingly focused on privacy and the ethical use of data, advanced techniques strive to align with evolving privacy regulations and ethical frameworks. Traditional methods, with their less nuanced approach, may not address these critical concerns in the same way. We must be cautious when developing these solutions to make sure they do not unintentionally reduce the usefulness of images for their intended purposes.
Finally, these newer methods strive to minimize the cognitive load on viewers. By maintaining essential information, they aim to make images easier to understand. In contrast, the simplicity of some traditional techniques can result in loss of contextual information, forcing users to work harder to extract meaning from a distorted image. These are important considerations when choosing an appropriate obfuscation method, especially when accessibility is a factor.
Overall, advanced obfuscation techniques represent a significant improvement over traditional methods in various aspects. However, their complexity introduces new challenges, particularly regarding real-time application, computational efficiency, and ethical implications. As facial recognition continues to evolve, the continuous development and refinement of advanced techniques will be crucial for safeguarding individual privacy in an increasingly visual world.
Precision Blurring A Deep Dive into Selective Image Obfuscation Techniques for 2024 - Evaluating Robustness Against Deep Learning-Assisted Attacks
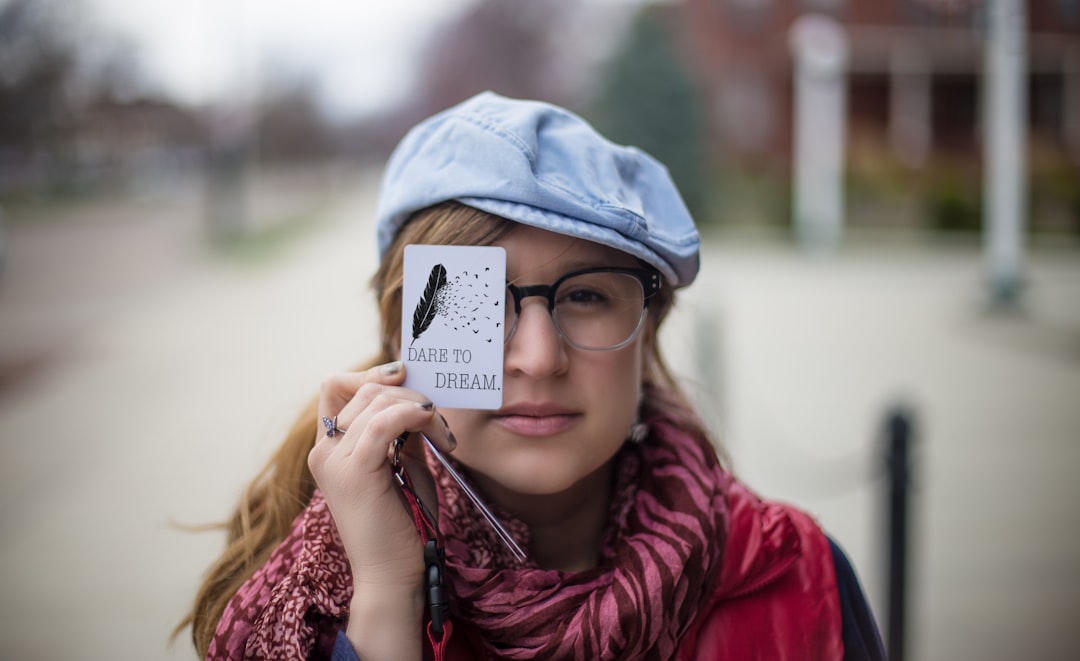
Within the field of image manipulation, evaluating the effectiveness of obfuscation techniques against attacks leveraging deep learning has become paramount. A new evaluation framework has emerged to assess the resilience of common methods like pixelation, blurring, and masking, particularly in safeguarding sensitive information such as faces within images. With the increasing sophistication of deep learning-based attacks, it's becoming clear that traditional obfuscation techniques aren't always adequate, often proving vulnerable to facial recognition systems. This necessitates a critical reevaluation of how we obscure sensitive information within images while maintaining both privacy and useful image quality. The tension between preserving privacy and maintaining image usability continues to be a challenging aspect in today's visually-driven world. A key aspect of developing robust obfuscation methods is a thorough understanding of the capabilities and goals of those who might try to circumvent these methods, as this knowledge is essential for creating defenses that can withstand the evolving threat landscape.
1. **Understanding Attack Strategies through Adversarial Training**: To really understand how well image obfuscation techniques hold up against deep learning-based attacks, we need to use adversarial training. This means purposely feeding the model misleading data during training to help it become more resilient against clever attack methods.
2. **Focusing on Weak Spots**: It turns out that certain layers within the neural networks that drive these recognition systems are more vulnerable to attacks. Knowing this allows researchers to create more focused defense mechanisms. Instead of a one-size-fits-all solution, we can tailor the defenses to the specific weaknesses found in different layers.
3. **The Complexities of Attacks**: When we look at image processing systems, the ways they can be attacked are surprisingly varied. Attacks can manipulate pixels, change metadata, and use other sneaky tricks. This wide range of attack possibilities makes it challenging to build a single, effective defense.
4. **The Speed Bump of Real-Time Processing**: When we evaluate how robust these image obfuscation systems are, we often find that real-time performance can be a bottleneck. For example, while DeepBlur does a great job of intelligently blurring images, it might struggle to keep up with fast and adaptive attack strategies in real-time scenarios. This highlights a need to refine these methods for speed and efficiency.
5. **The Surprising Impact of Attack Transfer**: One unexpected finding is that attacks designed for one neural network model can sometimes work on other similar models. This means that when we're evaluating the robustness of a system, we need to consider the broader ecosystem of models it interacts with. Looking at a single model in isolation doesn't give us the full picture of its weaknesses.
6. **Leveraging Context for Attacks**: Attackers are becoming more sophisticated. They often use context, such as the image's intended audience or its environment, to make their attacks more effective. This means we have to build robustness evaluations that include context as a factor. We can't just ignore the 'where and why' of an image when evaluating how secure it is.
7. **The Human Element in Attacks**: It's interesting that human perception can influence how well attacks work against image obfuscation techniques. For example, how a person interprets visual cues can affect the success of an attack. This emphasizes the importance of incorporating aspects of human psychology into the design and evaluation of these defense mechanisms.
8. **New Metrics for New Challenges**: Evaluating robustness requires new types of metrics that look at both the accuracy of the image recognition systems and the effectiveness of the image obfuscation techniques. Traditional metrics may not be able to capture the subtle effects of adversarial conditions, so new evaluation tools are needed to assess performance accurately in these more complex scenarios.
9. **The Ever-Evolving Landscape of Attacks**: Adversarial attacks are becoming more dynamic, adapting in real-time to the defenses they encounter. This constant evolution is a significant hurdle for researchers, who need to continually refine defenses to stay ahead of the curve.
10. **The Ethical Side of Robustness**: As techniques for evaluating robustness improve, there are ethical implications for areas like surveillance and privacy. When we strengthen systems against deep learning-based attacks, we have to make sure we don't inadvertently harm individual rights and freedoms. There's a fine line to walk, and we need to carefully consider these implications in our evaluation efforts.
Precision Blurring A Deep Dive into Selective Image Obfuscation Techniques for 2024 - Selective Blurring Techniques for Preserving Image Content
Selective blurring techniques are essential for safeguarding sensitive information while retaining the valuable content within an image. Newer methods like DeepBlur demonstrate how deep learning can be leveraged to create a more nuanced approach to blurring. Instead of simply applying a uniform blur, these techniques analyze the image and strategically apply blurring to specific areas, often those containing sensitive information like faces. This approach stands in contrast to older methods like Gaussian blur or pixelation, which often result in a noticeable degradation of image quality and can be relatively easily bypassed by advanced facial recognition technologies. By retaining important image features and the overall context of a scene, selective blurring offers a balance between maintaining visual appeal and enhancing privacy. This intelligent approach represents a potential leap forward in protecting privacy within our increasingly visual world, while mitigating the risks that come with traditional blurring methods that are becoming ineffective.
Selective blurring techniques represent a significant leap forward from the older, simpler ways of blurring images. DeepBlur, for example, demonstrates how concentrating the blur on specific areas within an image—like faces—can keep the image looking good while still making it harder for both humans and machines to identify individuals. It's an improvement over older techniques like just making the whole image blurry, which can often ruin the overall context.
One cool aspect is how these new methods can adapt to the viewer's situation. They can adjust how much blurring is applied based on how far someone is from the image. This allows for a more flexible approach to privacy compared to the fixed, one-size-fits-all blurring of older techniques. It's notable that blurring techniques can significantly confuse advanced facial recognition algorithms, making it challenging for them to pick out faces accurately. This is a stark contrast to methods like pixelation, which sometimes leave hints that can be exploited by these systems.
A key benefit is the layered defense aspect. You can use multiple blurring approaches, one after another, to make the image more secure against attacks that try to exploit specific weaknesses. Adversarial training is a strategy employed in developing these blurring techniques, forcing the algorithms to handle situations that might normally fool them. It's an effort to create more robust blurring methods.
Interestingly, these newer blurring techniques aren't just about algorithms; they also think about how humans perceive images. They aim to make the blurring less jarring and obvious to our eyes while still doing the job of protecting sensitive information. While improved blurring techniques offer great benefits, they still need to be optimized for speed. This is a challenge, especially if these techniques need to run in real-time, such as in video surveillance.
Simple pixelation and the older ways of blurring images often have problems. They create obvious patterns or artifacts that sophisticated image recognition systems can exploit. Researchers are creating new and better evaluation methods to test the security of these blurring approaches, making it easier to assess how effective they are.
We also need to carefully consider the ethical implications as selective blurring techniques get more advanced. There are questions around things like user consent and transparency, especially when images contain sensitive details. This raises concerns about how we use these blurring techniques ethically, making sure we don't inadvertently violate people's rights in the name of security.
It's clear that selective blurring is a promising area for image privacy. It improves upon the limitations of earlier methods and allows us to refine privacy solutions, but there are still aspects to work on and careful consideration to be given to the ethical implications of more powerful image manipulation techniques.
Precision Blurring A Deep Dive into Selective Image Obfuscation Techniques for 2024 - Future of Image Privacy in the Era of Advanced Facial Recognition
The future of image privacy in the age of advanced facial recognition presents a growing concern. While methods like pixelation and basic blurring were once sufficient, they now struggle against increasingly sophisticated facial recognition systems. This has led to the development of more advanced techniques, like DeepBlur, that use deep learning to intelligently blur specific image features—primarily faces—while preserving visual quality. These new approaches attempt to protect privacy without making images unusable. However, as these techniques progress, we face intricate ethical and practical questions surrounding privacy, image usability, and the broader implications for a visually-centric society. Finding a balance between the desire for privacy and the utility of images will require continued innovation and careful consideration of the consequences in our evolving digital world. The risks to privacy are amplified in a world that increasingly leverages visual data in public spaces and online, demanding a thoughtful and adaptable approach.
The landscape of image privacy is rapidly evolving, particularly with the increasing sophistication of facial recognition technologies. We're seeing a growing awareness of the potential threats to privacy posed by these systems, and this has led to a surge in regulations aimed at controlling their deployment in various sectors. Companies are being compelled to re-evaluate their practices and explore more privacy-focused approaches, including innovative image obfuscation methods like DeepBlur.
While facial recognition algorithms have become remarkably adept at identifying individuals, recent developments in selective blurring strategies offer a compelling counterpoint. Techniques like DeepBlur have emerged to address these advancements, strategically adjusting blurring intensity based on factors like proximity to the image and the surrounding environment. This adaptability is a major step forward compared to older, static methods.
Furthermore, the public’s attitude towards surveillance technologies is shifting. People are becoming more conscious of the privacy risks associated with constant visual monitoring and are increasingly demanding stronger protections for their personal identities. This growing awareness could pave the way for more widespread adoption of image obfuscation techniques.
Surprisingly, even facial recognition algorithms themselves are increasingly reliant on synthetic data for training. While this may seem beneficial for development, it also introduces ethical complexities and, perhaps counterintuitively, can make traditional privacy measures less effective. Synthetic faces can be engineered to evade conventional obfuscation methods, highlighting the urgent need for more sophisticated image blurring techniques.
Another interesting aspect of facial recognition algorithms is their susceptibility to targeted attacks. Each algorithm often exhibits specific vulnerabilities, suggesting that targeted obfuscation methods can be developed to counter these weaknesses. A better understanding of where these recognition systems struggle can lead to more targeted and effective image blurring strategies.
Simultaneously, the field of image retrieval is advancing, and when combined with facial recognition, it can inadvertently amplify the risk of privacy violations. These interconnected technologies highlight the importance of robust image obfuscation solutions to prevent unintentional data extraction while preserving the usability and functionality of images.
Interestingly, human perception plays a significant role in the success of image obfuscation techniques. Research suggests that the ways in which we process visual cues can impact how effectively a blurred image safeguards privacy. This emphasizes the importance of considering human psychology when designing obfuscation methods.
As image manipulation technologies continue to evolve, ethical concerns about user consent are becoming increasingly prominent. Individuals may not fully understand the implications of sharing images in our increasingly interconnected world and the potential for them to be manipulated. Greater transparency in the application of obfuscation techniques is therefore critical.
We're also becoming more aware of the cascading effects of privacy breaches. Compromised images can be used to connect to other personal information, widening the scope of a privacy violation. This makes it essential for obfuscation techniques to be designed not just to protect individual images but also to limit broader risks to identity and personal data.
Finally, the rising awareness of privacy risks has driven increased investment in privacy-enhancing technologies. Companies are increasingly looking for tools that balance high-quality image preservation with effective obfuscation, reflecting a major shift in consumer demand and market trends. These investments and the broader societal focus on privacy suggest that robust image obfuscation will play an increasingly vital role in shaping the future of image privacy in our visually-saturated world.
Colorize and Breathe Life into Old Black-and-White Photos (Get started for free)
More Posts from colorizethis.io: