The Evolution of Text-to-Image AI A Comparative Analysis of 2022 vs 2024 Models
The Evolution of Text-to-Image AI A Comparative Analysis of 2022 vs 2024 Models - Improved Visual Realism in 2024 Models
The leap in visual fidelity achieved by text-to-image AI models in 2024 is undeniable, surpassing the quality seen in 2022 iterations. Models like OpenAI's DALLE 3 and Google's Imagen2 demonstrate this progression through their capacity to generate images with a high degree of photorealism and a better adherence to user instructions. While impressive, concerns about accessibility persist. Many of these advanced models are built upon proprietary datasets and lack transparency about their inner workings, hindering wider adoption. Furthermore, areas like culturally sensitive image generation and advanced image manipulation techniques, seen in Meta AI's work, suggest that the pursuit of realism needs to be paired with broader considerations. The evolution of these models in 2024 represents a crucial step in the development of AI-generated visuals. They have the potential to significantly refine how we use imagery in storytelling and content creation, thanks to improved realism and a greater connection to user intent. However, responsible development and deployment of these powerful tools are crucial to ensure that benefits are accessible to all and not confined by restrictive data practices.
The visual fidelity of AI-generated images has seen a remarkable leap forward in the 2024 models compared to their 2022 counterparts. We're seeing a greater attention to detail in the synthesis of textures. For instance, materials like skin, fabrics, and metals are now rendered with a level of surface complexity that better matches their real-world counterparts. This is achieved through the use of more refined algorithms.
Further, the handling of light has become more sophisticated. The ability to realistically simulate lighting conditions, including shadows and highlights, is noticeably improved. This comes from new methods that factor in environmental elements to enhance the depth and three-dimensionality of the images.
Additionally, AI's portrayal of human faces has become much more expressive. Leveraging advancements in facial recognition, the models can now capture a broader range of nuanced emotions, giving AI-generated figures a more authentic feel.
The adoption of HDR rendering techniques has also been a major contributor to this surge in visual realism. This enables a wider dynamic range of light and color, ensuring that both bright highlights and dark areas maintain detail, further bridging the gap between computer-generated and photographic imagery. However, achieving a truly photographic look remains challenging, as the subtle complexities of real-world lighting continue to pose a difficulty.
It's not just about the individual elements, but how they are arranged in a scene. Improved scene understanding allows the models to produce more coherent and meaningful image compositions. The AI is now better at considering spatial relationships between elements, resulting in more balanced and visually appealing layouts. This includes the handling of material interactions, where the rendering of reflective surfaces like glass and water is becoming increasingly accurate.
Interestingly, there's a growing focus on using color theory principles in image generation. This ensures that the color palettes used not only align with real-world observations but also contribute to the overall harmony of the image. This can lead to a more aesthetically pleasing output compared to past models.
The concept of depth is also better realized. New approaches in depth mapping create a more convincing three-dimensional space, lending a sense of volume and tangible presence to the generated objects. This improves the overall sense of immersion for the viewer.
One notable trend is the increasing adaptability of the models themselves. Users can now often tailor the style of the images through prompts, which allows them to seamlessly navigate between a photorealistic and a more artistic or stylized approach to generation. It will be interesting to see how far this adaptability will be pushed in future models.
Finally, in the realm of animation, a major challenge has been to maintain consistent visual realism across a sequence of frames. Significant improvements in temporal consistency suggest that the hurdle of seamlessly blending together AI-generated images into a cohesive, believable animation is being gradually overcome. This has clear implications for the future of AI in storytelling and the creation of animated content.
The Evolution of Text-to-Image AI A Comparative Analysis of 2022 vs 2024 Models - Advancements in Text-Image Alignment
The progress made in aligning text and image outputs has been a driving force behind the evolution of text-to-image AI, especially between 2022 and 2024. One significant area of advancement has been in handling longer, more complex text prompts. Older methods, like those used by CLIP, were sometimes inadequate, leading to the development of techniques like LongAlign, which better processes segmented parts of lengthy prompts.
This push for improved alignment is also seen in models like Playground v3 (PGv3), which leverages deeper learning methodologies and attention mechanisms. These newer models strive to build a strong connection between the text input and the resulting image. This pursuit of semantic consistency has become crucial. It allows AI to not just produce an image based on keywords, but to generate visuals that are richer in meaning and more reflective of the intended narrative conveyed through the prompt.
We see a greater emphasis on building AI models that can truly understand and represent the text's meaning visually. As these models grow more sophisticated, their ability to craft engaging visuals that precisely capture the user's intentions becomes increasingly evident. It appears that the future of text-to-image AI relies on this continued focus on enhancing the connection between language and visuals.
The field of text-to-image (T2I) synthesis has witnessed remarkable advancements in 2024, particularly concerning how well the generated image matches the textual description. Older approaches, often relying on CLIP for encoding text, started showing limitations when dealing with longer or more nuanced prompts. To tackle this, researchers have developed methods like LongAlign, which breaks down longer prompts into segments for better processing. This is a key area as it shows a shift towards more complex and detailed instructions that users can provide.
Several new models have emerged, each contributing to the improvement of T2I capabilities. We've seen models like IIRNet, CRDCGAN, GALIP, and others exploring techniques to boost image quality, diversity, semantic understanding, and object detail. The prominence of Playground v3 (PGv3) stands out, achieving impressive results across benchmarks and utilizing Large Language Models for better prompt understanding, a significant change from the reliance on pre-trained encoders in previous iterations.
This drive towards richer T2I capabilities has led to increased use of deep learning. These models have become more sophisticated in interpreting textual descriptions and translating them into highly realistic images. A core concept is the control of the denoising process in latent diffusion models. Text prompts act as guiding forces, ensuring image generation stays on track with the intended meaning, highlighting the vital role of text-image alignment in the overall quality.
Measuring the effectiveness of these models has also improved. Metrics like TIAM provide frameworks to assess how well the generated images reflect the text prompt. This is critical as it provides researchers a quantitative way to track progress and compare different models.
Furthermore, AI's capacity in image-text matching tasks has increased thanks to the evolution of foundation models. This ability allows models to identify and link text elements to corresponding visual elements with greater accuracy. It's become evident that the methods used to generate T2I outputs are becoming more sophisticated. Attention mechanisms and innovative architectures have enabled models to finely tune the visuals based on complex text inputs.
Ultimately, the push towards more intricate and engaging visual experiences in an increasingly image-driven world is driving the development of these T2I models. Researchers are constantly exploring new approaches, pushing the boundaries of what's achievable through this fascinating intersection of artificial intelligence and human creativity. There's still a long way to go before AI can perfectly represent the richness and complexity of human expression in image form, but the rapid pace of developments suggests a future where the gap between text and image will shrink considerably.
The Evolution of Text-to-Image AI A Comparative Analysis of 2022 vs 2024 Models - The Rise of Diffusion Models in Image Generation
Diffusion models have emerged as a dominant force in image generation, distinguished by their ability to produce high-quality images through a gradual refining process. Models like Imagen and DALL-E 2 demonstrate the effectiveness of this approach, successfully translating intricate text descriptions into visually rich images. The progress from 2022 to 2024 is characterized by a noticeable leap in image quality and a stronger connection between the generated image and the user's text prompt. This signifies a more profound understanding of the nuances of language within the models themselves.
While diffusion models have shown great promise, they are not without limitations. The demands of training these models are substantial, and their computational requirements are significant. This aspect raises important questions about the future accessibility and scalability of this technology. As diffusion models continue to evolve, their potential to influence visual storytelling becomes increasingly evident. It's critical to consider the broader implications of this power, particularly ensuring a balance between innovation and accessibility for a wider audience.
Diffusion models have quickly become a prominent force in image generation, captivating researchers with their novel approach. Unlike the adversarial training methods used in GANs, diffusion models refine random noise into well-defined images through a series of denoising steps. This methodical process offers a greater degree of control over the creative process, which is one of the reasons for their increasing adoption.
A key aspect driving their rise is their ability to represent intricate data distributions more accurately, leading to remarkably high-quality image output. The resulting images frequently exhibit a photorealism exceeding what was achievable with earlier generative models, which is a substantial improvement.
Excitingly, recent work has shown that, with enhanced model architectures and optimization strategies, diffusion models can achieve near real-time generation speeds. This is a significant leap forward, considering previous versions often demanded substantial computational resources for comparable outcomes.
Intriguingly, these models also demonstrate a stronger ability to handle unusual or noisy text prompts, preserving the quality of the generated image even when presented with atypical input. This robustness is particularly beneficial for real-world applications where unexpected text prompts are common.
Further improvements like classifier-free guidance have broadened the scope of diffusion models, giving users the ability to guide the generation process towards desired styles or content without relying on pre-labeled data. This makes interacting with the models simpler and more intuitive.
Another intriguing facet of these models is their effectiveness in tasks like image inpainting and editing. They can effectively fill in missing parts of an image while preserving its underlying meaning, making them valuable for various creative fields.
Comparisons against GANs have revealed diffusion models to be superior in synthesizing detailed textures, particularly for natural scenes. This is potentially groundbreaking for industries like gaming and film, where convincingly rendered environments are paramount.
Researchers are actively investigating the scaling capabilities of diffusion models, specifically multi-scale training methods, to improve image resolution without sacrificing detail. Such advancements could prove instrumental in high-resolution content creation.
Emerging insights into the underpinning diffusion processes show that manipulating the noise schedule can fine-tune elements like stability and detail of generated images. This provides a promising direction for future investigations and refinements of these methods.
Finally, the simplification of certain aspects of the diffusion process has facilitated the successful integration of these models into diverse applications. From augmented reality experiences to personalized content production, diffusion models show immense potential to redefine how we interact with and produce digital media. This holds exciting prospects for the future evolution of how we design and use the digital world around us.
The Evolution of Text-to-Image AI A Comparative Analysis of 2022 vs 2024 Models - Expanded Diversity in Generated Content
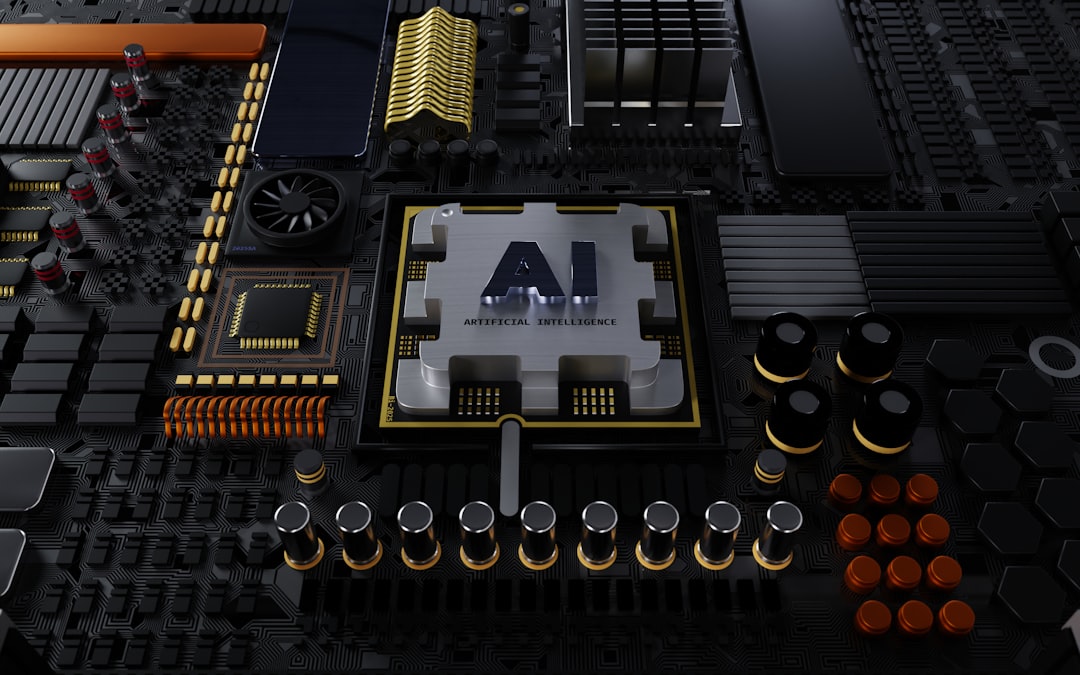
The evolution of text-to-image AI from 2022 to 2024 has brought a welcome emphasis on expanded diversity within generated content. No longer limited to addressing just gender and ethnicity, models are now incorporating a wider range of attributes into the images they produce. Techniques like Diverse Diffusion highlight this shift, striving to foster a more inclusive and varied visual landscape. This means images can explore a more extensive spectrum of colors, textures, and styles, potentially enriching the artistic possibilities of AI-generated content.
Despite the impressive strides in realism, concerns regarding originality and the potential for repetition across different contexts persist. While AI can now mimic real-world visuals with exceptional accuracy, some models still exhibit a tendency to fall back on similar patterns, raising questions about the extent to which they truly capture unique perspectives. The pursuit of greater diversity in image generation acknowledges the importance of offering a wider variety of creative possibilities, ensuring that AI-generated art and visuals are more representative of the richness and diversity of human experience. The direction towards a more inclusive and representative visual output signals a growing understanding of the vital role that diverse content plays in creative expression.
The Evolution of Text-to-Image AI A Comparative Analysis of 2022 vs 2024 Models - Introduction of HEIM Benchmark for Model Evaluation
The development of the HEIM benchmark, or Holistic Evaluation of Text-to-Image Models, introduces a more thorough approach to evaluating the capabilities and potential issues of text-to-image AI. While earlier evaluations tended to focus mainly on how well the image matches the text prompt and the quality of the image, HEIM takes a broader perspective. It considers a range of 12 factors, including the realism and aesthetics of the image, as well as concerns like bias, fairness, and the model's robustness. This wider perspective is crucial because it allows us to better understand the varied ways these models might be used and the impacts they could have.
The benchmark was created in response to the remarkable progress in the field of text-to-image generation. It's clear that there's a growing need to understand these models quantitatively, beyond just anecdotal evidence. HEIM provides a consistent way to compare and contrast a wide variety of recent text-to-image models (26 were assessed initially). By standardizing the evaluation process, researchers and practitioners can more effectively compare and contrast the strengths and weaknesses of different approaches to text-to-image generation.
It's anticipated that HEIM will influence future development within the field. By pinpointing areas where current models struggle, the benchmark could push research towards mitigating bias, improving fairness, and addressing potential problems before they become widespread issues in real-world applications. Overall, HEIM represents a step forward in understanding and evaluating this rapidly advancing technology. It's a move towards the more careful and responsible development and use of text-to-image AI.
The HEIM (Holistic Evaluation of Text-to-Image Models) benchmark has emerged as a new tool to thoroughly assess the capabilities and potential risks of text-to-image AI models. Previous evaluation methods primarily focused on the match between text prompts and generated images, along with general image quality. HEIM expands upon this, taking a more holistic view that encompasses 12 key areas. These include aspects like image quality, realism, creativity, adherence to the prompt, reasoning abilities, knowledge integration, potential biases, and even the models' efficiency.
It's fascinating that HEIM arose from the rapid improvements in text-to-image models and a growing need for a robust, quantitative way to understand their strengths and weaknesses. The increasing adoption of text-to-image AI across various applications, like content creation and even scientific visualization, has underlined the need for such a benchmark. It offers a standardized way to compare models and helps to identify areas where they excel or struggle.
One particularly interesting element of HEIM is its inclusion of human assessment alongside objective metrics. This hybrid approach tries to address the fact that simply achieving high scores on certain metrics doesn't necessarily mean the model is successful in conveying meaning or creating emotionally resonant outputs. In essence, it brings a more subjective element to a traditionally objective process.
The benchmark's use of a diverse range of datasets is quite compelling. It challenges models by pushing them to handle different content, styles, and cultural contexts. This broad scope is important, as it exposes weaknesses in models that might otherwise go unnoticed in more narrow tests. HEIM emphasizes the importance of "descriptive richness," which focuses on whether generated images accurately and comprehensively reflect intricate details and contextual elements from the input text. This is a departure from simply generating images based on individual keywords.
Additionally, HEIM also brings attention to how models might inadvertently reproduce biases present in their training data, specifically highlighting biases across demographics. This emphasizes the importance of actively working to mitigate bias in training datasets to foster more equitable visual representation in AI models.
Moreover, HEIM incorporates elements of evaluating the emotional responses generated images elicit from humans. This signifies a shift towards understanding how text-to-image models affect viewers on an emotional level, essentially evaluating their storytelling capabilities. It's remarkable how this framework is also being applied to assess the coherence and consistency of animations across multiple frames, a crucial consideration for future applications of text-to-image AI in areas like film or animation.
It's rather intriguing that HEIM reveals that high performance in objective evaluation metrics isn't necessarily a predictor of meaningful visual communication. This has generated a lot of discussion on whether AI can truly capture the complexity of human intent and expression through images.
Furthermore, the need for ethical guidelines and accountability for AI outputs has become a focal point of research in this area. HEIM has prompted important conversations on how generated images can reinforce existing biases and stereotypes, particularly concerning different communities. This heightened attention to the societal impact of these models is a proactive step towards developing and implementing them responsibly.
In the future, it's likely HEIM will influence how researchers assess the overall quality of text-to-image models, particularly when it comes to designing visually rich content that fosters a sense of empathy and connection with viewers. There's a real chance that HEIM could set the foundation for new standards in AI evaluation, ensuring models move beyond simply technical proficiency and develop a greater understanding of the social and emotional context in which images are created and consumed. This is a significant step in fostering a future of AI-generated content that is not only impressive visually, but also responsible and insightful.
The Evolution of Text-to-Image AI A Comparative Analysis of 2022 vs 2024 Models - Integration of Multimodal Approaches in 2024
The landscape of text-to-image AI has seen a substantial shift in 2024 with the increasing prominence of multimodal approaches. We are witnessing a move towards AI systems that can handle multiple data types simultaneously, leading to more sophisticated and nuanced results. This means that models can now not only generate images from text but also better understand and integrate visual elements within the generative process itself.
This integration is driven largely by the development of Multimodal Large Language Models (MLLMs). These models are designed to process and generate outputs that are intertwined with both text and images. The result is a more holistic AI experience, where text prompts can now generate outputs that are far more attuned to the user's intent. Models like Gemini, which are built from the ground up to be multimodal, are demonstrating the power of this approach. They can be trained on a wide range of data formats, such as text, images, audio, and video. This allows for a higher level of performance across various generative tasks.
While the rise of multimodal models is incredibly promising, it does present new hurdles. Scaling these models to incorporate a wider array of data types poses significant technical challenges. Researchers are wrestling with how to efficiently integrate different forms of information and ensure that these models are not just powerful but also safe and fair. Concerns surrounding bias in AI-generated visuals and the need for responsible deployment continue to be a vital part of the conversation in this field. It's critical to consider the broader societal implications of these tools, as they continue to grow more potent and versatile.
The landscape of text-to-image AI has been significantly altered by the integration of multimodal approaches in 2024. We're seeing a movement away from solely text-based systems towards a more integrated understanding of text and images. This integration is driven by the development of frameworks that simultaneously process both modalities during training, leading to more efficient learning and a stronger link between the linguistic and visual aspects of data. This has resulted in a notable improvement in generating images that closely adhere to even the most intricate instructions embedded in prompts.
One of the exciting developments in multimodal machine learning (MMML) has been the emergence of real-time adaptation capabilities within models. Users can now modify the generated image in real-time based on immediate feedback they provide, creating a much more interactive experience during content creation.
Furthermore, there's a clear trend of applying insights from cross-modal perception research within these models. This research explores how humans naturally connect text and images, and AI models are now leveraging this knowledge to create more intuitive and contextually relevant images. They’re beginning to generate images that align better with the way our minds interpret text and visuals, showing a greater awareness of the nuances in human cognition.
Another key change is the noticeable diversification of the datasets used to train these models. They now incorporate a broader range of cultural contexts and stylistic variations, which reduces the risk of producing visually homogeneous outputs. This allows for richer explorations of visual expression and helps ensure that AI-generated content isn't limited to specific styles or perspectives.
We're also witnessing an increase in the integration of contextual metadata into the input process, such as user preferences or specific environmental factors. This is leading to a greater degree of personalization in image generation. AI models are tailoring outputs to better match the intended narrative by considering a wider array of input factors.
There’s also a shift toward generating images that are emotionally resonant, with models showing an increased capacity to recognize and mirror the emotional tone of a given text prompt. This capacity to "understand" emotion and translate it into visual language is crucial in making AI-generated images more relatable and engaging for viewers.
Moreover, the ability to process multiple types of inputs simultaneously, such as text, images, and even audio, is a growing capability of these multimodal models. This is allowing for the generation of content that's richer in context and detail, offering more immersive experiences for users, particularly in storytelling contexts.
However, the advancement of multimodal approaches hasn't been without its challenges. Concerns over bias in training data still persist, but in response, some models are implementing automated bias correction mechanisms. These mechanisms try to adjust the output in real-time if the model detects potential bias within the generated image. While still a work in progress, it represents a conscious step toward ethical development.
Additionally, these models are demonstrating a greater ability to translate different visual styles onto generated images. This enhanced control over the stylistic aspects of outputs is crucial for applications where visual consistency and a specific aesthetic are important. Areas like fashion or design stand to greatly benefit from this capability.
Finally, we’re starting to see a rise in collaborative multimodal tools. These tools enable multiple users to work together, contributing to the image generation process from diverse viewpoints. This opens doors to novel creative opportunities and fosters a more communal approach to digital content creation.
The development of these advanced multimodal models represents a critical step forward in the evolution of text-to-image AI. It's clear that as AI's ability to process and understand diverse inputs improves, its potential to seamlessly integrate across different modalities will continue to grow, leading to further exciting breakthroughs in the realm of digital content generation.
More Posts from colorizethis.io: