Colorize and Breathe Life into Old Black-and-White Photos (Get started for free)
The Evolution of AI Image Generation A Look at Emerging Techniques in 2024
The Evolution of AI Image Generation A Look at Emerging Techniques in 2024 - Multimodal AI Models Revolutionize Image Generation
The field of AI image generation is experiencing a significant shift in 2024, largely driven by the emergence of multimodal AI models. These models represent a leap forward, going beyond the limitations of single-data-type systems by combining various information sources like text, code, and images. We see examples of this in models such as GPT-4 and DALL-E, which showcase how the blending of these different modalities can spark innovation in artistic and creative endeavors. Furthermore, AI's ability to understand and interact with the world through images is broadening, as seen in projects like the Multimodal Open Language Model (Molmo). This model demonstrates the potential for AI to not just interpret visuals, but also participate in interactive tasks, expanding the realm of AI's capabilities.
As we see more integration of multimodal features into mainstream products and tools, understanding how to effectively manage and synthesize the diverse information becomes paramount. This necessitates a sophisticated approach to the fusion and processing of multimodal data to ensure the accuracy and quality of the resulting images. This is particularly evident in advancements like conditional neural radiance fields (NeRFs), which demonstrate the benefits of using multimodal data to achieve highly realistic and detailed 3D-aware image generation. In essence, the integration of multiple data types within image generation is no longer just a novel approach; it is becoming the defining characteristic of future progress in this field, promising new and exciting avenues for both artistic expression and technical achievement.
Multimodal AI models are pushing the boundaries of image generation by incorporating different types of data, like text and images, to understand context more deeply. This approach goes beyond the traditional methods that relied on a single type of input, resulting in a more sophisticated understanding of the generation process.
Researchers are increasingly focusing on techniques like cross-attention, which allows these models to pinpoint specific parts of both the image and text inputs, enhancing their ability to create extremely accurate representations. This approach is showing promise in generating images that are more culturally and contextually relevant, something that single-modality models often struggle with due to their limited understanding.
A new trend in multimodal image generation is the use of reinforcement learning to refine the model's behavior as it creates. This allows the models to align their outputs better with user needs and preferences, offering more control over the generated imagery. We're also seeing these models tackle abstract concepts and metaphors expressed in language, producing images from descriptions that would confuse more traditional AI systems.
The challenge of training these complex multimodal models has sparked a wave of innovations in computing hardware. Researchers are exploring specialized processors to accelerate the training process, making it more feasible to work with these complex systems. However, we need to be mindful of the potential for biases in the generated images. Since these models learn from the data they're trained on, it's important to continuously monitor their outputs to ensure fairness and accuracy.
The applications for this technology are diverse, extending beyond art and advertising. We could see multimodal AI employed in product design to visualize early concepts or even in interactive environments where users can modify images with simple text prompts. This ability to make changes with simple instructions holds the potential to greatly streamline creative workflows. Furthermore, the field is exploring incorporating time-based data into these multimodal systems, potentially leading to images that evolve and change dynamically, adding a new dimension of depth and narrative to visual content.
The Evolution of AI Image Generation A Look at Emerging Techniques in 2024 - Neural Network Advancements Push Boundaries of Realism
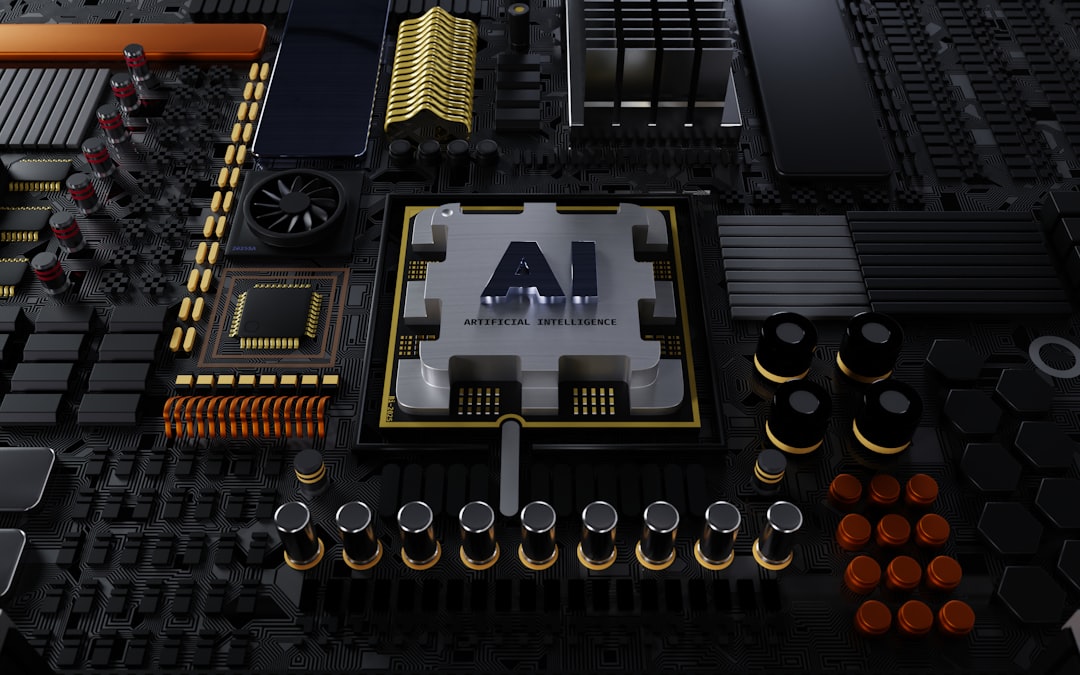
The evolution of AI image generation in 2024 is strongly tied to significant advancements in neural networks. These advancements are driving a new wave of realism and detail in generated images. Techniques like Neural Radiance Fields (NeRF) and 3D Gaussian Splatting are improving the creation of both 2D and 3D images, allowing for a level of fidelity that was previously unattainable. Convolutional neural networks are also playing a key role, enabling a smoother transition from vector data into complex, visually rich 2D images. The increasing interplay between 2D and 3D methods is indicative of a growing sophistication in generative AI models, which in turn allows for more dynamic and intricate visuals. However, as AI image generation continues to progress, it's crucial to acknowledge the accompanying ethical and governance concerns that need careful consideration. The potential for misuse and the need for responsible development remain central challenges that must be addressed as the technology matures.
The landscape of AI image generation is being reshaped by ongoing advancements in neural networks. We're seeing a surge in the creation of incredibly lifelike images, almost indistinguishable from real photographs, thanks in part to the development of techniques like Generative Adversarial Networks (GANs). This has sparked legitimate discussions about the reliability of visual information in our increasingly digital world.
Diffusion models have emerged as a new and powerful approach to image synthesis. These models begin with random noise and gradually refine it into highly detailed pictures, a departure from earlier approaches that relied on direct image sampling. This iterative refinement process demonstrates an impressive degree of control over image generation.
Excitingly, the efficiency of neural network architectures is improving, enabling the generation of higher-resolution images with fewer computational resources. This increased accessibility to advanced image generation tools has the potential to democratize the process, although it also raises concerns regarding potential misuse.
Beyond static images, researchers are exploring neural networks that can understand and process sequences of images, a step towards more dynamic applications in video creation and animation. This capacity could potentially revolutionize how we experience visual storytelling in various media.
The field has also made strides in fortifying neural networks against attempts at manipulation and distortion through adversarial training methods. This is increasingly important as concerns over misinformation in AI-generated content continue to rise.
Furthermore, incorporating real-time feedback mechanisms into the image generation process empowers neural networks to adapt to user preferences and refine output dynamically. This is pushing image creation towards a more personalized and interactive experience.
The ability of neural networks to generate 3D models directly from 2D images is an intriguing development. It breaks down the traditional boundaries between 2D and 3D content creation, opening up new possibilities for fields such as gaming, filmmaking, and virtual reality.
Building upon this, the integration of neural networks with optical flow techniques allows them to generate dynamic and realistic moving images, a notable advancement over previous static image generation systems.
The applications of neural networks are expanding beyond visual art and entertainment. We see them being used to visualize intricate biological processes in medicine or to simulate surgical outcomes. This showcases their remarkable adaptability and potential to tackle complex problems across diverse fields.
Finally, exploration into computational creativity using neural networks is generating unique artworks that emulate specific art movements or styles. This has sparked debate about the definition of authorship and originality in the context of AI-generated content, raising fascinating questions about human creativity and its interaction with artificial intelligence.
The Evolution of AI Image Generation A Look at Emerging Techniques in 2024 - Ethical Considerations Shape Development Practices
The rapid evolution of AI image generation in 2024 has brought ethical considerations to the forefront. Developers and researchers are increasingly mindful of the potential societal impact of these powerful technologies, recognizing the need to build ethical considerations into every step of the development process, from data collection to system deployment. This includes a growing awareness of the potential for bias within generated images, a consequence of the data these systems are trained on. Questions of fairness, transparency, and the potential for misuse are now central to the discussion.
Globally, organizations are establishing guidelines and frameworks aimed at responsible AI development, reflecting a growing consensus on the need for ethical oversight. This focus on ethics is vital for fostering trust and ensuring that the benefits of AI image generation are widely shared and used responsibly. The goal is to leverage the remarkable advances in image generation while mitigating potential harms, guaranteeing a future where these technologies contribute positively to society.
The field of AI image generation is not only advancing technologically but also sparking increasingly complex ethical debates. Researchers and creators are grappling with establishing clear boundaries for what constitutes appropriate content, especially when it comes to sensitive topics and the risk of spreading misinformation. One emerging concern is "data poisoning," where training datasets are deliberately manipulated to produce skewed or harmful results. This underscores the vital role of ensuring data integrity throughout the AI development process.
Studies have highlighted biases in AI-generated images, frequently perpetuating existing societal stereotypes. This points to a critical need for diversity in the training data to help foster impartiality in AI outputs. A related notion gaining prominence is "algorithmic accountability." This concept pushes engineers to develop mechanisms that trace AI-generated content back to its source data, leading to more transparency and allowing for easier audits of the processes.
The widespread use of AI for image generation in both personal and professional settings is raising a critical question about ownership and intellectual property rights. Generating images based on existing works creates a blurred line between inspiration and infringement. To address this, researchers are exploring the potential of real-time ethical feedback systems. These systems would allow the AI models themselves to evaluate whether their outputs align with community norms and ethical principles during the image creation process, fostering a greater sense of ethical responsibility in the use of AI.
Further, there's a growing emphasis on the "explainability" of AI-generated images. Developers are realizing that it's not sufficient to simply generate outputs. They need to also provide clear explanations of how those outputs were derived, promoting user trust and confidence in the technology. The potential for misuse of AI generated images, like the creation of deepfakes, has led to calls for stronger regulatory frameworks. The goal is to mitigate the risks of identity theft and defamation while maintaining a balance between innovation and public safety.
Research also suggests that even seemingly unbiased training data can unintentionally reflect cultural biases. This emphasizes the need for a more meticulous and nuanced approach to data selection and preparation for model training, if we're to uphold ethical standards in image generation. As the technology matures, concerns about user consent are surfacing. The idea that individuals whose likeness appears in generated images should have a voice in how those images are utilized and portrayed is pushing the field towards a more ethically responsible engagement with the creative process. This complex interplay of technical progress and societal impact underscores the growing need for robust ethical frameworks that guide the development and application of AI image generation technology.
The Evolution of AI Image Generation A Look at Emerging Techniques in 2024 - User-Friendly Interfaces Democratize AI Art Creation
The year 2024 is seeing a shift in AI art creation, marked by the rise of user-friendly interfaces. These intuitive tools are making it easier for anyone, regardless of their technical background, to explore and create AI-generated art. This broader access to creative tools fosters a wider range of perspectives and artistic styles, enriching the overall creative landscape. The simplification of complex processes allows artists to concentrate more on the core concepts and ideas behind their work, rather than getting bogged down in technical hurdles. While this democratization of art offers incredible opportunities, it also raises valid questions about the nature of art itself. The growing presence of AI-generated pieces within traditional art contexts challenges established notions of creativity, originality, and artistic authorship. As this technology continues to evolve, it's crucial to consider the implications of AI's influence on the art world and how human and artificial creativity can coexist and interact.
The emergence of user-friendly interfaces for AI art tools has significantly broadened the pool of individuals capable of generating compelling artwork. This accessibility is fostering a more inclusive artistic landscape, allowing individuals without specialized technical training to participate in the creative process. We see that intuitively designed interfaces are enabling a wider range of users to engage with these powerful systems and contribute fresh artistic perspectives.
This trend towards simplification aligns well with research in cognitive science, which shows that intuitive interfaces can boost engagement and creative output. By abstracting away the complexity of the underlying AI algorithms, these interfaces enable users to focus on their artistic vision rather than getting bogged down in technical details.
Interestingly, the rise of low-code or no-code platforms for AI art has had a notable impact on the creative workforce. Artists and designers who may not have traditionally been involved with coding are finding themselves increasingly able to utilize these tools. This convergence of traditional art forms with technology is leading to new types of collaboration and opportunities at the intersection of art and technology.
Many of these user-friendly tools rely on sophisticated algorithms that utilize reinforcement learning. These algorithms dynamically adapt to user preferences over time, leading to a more personalized creative experience where the AI system effectively learns an individual's unique style. This adaptability provides a level of customization previously unseen in art generation tools.
Furthermore, these interfaces serve as a valuable source of data about how users interact with the technology and what types of images they prefer. This data, which reveals user preferences and behavior, can be used to refine the algorithms that power these interfaces and ultimately improve the AI's ability to understand and anticipate user needs in future iterations.
The proliferation of user-centric AI art platforms has stimulated research into human-computer interaction, particularly in the realm of creativity and artistic expression. This research is crucial for understanding how users perceive authorship and creativity when interacting with AI, which in turn is reshaping the discussion around intellectual property and ownership in the context of AI-generated art.
However, as the generation process becomes more streamlined and accessible, it also raises important questions regarding the ability to distinguish between human-created and machine-generated images. This ambiguity has brought to light ongoing conversations about authenticity and the core values associated with artistic creation.
Researchers are finding that these user-friendly tools can sometimes contribute to what can be called "creative overload." With such a wide array of options and styles available at their fingertips, some users find it challenging to make choices and execute their creative vision, which can impact their output.
The implications of user-friendly AI interfaces extend beyond the art world, influencing education and training in creative fields. Students are now exposed to new models of creative practice that blend traditional skills with the possibilities of advanced technology.
As user-friendly AI art interfaces continue to evolve, they are also serving as a critical testing ground for ethical considerations within AI. The nature of these tools, which allow for direct user interaction with sophisticated systems, can readily reveal potential biases embedded within AI systems. This can heighten awareness of the social implications of AI-generated content, further solidifying the importance of ethical considerations in the development and deployment of these tools.
The Evolution of AI Image Generation A Look at Emerging Techniques in 2024 - Integration of AI-Generated Images in Creative Industries
The integration of AI-generated images is reshaping creative industries by altering traditional processes and augmenting human creativity, not replacing it. We're seeing major platforms like YouTube integrate AI into their creative tools, fostering a growing use of AI in art, music creation, and writing. The ability of AI to make creativity accessible to more people allows for a broader range of artistic voices and ideas within the art world, but also sparks crucial conversations about who owns the creative work and what it truly means to be original. However, this rapidly changing technology brings complications, highlighting the need for well-thought-out ethical frameworks and guidelines to manage issues like potential biases within the generated content and questions about copyright and ownership. As these AI tools continue their rapid development in 2024, we're forced to grapple with the relationship between human and AI creativity and the evolving nature of artistic expression itself.
The integration of AI-generated images is reshaping various creative industries. For instance, fashion designers are utilizing AI to experiment with different styles and visualize garments before they're physically produced. This can streamline the design process and potentially minimize the costs of creating physical samples.
Similarly, the gaming industry is increasingly relying on AI-generated imagery for things like character design and environmental art. This allows for faster design iterations and richer, more complex game environments, ultimately contributing to a more immersive player experience.
The advertising industry is seeing a surge in demand for AI-generated visuals. Marketers are using AI to craft targeted campaigns for specific demographics, enhancing the effectiveness of advertising by better engaging consumers.
Architectural visualization is also benefiting from this trend, with architects using AI to rapidly create both 2D and 3D representations of their designs. This helps facilitate communication with clients who can more readily grasp the designer's intent.
Researchers are even investigating the use of AI-generated images in medicine. One potential application is in creating detailed simulations of anatomical structures for surgical training or patient education, expanding the horizons of medical imaging.
Journalism is another field where AI-generated images are being explored. They could be utilized to create compelling visuals for articles, particularly when real-world images aren't easily available. This opens the door to presenting hypothetical scenarios or visualizing historical events in a more tangible way.
The role of art curators is also evolving with the rise of generative AI art. Curators are now incorporating AI-generated pieces into traditional art spaces, leading to interesting discussions about new forms of curation and how we understand creativity within a digital environment.
Furthermore, AI-generated images are finding their way into education. They offer an innovative approach to teaching a variety of subjects, providing engaging visuals that can enhance understanding and perhaps bridge gaps in traditional teaching methods.
The ability to create hyper-realistic images is prompting industries like film and advertising to explore the concept of 'virtual talent.' AI could be used to generate stunning visuals without needing human actors, which might lead to changes in how we perceive talent and performances.
However, the widespread use of AI-generated images raises concerns about their impact on our perception of reality. There are ongoing conversations regarding the potential for misinformation and the creation of misleading visuals that could influence public opinion and cultural narratives. It's a complex area that requires ongoing critical thought and responsible development practices.
The Evolution of AI Image Generation A Look at Emerging Techniques in 2024 - Emerging Techniques Address Current Model Limitations
The field of AI image generation in 2024 is actively addressing limitations found in earlier models, introducing novel techniques to improve their capabilities. A notable development is the rise of diffusion models, which start with random noise and progressively refine it into detailed images, greatly accelerating and enhancing the generation process compared to earlier methods. This approach, coupled with the incorporation of multiple data types, is helping AI systems generate images with richer contextual understanding, moving beyond the constraints of single-modality input. Furthermore, significant research efforts are directed towards bolstering the reasoning abilities of these models. This pursuit of enhanced cognitive functions reflects a desire to build AI systems that are not just image generators but also possess a deeper grasp of the concepts behind the images they produce. These improvements suggest a broader shift towards more sophisticated AI methodologies for image generation, leading to outputs that better align with human artistic expectations and creative visions.
### Emerging Techniques Address Current Model Limitations
The field of AI image generation is facing and overcoming challenges associated with traditional models. While we've seen tremendous progress with current models, there's a growing focus on refining and expanding their abilities. One notable area of research is cross-modal learning. It's fascinating how researchers are exploring methods that link different types of data—like text and visuals—to enhance the image creation process. This interweaving of information sources leads to a deeper grasp of cultural contexts within images, something that was previously difficult for single-modality systems.
Another intriguing development is the rise of adaptive neural networks. These are networks that can alter their structure during training based on the specific data they're processing. This dynamic ability represents a shift from fixed, static models, potentially leading to more efficient and specialized image generation for different tasks. And the integration of temporal data into the generation process is quite exciting. This means the possibility of AI-created images that evolve and change over time, opening doors to animation and visual storytelling that responds to user input. However, we need to keep in mind that these innovative techniques also introduce challenges and complexities.
The concept of generative feedback loops is also gathering momentum. It's like a chain reaction where the output of one model becomes the input for another, improving the refinement process. It's a collaborative method where multiple models work together to produce high-quality images, but the underlying complexities of these interwoven systems pose considerable challenges.
Furthermore, new model architectures are being developed that are specifically designed to improve the contextual awareness of the generated images. By incorporating vast quantities of contextual data during training, these systems are capable of generating images that are more aligned with cultural nuances and the situations depicted, ultimately refining the user experience.
It's crucial to recognize that training data often carries biases, and researchers are tackling this challenge with fairness-aware modeling. This approach aims to incorporate diverse perspectives and identities into the training datasets and algorithms, helping to eliminate biases in the outputs and ensuring equitable representation in AI-generated images.
Meanwhile, user-centric design principles are being implemented to ensure that even individuals with no technical background can engage with these systems. Simplified interfaces make image creation more accessible, democratizing the creative process and fostering greater participation.
Building on this, researchers are leveraging advanced machine learning techniques to create AI models capable of real-time adaptation. These systems can modify their outputs in response to immediate feedback, enhancing interactivity and enabling more responsive, customized image creation.
AI-generated images often encompass incredible levels of detail, and new approaches are being implemented to effectively handle this complexity. Hierarchical modeling is one approach where various levels of detail are handled independently during the image synthesis process. This means we can create highly complex images without needing tremendous computing resources.
Finally, the ability for AI systems to use user behavior data from past interactions to predict future preferences is noteworthy. This learned behavior allows the system to tailor future outputs, fostering an even more personalized and engaging user experience.
However, it's critical to remember that as this technology advances, we need to keep considering the ethical implications. While these emerging techniques show remarkable promise, careful and continued oversight is needed to ensure that they're developed and used responsibly.
Colorize and Breathe Life into Old Black-and-White Photos (Get started for free)
More Posts from colorizethis.io: