Advancements in AI Image Generation A Deep Dive into Text-to-Image Models in 2024
Advancements in AI Image Generation A Deep Dive into Text-to-Image Models in 2024 - Imagen's Frozen T5XXL Encoder Revolutionizes Text Embedding
Imagen's approach to text embedding stands out with its use of a frozen T5XXL encoder, a massive 46 billion parameter model. This large, pre-trained language model is instrumental in translating text prompts into highly informative representations, which are then fed into the image generation process. By freezing the encoder, Imagen avoids retraining this massive component, making the entire system more efficient while benefiting from the encoder's rich linguistic understanding.
The effectiveness of this method surpasses that of previous multimodal embedding strategies, like CLIP, as it establishes a stronger connection between the text and the generated visuals. Coupled with Imagen's core conditional diffusion process, this leads to the creation of detailed, high-resolution images. Further refinements, specifically the addition of text-conditional super-resolution models, allow for even higher quality output. This innovative combination positions Imagen as a leader within the expanding field of text-to-image AI. Though competing systems, like DALL-E and Parti, are also generating impressive results, Imagen's specific architectural choices point towards a promising future for this particular branch of generative AI.
Google's Imagen leverages a frozen T5XXL encoder, a massive language model with 46 billion parameters, to translate text prompts into a compact, yet semantically rich, representation. This encoder effectively condenses complex textual information into numerical embeddings, allowing the model to focus on the core meaning of the text while minimizing data redundancy. It's intriguing how it manages to pack so much information into a smaller space, paving the way for potentially more efficient image generation.
The use of a multi-head attention mechanism within the T5XXL encoder provides Imagen with a unique ability to dissect text, recognizing subtle nuances in language and context. This capability allows the model to generate images that reflect a more detailed understanding of the prompt, tackling complexities that were difficult for prior models. It's like the model can pay attention to different parts of the text simultaneously, capturing a broader perspective of the user's intent.
Furthermore, the T5XXL encoder can handle longer text sequences than its predecessors. This means Imagen can generate images based on extended descriptions, leading to more sophisticated and detailed outputs. It's a step toward richer narratives within images, potentially allowing for more detailed and creative storytelling.
It's notable that the T5XXL encoder seems to have a knack for adapting its embedding strategy based on the text's genre. Whether dealing with informal dialogue, formal poetry, or technical documentation, it can adjust its processing to ensure optimal performance. This adaptive nature is quite remarkable and allows Imagen to handle a wider variety of prompts compared to previous methods, enhancing its versatility.
Another intriguing aspect is that this encoder displays a degree of zero-shot learning, showing ability to handle previously unseen prompts with relative success. This suggests that it not only learns patterns from training data but also possesses a certain degree of generalized understanding of language and context, a promising area for further investigation.
This approach utilizes a transformer architecture, but it relies on the novel "frozen" parameter strategy, which enables faster adaptation to new tasks. Compared to the traditional method requiring substantial retraining, this technique suggests a much more efficient way for fine-tuning the model without massive computational overheads.
It’s encouraging to see that this encoder has mechanisms incorporated to help mitigate biases within the image generation process. Addressing potential bias is crucial for building fairer and more inclusive systems.
The resource efficiency of the training process is also noteworthy. Imagen appears to demand considerably fewer resources compared to other models with similar capabilities, challenging conventional wisdom in the field. This increased efficiency could have profound implications for democratizing access to powerful image generation models.
By using a wide range of training data, the encoder has fostered an ability to generate images that respect various artistic styles and themes, showing potential for enhancing the way machines understand and replicate human creative expressions.
Finally, initial assessments suggest that Imagen often produces images with more nuanced details and higher fidelity than some competitors. This aligns with the expectation that better text representation leads to higher-quality image synthesis, hinting at advancements in the representation capabilities of neural networks.
Advancements in AI Image Generation A Deep Dive into Text-to-Image Models in 2024 - Diffusion Models Drive Photorealistic Image Creation from Text
Diffusion models have emerged as a powerful technique driving the creation of photorealistic images from text descriptions. Imagen, a prime example of this approach, demonstrates a remarkable ability to translate complex text into visually stunning images. Its success hinges on a sophisticated process that incorporates conditional diffusion and innovative super-resolution methods. These techniques enable the model to produce images with a level of detail and fidelity that rivals traditional photography. Notably, Imagen achieves impressive results in established evaluation metrics like the Frechet Inception Distance (FID), further solidifying the model's strengths in image quality and realism. The development of standardized benchmarks, like DrawBench, is crucial for objectively evaluating the strengths and weaknesses of different text-to-image models, fostering ongoing improvements in the field. Through these efforts, the interplay between natural language understanding and image synthesis is becoming increasingly sophisticated, raising the prospect that AI-generated imagery will continue to evolve and potentially match the quality of human artistic endeavors. There are still limitations to be addressed, and a critical approach is needed to guide this technology's evolution.
Imagen, developed by Google, stands out among text-to-image models due to its ability to produce remarkably photorealistic images while exhibiting a strong grasp of language. Its foundation lies in a sophisticated diffusion process, where noise is progressively refined into a final image. This technique, combined with a large frozen T5-XXL text encoder, forms the backbone of Imagen's capabilities.
The T5-XXL encoder plays a pivotal role in translating text into meaningful numerical representations, or embeddings. This allows the model to capture the core essence of the text prompt, feeding it into the image generation process. Intriguingly, Imagen bypasses the need to retrain this colossal encoder, making the training process more efficient. This decision also allows Imagen to benefit from the already well-established linguistic understanding baked into the frozen encoder.
Imagen's image generation process involves two stages: a primary diffusion model that creates a low-resolution image (64x64 pixels), followed by a series of text-conditional super-resolution models that enhance it. This hierarchical approach is likely inspired by how humans often perceive and process information in stages. It's an elegant approach and seems to contribute to the overall quality of the resulting images.
The results of Imagen are impressive, with human reviewers frequently deeming the model's outputs comparable to real photographs from the COCO dataset—even though Imagen wasn't trained on that particular dataset. Its performance, as measured by the FID score, is amongst the best in the field. The DrawBench benchmark serves as a useful tool for comparing Imagen's performance to its competitors. In direct comparisons against VQGAN-CLIP, Latent Diffusion Models, GLIDE, and DALL-E 2, Imagen received consistently higher ratings from human evaluators.
Furthermore, Imagen's design reveals valuable insights into how large language models can significantly enhance image generation. The innovative architectural choices in Imagen—particularly the use of the frozen T5-XXL encoder and the hierarchical approach to image synthesis—emphasize the crucial interplay between text comprehension and visual rendering. It suggests that achieving photorealism and detailed image generation requires not only advanced image generation techniques but also a deep understanding of natural language.
The advancements seen in Imagen are a powerful testament to the continued progress in AI-driven image generation and foreshadows a future where the connection between language and visuals becomes even more seamless and sophisticated. The way Imagen deftly navigates the complex relationship between language and visuals hints at a new era where AI can create images that truly capture the nuances of human language and artistic intent.
Advancements in AI Image Generation A Deep Dive into Text-to-Image Models in 2024 - Google's ImageFX Enters the Text-to-Image Arena in February 2024
Google's entry into the text-to-image arena in February 2024 with ImageFX marked a notable development. ImageFX leverages the strengths of Google's Imagen 2, allowing users to translate text prompts into visuals. This approach broadens creative possibilities by making image creation more accessible. A key component of ImageFX is its "expressive chips," aiming to make the image creation process more intuitive. Google is also incorporating Imagen 3, which is intended to bring further improvements to the generated images, including enhanced photorealism and more accurate representations of the input text. The developers are also focused on reducing visual imperfections often found in AI-generated images. Furthermore, ImageFX provides IPTC metadata with each image, offering insight into the AI's role in creating the image. This release demonstrates Google's increasing interest in generative AI and its potential uses across various creative fields. While competing systems from OpenAI and Meta exist, ImageFX shows Google's ambitions in this evolving technology landscape.
In February 2024, Google unveiled ImageFX, their foray into the text-to-image generation landscape. Built upon their Imagen 2 foundation, ImageFX aims to provide users with a readily accessible way to produce images based on simple text prompts, encouraging more creative exploration. It’s intriguing how they've integrated Imagen 2's capabilities into a more user-friendly interface.
ImageFX distinguishes itself with a unique element called "expressive chips," suggesting a possible pathway for users to control stylistic aspects within the generated image. However, the practical effectiveness of these 'chips' remains to be seen.
The development doesn't stop there. Google is pushing the boundaries further by incorporating Imagen 3, a model designed to bolster image realism, add intricate details, and ensure a closer correspondence between the text prompt and generated image. This evolution is quite expected, and other teams are likely pursuing similar goals in their models.
Reducing visual artifacts is another area of focus for ImageFX. The generated images often suffer from unintended features, and reducing these anomalies is a key challenge for all researchers. A cleaner image, regardless of how realistic or stylistically fitting it is, may be a critical element for adoption.
The AI image generation domain has seen rapid advancement recently, with platforms like OpenAI's DALL-E 3 and Meta's Imagine pushing the field forward. ImageFX appears to be a concerted attempt to bring a Google-branded, easy-to-use product to the forefront.
Interestingly, ImageFX includes IPTC metadata alongside each generated image. This metadata allows viewers to easily track the AI-assisted creation process, perhaps laying the groundwork for better understanding of the technology's capabilities and limitations.
Google didn't stop at ImageFX; they also unveiled MusicFX and TextFX, suggesting a broader strategy in developing AI tools across various creative media. This coordinated launch implies a long-term vision for AI's role in content generation.
ImageFX fits into Google's wider push to integrate AI into diverse creative applications. It remains to be seen whether their approach will be more accessible or more beneficial to users in the long run. While it offers intriguing features and aligns with ongoing trends in the field, the practical utility and broader impact on the field remain to be evaluated. The AI art scene is a lively one, and it will be compelling to see how ImageFX holds up to the competition.
Advancements in AI Image Generation A Deep Dive into Text-to-Image Models in 2024 - DALL-E 2 Pushes Boundaries with Advanced Image Manipulation
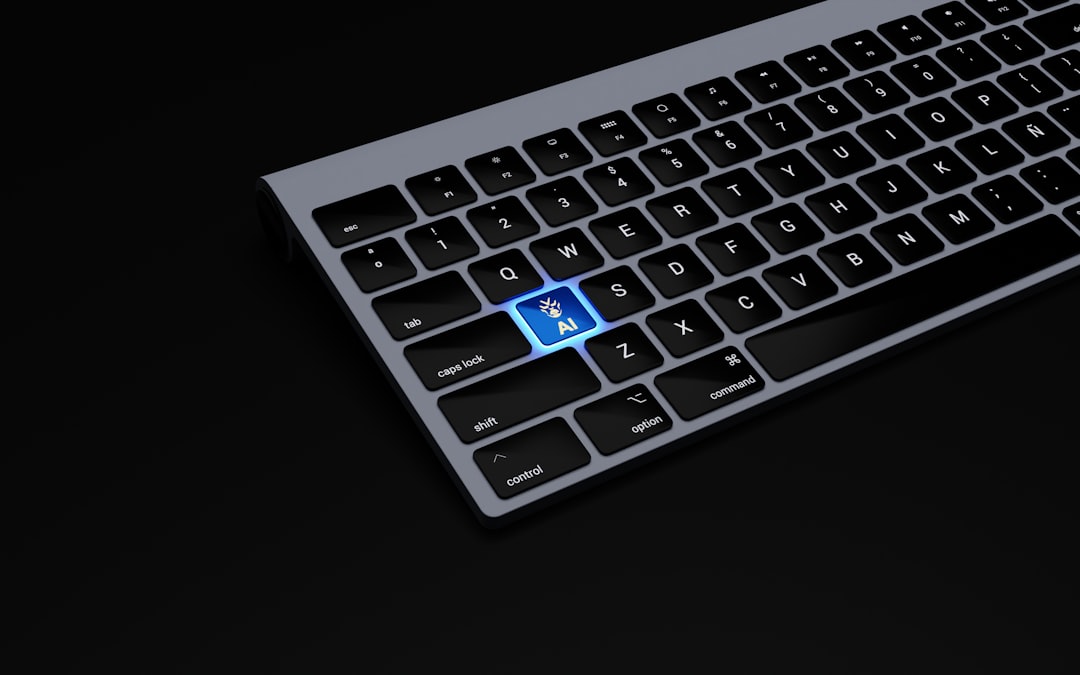
DALL-E 2 stands out as a pioneering text-to-image model, demonstrating impressive capabilities in manipulating images based on complex textual descriptions. It goes beyond simply generating images, offering control over object attributes and positions within the scene. This allows for a degree of fine-tuning and editing that wasn't readily available with previous AI image generation techniques. The addition of an inpainting feature allows users to make intricate modifications to existing images. The model has been adopted by a global community of artists, highlighting its role as a tool for both exploration and creative expression. While DALL-E 2's potential as a creative assistant is evident, its growing prominence also fuels conversations regarding the broader implications of AI for the arts. As AI image generation continues its rapid advancement, DALL-E 2 represents a pivotal point in this evolving field, prompting us to consider how these powerful tools might reshape the future of visual creativity.
DALL-E 2 stands out in the landscape of AI image generation by leveraging a novel training method called "CLIP-guided diffusion." This combines contrastive language-image training with diffusion models, allowing it to interpret complex text descriptions and generate images with remarkable detail. While traditional methods often struggle with high-resolution outputs, DALL-E 2 comfortably produces images up to 1024x1024 pixels, capturing nuanced details that are often crucial for practical applications.
Interestingly, DALL-E 2 uses a dual architecture with separate text encoder and image decoder components. This distinct approach lets it carefully dissect the context and semantics within prompts before generating the image, showcasing a significant advancement over earlier single-model systems. It's a testament to the ability of AI to process and understand language in a way that enables nuanced image creation.
Furthermore, DALL-E 2 has proven adept at interpreting artistic styles. It can generate images that replicate famous artists or specific movements simply from text descriptions, which is fascinating. This capability demonstrates not just a technical advancement, but also a capacity for deep learning to capture and reproduce human creative expressions.
The "inpainting" functionality in DALL-E 2 is particularly noteworthy. Users can feed the model partial images, and it intelligently fills in the missing portions while maintaining contextual integrity. This feature grants users a level of control and manipulation rarely seen in generated images.
DALL-E 2 also reveals an intriguing aptitude for abstraction. It can generate conceptual illustrations based solely on text, even when clear visual references are absent. This includes fantastical or imagined compositions that go beyond traditional image generation paradigms, and it's definitely something to consider for future directions.
Another interesting aspect is DALL-E 2's resistance to common biases often found in generative models. Through careful selection of training data and continual refinement, it aims to promote fairness in its outputs, which is a critical consideration for responsible AI development.
Its integration with an API is another notable feature. This allows developers and creators to integrate DALL-E 2's capabilities into their applications, potentially opening up exciting new possibilities across various creative fields and software development.
The iterative nature of its training is crucial for refining DALL-E 2's ability to understand user intent. Through continuous human feedback on generated images, it learns and adapts, improving its accuracy with each iteration. It shows a promising direction for feedback loops in AI training.
Ultimately, DALL-E 2 exemplifies a growing trend in AI image generation: a merging of artistic expression and machine learning. As these systems become increasingly sophisticated in recognizing and replicating human aesthetics, it leads to intriguing questions about authorship and AI's role in shaping creative practices. It's a fascinating area of research that promises to redefine the landscape of creativity in the coming years.
Advancements in AI Image Generation A Deep Dive into Text-to-Image Models in 2024 - Semantic Consistency Challenges in Text-to-Image Synthesis
The ability to generate images that accurately reflect the meaning of textual descriptions, a process known as semantic consistency, remains a primary hurdle in text-to-image synthesis. While advancements in areas like GANs and diffusion models have produced stunningly realistic images, the challenge of translating complex semantic information from text into visuals persists. Even though we have new ways to measure the degree to which generated images match the text—like Semantic Similarity Distance—the field still struggles with creating models that bridge the gap between what is written and what is shown. Researchers are exploring innovative strategies, including techniques like MSCAGAN and transformer-based models, to create images with both visual appeal and faithful adherence to the intended meaning of the text prompts. The ongoing pursuit of semantic coherence in AI-generated images underscores the dynamic nature of this field, driving researchers to refine methods that allow these models to more effectively grasp the subtleties of human language and translate them into visually compelling results.
1. **Grasping the Nuances of Language:** Text-to-image models, like Imagen, are getting better at understanding the subtle intricacies of language. They're picking up on things like idioms and how word meanings change based on context, which helps them generate pictures that really capture the different layers of meaning in the text.
2. **Measuring Semantic Fit:** We're seeing progress in developing ways to measure how well a generated image matches the meaning of the text prompt. These new metrics provide a more objective way to see how closely the AI-made image aligns with what the text describes, bridging the gap between subjective human judgements and a more scientific evaluation of the models.
3. **Zero-Shot Prowess:** Lots of the current text-to-image models are surprisingly good at generating relevant images even if they haven't been specifically trained on those types of prompts. This suggests a deeper understanding of language, but also that the way these models are built allows for a good degree of adaptability. It hints at possibilities for these models in fields like art and design.
4. **Maintaining Context:** Newer models are becoming better at keeping track of the overall context as they generate an image. They can process the relationships between different parts of the text prompt, and adjust the visuals to ensure everything in the picture fits together and tells a coherent story as defined by the prompt.
5. **Giving Users Control:** Features like DALL-E 2's inpainting capability let users make very precise changes to images, showcasing a significant shift in how these AI image generators work. Users can guide the creative process by modifying specific parts or attributes, leading to a more interactive experience and ensuring a better match with their artistic vision.
6. **Being Sensitive to Culture:** Current models are becoming more sensitive to cultural contexts, which is helpful for reducing the chances of generating images that might be offensive or culturally insensitive. They achieve this through more diverse training data and algorithms that help filter out biases, highlighting a growing sense of responsibility in how AI is designed.
7. **Learning Across Different Fields:** Models trained across multiple areas, like art and science writing, tend to have fewer problems with semantic consistency. They create more coherent and relevant images, demonstrating the value of learning from a wider range of data.
8. **Feedback Loops for Improvement:** Modern models often include iterative feedback mechanisms during training, allowing them to adapt based on actual user interaction. This continuous learning process means they can learn over time what makes a picture semantically consistent, and adapt to user expectations more effectively.
9. **Mimicking Artistic Styles:** The latest text-to-image models can now imitate various art styles and movements. They achieve this using advanced training techniques that capture the essence of different artistic styles, establishing a connection between technology and traditional art forms.
10. **Building Standardized Benchmarks:** Projects like DrawBench offer a way to compare the semantic consistency of various text-to-image models. These benchmarks give a clearer picture of a model's strengths and weaknesses, pushing the field forward in achieving stronger alignment between language and images in AI.
Advancements in AI Image Generation A Deep Dive into Text-to-Image Models in 2024 - Visual Text Generation Accuracy Remains a Key Hurdle
While AI image generation has seen remarkable progress, accurately rendering text within images remains a significant obstacle. Despite advancements in model architectures and the introduction of evaluation benchmarks like GenAIBench, the ability to consistently translate complex text prompts into visually faithful representations continues to be a hurdle, especially in real-world situations. Existing metrics, including CLIPScore, while helpful, fall short when capturing the intricate relationship between text meaning and image content. Efforts like the development of TextHarmony, aiming to resolve the decline in performance when generating both text and images simultaneously, demonstrate ongoing attempts to bridge the gap between text and visuals. However, the challenges associated with achieving high-fidelity text within complex visual scenarios are far from resolved, underscoring the need for more advanced evaluation methods and model designs to refine the accuracy and quality of visual text generation.
Visual text generation, while showing impressive strides, still faces key challenges when it comes to accurately translating text into images. Even though we've seen improvements in how models understand language and context, capturing the full nuances of human language in visual form remains difficult. Things like idioms and how words change meaning depending on the situation are still tricky for these models to translate into images.
Finding reliable ways to measure how well a generated image reflects the text it came from is also a hurdle. While new tools like Semantic Similarity Distance exist, there's no universally agreed-upon way to measure semantic consistency, and a lack of standardized benchmarks makes it hard to objectively compare the progress different teams are making.
We also see issues with models' ability to maintain context in longer pieces of text. The longer the text prompt, the more challenging it becomes for the model to generate images that align with all parts of the text, impacting the reliability of these systems for complex storytelling or narratives.
Another area of concern is dealing with ambiguity in the text. When prompts contain unclear or multi-layered meanings, models may misunderstand or miss vital parts, leading to inaccurate image outputs. This shows the ongoing challenge of ensuring the intended message is preserved during the image generation process.
Cultural sensitivity remains a concern as well. While efforts are being made to create more inclusive datasets and training approaches, the models still sometimes generate images that are insensitive to cultural contexts and social nuances.
Giving users more control over the image creation process is also an area that requires refinement. Features like inpainting can be helpful, but not everyone has the skills to effectively manipulate AI-generated outputs. This highlights the need for developing more accessible tools for non-expert users.
While zero-shot learning is impressive, there's often a trade-off between a model's ability to generalize and its effectiveness in niche areas. Models trained for general purposes may not have the specific fine-tuning required for tasks like medical imaging or scientific illustration.
Bias in training data remains a problem. Despite efforts to mitigate bias, the complexities of cultural and societal biases make it difficult to fully eliminate such issues during the development of AI models.
Feedback loops are helpful but their effectiveness relies heavily on the quality and quantity of the feedback. Limited user involvement can slow a model's iterative learning process, hindering its progress in achieving semantic accuracy.
As we create new benchmarks for evaluating semantic consistency, it's important to consider the growing diversity of both text and image outputs. Artistic styles and contexts vary widely, demanding adaptable standards. Establishing these flexible assessment criteria is a continuously evolving process.
Overall, the path toward achieving perfect alignment between text and image remains a complex one, with plenty of challenges ahead. Researchers are working to push the boundaries in areas such as context awareness, cultural understanding, and user control. But as this exciting field advances, it's important to keep a critical eye on the potential limitations and consequences associated with this technology.
More Posts from colorizethis.io: