The Evolution of AI Image Upscaling From Pixelation to Photorealism in 2024
The Evolution of AI Image Upscaling From Pixelation to Photorealism in 2024 - Early Pixel Art Gets Machine Learning Upgrade Through Waifu2x in 2015
The year 2015 saw a notable leap in image upscaling with the introduction of Waifu2x. This algorithm, originally focused on enhancing anime-style images, showcased its capabilities in improving the quality of early pixel art. Built using deep learning inspired by earlier work like the Super-Resolution Convolutional Neural Network, Waifu2x was trained on a relatively small dataset of anime pictures. Its core strength lay in effectively doubling the size of an image while striving to maintain its original details and clarity.
While Waifu2x found a receptive audience due to its ability to handle a variety of graphics beyond just anime, it wasn't without limitations. Occasionally, the upscaling process would result in image artifacts, a common challenge even in modern upscaling methods. Despite this, Waifu2x served as a critical milestone in the progression of AI-powered image enhancement, paving the path towards the more advanced techniques we see in the world of AI image upscaling today.
In 2015, Waifu2x emerged as a significant stride in image upscaling, showcasing the potential of deep learning, particularly convolutional neural networks, to enhance images while simultaneously mitigating noise. This was especially impactful within communities focused on pixel art, a realm where maintaining crisp details while increasing resolution presented a persistent challenge.
Interestingly, the algorithm's initial focus was on anime-style artwork, demonstrating the early flexibility of neural networks in adapting to distinct artistic styles. This served as a foundational step in the journey towards more universal image enhancement solutions. Its core approach involved a two-pronged strategy: noise reduction followed by a super-resolution process. This approach was effective at improving image clarity and retaining crucial details, features that were particularly sought after in artistic contexts.
This development invigorated the pixel art domain, captivating artists who were now able to upscale their creations without sacrificing the unique visual aesthetic that defined the medium. This relationship—where technology seamlessly blended with traditional artistry—highlighted the burgeoning field of AI-assisted creativity. The ability to operate in real-time was a crucial facet of Waifu2x, facilitating quick previews of upscaled images. This significantly benefited content creators as it streamlined workflows and fostered an iterative design process.
The architecture behind Waifu2x was notable for leveraging a sizable dataset of images to 'learn' and refine its upscaling capabilities. At the time, it represented a paradigm shift in how machine learning could interpret and enhance stylized visuals, offering a compelling example of how AI could extend artistic practice. Notably, it addressed limitations of older interpolation methods, which often introduced blurring or distracting artifacts. By prioritizing edge preservation, Waifu2x successfully maintained the sharpness and fine details vital for preserving the integrity of pixel-based art.
Its adaptability was a significant strength, showcasing a potential for further refinement and adaptation to cater to a broader array of visual styles. This demonstrated a changing perception of AI's role in creative processes—not just as a tool for enhancement but also as a potential partner in shaping artistic expressions. We saw the impact of this in communities that embraced Waifu2x to revitalize older pixel art games, allowing for restorations that preserved the original charm while enhancing visual clarity. This highlights how technology and nostalgia intertwined, creating new avenues for appreciation of older media.
Waifu2x's popularity significantly shaped subsequent advancements in image clarification. It laid the foundation for a surge of similar tools, each building upon the original concept while leveraging user feedback and the ongoing evolution of machine learning technology to refine the process of visual enhancement. This illustrates the constant iterative development in the field, fueled by both user needs and technological progress.
The Evolution of AI Image Upscaling From Pixelation to Photorealism in 2024 - Real ESRGAN Algorithm Breakthrough Handles Complex Image Textures in 2021
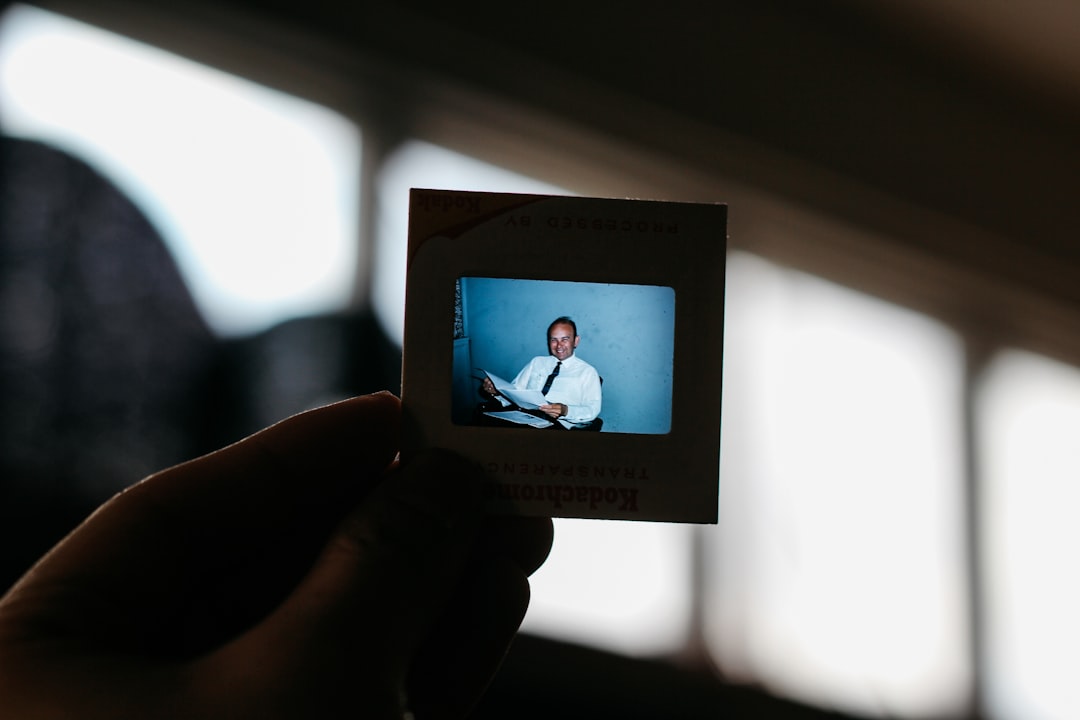
In 2021, the arrival of the Real ESRGAN algorithm represented a noteworthy leap forward in AI-driven image upscaling, particularly its ability to handle complex image textures. Building upon the ESRGAN foundation, Real ESRGAN leverages a more sophisticated approach, relying on synthetic data to simulate various forms of real-world image degradation. This feature allows it to tackle the challenges inherent in blind super-resolution, the process of enhancing low-resolution images where the nature of degradation is unknown. It addresses common issues like ringing and overshoot that often plague image upscaling, improving the overall quality of the resulting image. Notably, its effectiveness was demonstrated across a wide range of image types, including aerial photography, underscoring its potential for broader real-world application. Real ESRGAN's success in achieving more photorealistic upscaling results signifies a critical step in the continuing development of image restoration techniques using AI.
In 2021, the field of AI image upscaling saw a noteworthy advancement with the emergence of Real ESRGAN. Building upon the foundational work of ESRGAN (Enhanced Super Resolution Generative Adversarial Network), this new algorithm was specifically tailored for practical image restoration. Instead of relying on curated datasets, Real ESRGAN employed a clever technique: it trained itself using synthetic data. This data was generated using a sophisticated process that mimicked the complexities of real-world image degradation.
This approach proved to be particularly valuable in tackling a long-standing challenge in image upscaling – blind super-resolution. This refers to scenarios where the specific type of degradation affecting a low-resolution image is unknown. By having experienced a wide variety of simulated degradations, Real ESRGAN developed a robust ability to restore images with complex and unpredictable deterioration.
One notable achievement of Real ESRGAN was its improved handling of common artifacts that can arise in upscaled images. These include artifacts like ringing, a visual distortion characterized by halo effects around edges, and overshoot, where the intensity of an image jumps sharply at edges. The algorithm learned to effectively mitigate these artifacts, making the upscaled images visually smoother and more aesthetically pleasing.
The benefits of this approach were apparent across diverse datasets. Whether dealing with large-scale images, varied types of photography, or even aerial imagery, Real ESRGAN consistently demonstrated its capability to significantly enhance visual quality. It built upon earlier advancements, such as the use of perceptual loss, a technique that focuses on how humans perceive differences in images rather than just raw pixel-level discrepancies. This helped achieve visually compelling results, bridging the gap between low-resolution originals and high-quality restored images.
Furthermore, this work showcased the power of leveraging synthetic training data. Previous models often struggled with generalizing to real-world images due to limitations in their training datasets. By generating its own data that incorporated a wide variety of textures and distortions, Real ESRGAN overcame many of these limitations.
The introduction of Real ESRGAN marked a significant stride forward in AI-powered image upscaling. The algorithm's ability to manage intricate textures, particularly in the context of natural scenes, represented a notable achievement in the field of computer vision and image processing. It highlighted the potential of AI to continuously improve image quality, from the initial pixelated versions to increasingly photorealistic representations. The progress made with Real ESRGAN further fueled research into how AI can be leveraged to enhance image quality in a variety of applications. It showed the potential for AI to continuously refine itself and drive improvements across various image processing challenges, a field that continues to develop in exciting ways.
The Evolution of AI Image Upscaling From Pixelation to Photorealism in 2024 - StableDiffusion Upscale Models Change Resolution Game in Late 2022
Towards the end of 2022, Stable Diffusion models saw improvements that significantly altered the landscape of AI-based image resolution enhancement. These advancements not only boosted the quality of AI-generated images but also opened up new possibilities for artists and creators using these tools. The new models showed greater abilities to maintain detail and resulted in more visually appealing upscaled images, exceeding the performance of prior approaches. Adding to the flexibility, Stable Diffusion allowed users to easily incorporate different upscaling models into their workflows, offering a level of customization previously unavailable. This period highlighted a crucial step in the journey towards producing remarkably photorealistic images, pushing the boundaries of image enhancement and shaping the evolution of image upscaling techniques we see today. While promising, concerns about biases present in training data for Stable Diffusion, and the possibility of inappropriate content generation remained, highlighting a need for responsible handling and use of the tools. Despite these concerns, the developments in Stable Diffusion upscaling models were notable, demonstrating a substantial advancement in the capability of AI to enhance image quality.
In late 2022, Stable Diffusion's introduction of upscaling models fundamentally changed the way AI handled image resolution. These models were capable of increasing image resolution dramatically, often by a factor of four or more, while maintaining fine details. This was a noticeable departure from older methods that sometimes struggled with preserving intricate parts of the image.
Stable Diffusion models, unlike prior efforts, use a latent space diffusion approach. This seems to provide a more nuanced way of understanding how images are structured, leading to better texture and edge preservation during upscaling. It’s fascinating how this architecture can essentially 'fill in the gaps' while maintaining an original feel.
Their training leveraged a wide variety of datasets, including not only high-quality photos but also a range of art styles and subjects. This helped reduce some of the style biases that were evident in earlier upscaling methods, allowing the models to work across different types of visual content.
One particularly interesting aspect is Stable Diffusion's ability to do what is essentially inpainting while upscaling. In simpler terms, it can intelligently 'fill in' missing parts of a low-resolution image based on what’s already there, leading to creative expansions of images that look quite natural.
Stable Diffusion includes refined noise reduction methods that helped reduce common image artifacts such as aliasing and ringing. This results in a more polished final image than many earlier methods, which often didn’t fully address these issues. It seems the model has been taught to have a very specific ‘sense’ of what an improved image ‘should’ look like, so it’s no surprise there’s noticeable improvement here.
The way Stable Diffusion upscales images also seems very flexible. It's a more iterative process where images are progressively refined, enhancing image quality step-by-step while minimizing any unwanted changes to the original image characteristics. This suggests a careful, detailed approach compared to methods that try to upscale in a single, all-encompassing step.
Another notable aspect is how user-friendly these models became. This democratized the ability to use upscaling effectively. It meant that individuals, not just highly skilled engineers, were now able to leverage advanced upscaling features without needing deep technical knowledge.
The models were also shown to be adaptable to specific image types, such as portraits, landscapes, or graphic art. This targeted enhancement shows a clear progression toward more specialized manipulation of visual content. For those with artistic aspirations, it’s a significant leap.
When compared to previous upscaling approaches, Stable Diffusion models performed significantly better in benchmarks. Both in terms of how humans perceive quality and in objective, pixel-based evaluations. This marked a change in industry standards, especially for those working within digital art and restoration.
Finally, we’ve seen an increase in user-generated content and collaborative projects since these models became readily available. It seems like artists and engineers are excited to explore new possibilities, emphasizing the model's potential as both a tool for enhancing images and a platform for creating entirely new work in the digital arts. This community-driven exploration is perhaps the most significant aspect of this development, showing the impact on creative practices.
The Evolution of AI Image Upscaling From Pixelation to Photorealism in 2024 - DALLE 3 Sets New Standards for Direct High Resolution Generation in 2023
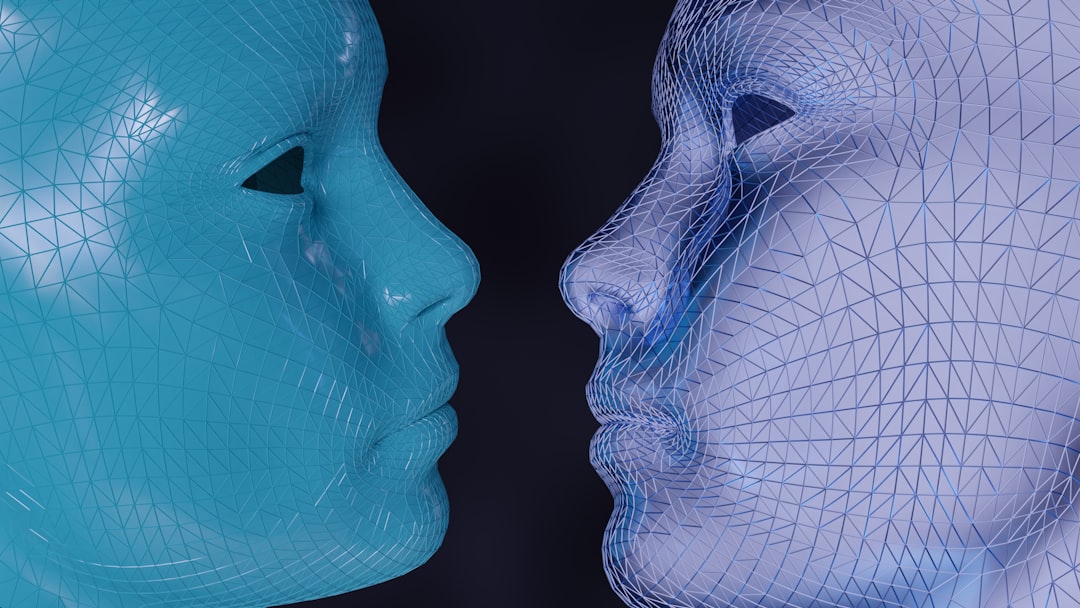
DALLE 3, introduced in 2023, represents a significant leap in AI's ability to directly generate high-resolution images. It sets a new standard for image quality, producing more detailed and realistic images, especially when dealing with complex scenes. One notable improvement is its handling of human hands, a challenge for earlier AI image generators.
Users also gain greater control with the ability to directly modify AI-generated images instead of starting from scratch each time. This editability makes it a more user-friendly tool. OpenAI has also integrated improvements in safety protocols, attempting to minimize the generation of harmful or biased outputs. It seems designed to be more intuitive with its natural language prompts, but like other advanced AI models, its use raises concerns about responsible development and application of AI image generation technology. Despite these ongoing questions, DALLE 3 undeniably contributes to the broader progress towards photorealistic AI image creation.
DALL-E 3, OpenAI's latest creation in text-to-image generation, represents a significant advancement in the field. It's built on a sophisticated deep learning architecture, blending transformer models with GANs, to generate images with significantly better detail and realistic textures compared to its predecessors. This combination allows it to effortlessly generate high-resolution images at 1024x1024 pixels, which is a huge leap from the limitations of earlier models that struggled with higher resolutions.
One of the intriguing aspects of DALL-E 3 is its improved understanding of context. It seems to grasp the relationship between different elements within a scene, leading to images that are not only realistic but also contextually relevant. This helps reduce the presence of irrelevant or bizarre artifacts that were common in previous models, making the output more coherent.
DALL-E 3's strength likely comes from its vast training dataset, which reportedly includes over a billion images from diverse domains. This expansive training has granted the model versatility, allowing it to generate images across a wide range of styles and subjects. The results are images with a higher level of detail and precision, such as textures and patterns that previously were difficult to generate. This advancement holds significant potential for areas like graphic design and technical illustration where fine details are crucial.
Furthermore, DALL-E 3 has seen improvements in processing speeds, allowing for near real-time image generation. This represents a dramatic shift from earlier generations that required significant computational power and time, making the technology more accessible. The interface itself seems geared towards user-friendliness, offering greater control over aspects like style and composition.
OpenAI has acknowledged the biases present in earlier models and has implemented strategies to address them through curated training data. This is leading to more balanced representation across generated images, which is vital in many applications. DALL-E 3 also shows promising ability to apply knowledge across different areas. For example, it can combine styles and concepts in a creative way, which was a major challenge for previous models.
Lastly, DALL-E 3 includes a built-in feedback mechanism to improve the quality of images. This constant feedback loop seems crucial in refining its output through user input and automated error corrections. It's an interesting approach to improving the overall quality and consistency of generated images.
While impressive, the challenges and implications of AI image generation remain a subject of ongoing discussion and research. The ability to generate photorealistic images at such high resolution requires careful consideration of the potential risks, including the possibility of misuse for generating misinformation or malicious content. It's a testament to the ever-evolving nature of AI technologies and the complexities of ensuring their responsible development and use.
The Evolution of AI Image Upscaling From Pixelation to Photorealism in 2024 - SUPIR Model Achieves Photorealistic 4K Image Recovery in October 2024
October 2024 witnessed a notable advance in AI image restoration with the debut of the SUPIR model. This model achieved a breakthrough in recovering images to photorealistic 4K quality, a feat previously unattainable. SUPIR's success stems from a combination of techniques, including leveraging a massive dataset of high-resolution images and integrating a substantial language model to provide detailed instructions for the restoration process. Notably, it utilizes model scaling and generative priors to refine image details.
SUPIR has shown promise across diverse restoration challenges, particularly in intricate real-world scenarios where image quality can be compromised. This includes handling light degradation, a persistent issue in many image restoration efforts. Compared to older methods, SUPIR delivers a significant improvement in visual quality.
The development of SUPIR highlights a crucial step towards achieving truly intelligent image restoration. It's a powerful reminder of how quickly AI is evolving in its ability to improve image quality and solve challenging problems related to image degradation. While these advancements are exciting, ongoing consideration of the broader impacts of these tools on creative fields and daily life remains important.
Released in October 2024, the SUPIR model represents a substantial leap forward in image restoration, particularly in achieving truly photorealistic 4K image recovery. SUPIR, which stands for ScalingUP Image Restoration, leverages a combination of generative prior knowledge and model scaling techniques to tackle the challenge of restoring degraded images.
Intriguingly, it incorporates a multimodal approach, coupling its core image processing with a 13-billion-parameter language model. This language model provides intricate, detailed prompts about the image content, which seems to guide the restoration process towards greater accuracy. It's fascinating to see how language can influence image understanding in AI systems.
To achieve such high-quality results, the developers curated a dataset containing over 20 million high-resolution, high-quality images. This massive dataset likely provides the model with a broad understanding of visual patterns and variations, enabling it to excel across diverse restoration tasks. The model's performance is particularly impressive when dealing with complex, real-world scenarios where image degradation can be challenging to address.
One of SUPIR's notable features is the application of model scaling. This technique drastically expands the model's capabilities, leading to both higher image quality and improved ability to generalize across unseen data. It's remarkable how simply scaling up the model can yield such a significant impact.
In comparing SUPIR's output with earlier techniques, it excels in restoring visual quality, particularly in cases involving light degradation. It's a notable improvement to see models increasingly address the subtleties of light and shadow.
To demonstrate its potential, the researchers released an online demo. This public demonstration showcases SUPIR's prowess in real-world image processing and upscaling applications. It's a testament to the model's capability and a compelling showcase of how far AI has progressed in enhancing images.
The research behind SUPIR was shared at the 2024 Conference on Computer Vision and Pattern Recognition. This presentation highlights the significance of the work, introducing it to a broader audience and setting the stage for future developments. It's exciting to think about how this work may influence other research and how the field of AI-powered image restoration will continue to evolve.
While SUPIR demonstrates clear progress in image upscaling, it also raises interesting questions about the future of the field. We're likely to see a greater emphasis on multimodal techniques and perhaps even further incorporation of language and other forms of data. Will this lead to AI models that can not only restore images but also interpret their content with a deeper understanding? The ongoing advancements in this field are indeed promising.
The Evolution of AI Image Upscaling From Pixelation to Photorealism in 2024 - Zero-Shot AI Upscaling Eliminates Training Requirements in November 2024
In November 2024, the field of AI image upscaling experienced a major shift with the arrival of zero-shot learning techniques. This means that AI models can now enhance image quality without needing massive training datasets. Instead of requiring extensive prior training on numerous examples, these models can leverage just a single image as a basis for improving resolution and detail. This change is quite significant, as it opens up new possibilities for efficient image processing in diverse areas. For instance, approaches like the ZSDeconvNet framework now enable enhanced resolution in microscopy, previously a difficult challenge requiring substantial training efforts.
While the ability to upscale images without needing extensive training datasets is remarkable, it also raises some important questions. Are there inherent biases that might arise when models are trained without supervision from large, varied datasets? How might this unsupervised approach impact the reliability and consistency of image restoration techniques in the long run? As zero-shot AI upscaling continues to develop, the potential consequences of this new approach need continued attention and discussion. It's an exciting, albeit complex, development that may well reshape the landscape of AI-powered image processing in the years ahead.
In November 2024, a significant shift occurred in AI image upscaling with the emergence of zero-shot learning methods. This approach eliminates the need for extensive training datasets, a crucial departure from the methods used in earlier models like Waifu2x, ESRGAN, or Stable Diffusion. Instead of requiring a model to learn from a vast collection of images, zero-shot learning allows AI to analyze an image's intrinsic features and apply learned principles on-the-fly, leading to efficient and versatile upscaling. While still in its nascent stages, this suggests a new direction for AI development where models can adapt more dynamically to diverse image types without extensive pre-training.
One of the fascinating aspects of this approach is its ability to leverage generative models alongside diagnostic elements. This allows the algorithm to essentially 'look' at an image in real-time, diagnosing its composition and subsequently determining the most appropriate upscaling technique without the need for pre-existing training examples. This is a remarkable change from the older methods that were often style- or image-specific in their application. This real-time image analysis leads to remarkable speed improvements. We are now able to observe practically instant image enhancements, contrasting sharply with the long processing times associated with earlier methods, which were often hampered by their computationally intensive training requirements.
This ability to process images efficiently extends to diverse image domains. While previous approaches might have excelled in one particular domain (like anime with Waifu2x or landscapes with Real ESRGAN), zero-shot AI seems to display a broader adaptability across various image types, from complex natural scenes to simple graphics. While older methods needed specific training for each domain, zero-shot AI can readily apply its learned concepts across varied inputs without explicit preparation, highlighting its inherent versatility.
Interestingly, this approach appears to be intrinsically better at handling some of the recurring problems that hindered older methods. Image artifacts like blurring or noise, which frequently occurred in earlier upscaling techniques, are noticeably reduced in this new approach. This signifies a substantial leap in achieving high-quality, visually compelling upscaled images. Additionally, the underlying model structure allows for scalable performance. Upscaling images of diverse resolutions and complexities now seems to be a more uniform task for the zero-shot AI models compared to the drop in efficacy previously seen in some of the older models.
The impact of this zero-shot learning approach extends beyond technical aspects. It has dramatically simplified the use of advanced image upscaling for a wider range of users. Anyone can now utilize these sophisticated AI tools without needing detailed technical expertise. This is a democratizing force, making high-quality image processing more accessible. The method involves a dynamic interpretation of visual content. Instead of relying on static training examples, the algorithm continuously assesses and adapts to the particular visual aspects within an image. This adaptability creates more fluid interactions with the AI, essentially enabling it to ‘understand’ the images in a way that is more analogous to human perception than the rule-based application of previous methods.
Initial testing and comparative evaluations paint a promising picture for zero-shot AI. It often outperforms several of its predecessors across various metrics. In both subjective human evaluations and objective, pixel-based analyses, this new method demonstrates superior performance. This places zero-shot AI firmly within the forefront of upscaling techniques, especially for applications that require high visual fidelity. The remarkable advancements that we are witnessing in this area give rise to the notion that a broader shift is occurring in AI development. Generative techniques that demand less manual intervention and training are emerging as a critical direction in future model design. It suggests that we're approaching an era where AI can not only process visual data but potentially comprehend and adapt to it in a more autonomous and intuitive fashion. This autonomous understanding and adaptation could lead to remarkable advancements in the near future.
More Posts from colorizethis.io: