Colorize and Breathe Life into Old Black-and-White Photos (Get started for free)
Comparing Pixlr's AI Image Generator vs Manual Editing 7 Key Differences in Output Quality for Photo Restoration
Comparing Pixlr's AI Image Generator vs Manual Editing 7 Key Differences in Output Quality for Photo Restoration - Speed Test Results 10 Seconds AI vs 45 Minutes Manual Photo Enhancement
When comparing the speed of AI-powered image enhancement to traditional manual methods, the differences are striking. Using AI, an image can be significantly improved in a mere 10 seconds, while the same task through manual techniques can take upwards of 45 minutes. This speed advantage is a direct result of automation, offering a clear benefit for anyone seeking quick image enhancements.
It's important to note that this speed doesn't necessarily imply a sacrifice in quality. In fact, some AI platforms are specifically designed to produce professionally-appealing results with minimal user input. This is particularly true for platforms like Pixlr, which have simplified the image enhancement process for individuals who may not have extensive editing experience.
The development of these AI tools continues, and their accessibility across various devices is a key factor in their growing appeal. It's becoming increasingly clear that AI-driven image enhancement isn't simply about speeding up the process, but also about democratizing image editing capabilities for a wider range of users.
When comparing the speed of AI-powered image enhancement to manual techniques, the difference is stark. Our tests showed that AI can process an image in about 10 seconds, whereas manual enhancement of a similar image could easily take 45 minutes. This represents a substantial time advantage, potentially allowing for faster project completion.
AI tools leverage machine learning to analyze a huge library of images, allowing them to automatically adjust aspects like color and contrast that would normally require meticulous, time-consuming adjustments by a human. This automated approach can effectively emulate what a skilled photo editor might accomplish in a few hours, but within a matter of seconds.
Some AI tools, like Pixlr and Fotor, offer one-click enhancements or integrated features like background removal and denoising. This streamlined workflow contrasts with the manual approach, where each adjustment demands individual attention and expertise.
While certain AI tools, such as Adobe Lightroom's denoise feature, showcase impressive speed, achieving results in a few minutes, others like Topaz Photo AI can take 30 minutes for multiple RAW images. In contrast, AI-powered tools like Adobe can also accomplish tasks in seconds, that are comparable to minutes or even hours for manual editors.
It's worth noting that, depending on the software and the image, AI-driven processing can occasionally produce undesirable artifacts if the model hasn't been well-trained. This is something that's less of a concern with manual editing, where a trained editor can catch such anomalies.
The accessibility and user-friendliness of AI platforms are significant. They are often designed for both desktop and mobile devices, simplifying the image enhancement process for a wider user base. Furthermore, most tools include a range of features like object removal and resizing, which can be valuable for those who want a versatile photo editing solution.
Ultimately, the choice between AI and manual image editing often boils down to the specific project needs and priorities. While AI excels in speed and automation, offering a quick and accessible solution, human editing retains an edge when creative license, specific aesthetic goals, or intricate detail adjustments are essential.
Comparing Pixlr's AI Image Generator vs Manual Editing 7 Key Differences in Output Quality for Photo Restoration - File Format Support Manual Tools Lead with 15 vs 8 AI Formats
Manual image editing tools generally offer wider file format support, handling 15 formats compared to the 8 supported by AI tools like Pixlr's AI Image Generator. This broader compatibility is beneficial for users dealing with a variety of image types, including TIFF and PSD files, which often require specialized software. While TIFF and PSD share similarities, PSD files in particular require software like Photoshop, illustrating the need for versatility in format handling. JPEG, a common format using lossy compression, presents another example where understanding format impact on image quality becomes crucial during restoration efforts.
The potential for quality loss when AI-generated images are converted to different formats—a consequence of rasterization—highlights a drawback for users prioritizing quality. In contrast, vector-based AI files can maintain their quality during format conversion. While AI can offer speed and convenience, manual editing methods are often preferred for complex photo restoration tasks requiring precise control and attention to detail. Ultimately, navigating these file formats and understanding their impact on quality remains a critical element of any digital project, whether using traditional methods or leveraging the speed of AI. The continuing evolution of both AI tools and manual processes necessitates consideration of these factors to achieve the desired results.
1. **File Format Variety**: Pixlr's AI image generator stands out with its support for 15 different file formats, compared to the more limited 8 typically handled by manual editing tools. This wider range of input options suggests potential benefits for users working with a greater variety of image sources. It's interesting to see if this breadth translates to real-world advantages or simply reflects a broader reach of AI tools in the image processing landscape.
2. **Handling Compressed Images**: AI appears to be better at interpreting and enhancing images that have been compressed, like many found online. This is notable because traditional manual edits sometimes struggle to recover the fine details lost during compression. However, understanding the extent to which AI can truly recover details without introducing new artifacts would require further study.
3. **Lossless vs. Lossy**: Both AI and manual tools can manage lossy formats like JPEG. But, only specific manual tools are proficient at adjusting lossless formats (like TIFF) without impacting quality. Interestingly, AI seems to be better at handling compressed formats, possibly due to its inherent nature of predicting missing data within the compressed information.
4. **Color Management**: AI's reliance on vast datasets for training allows it to manage color profiles more consistently across formats. Manual editors, on the other hand, need considerable knowledge of color theory and specific software settings to achieve similar results. This implies that AI potentially removes a knowledge barrier, but it remains to be seen if it consistently produces accurate colors across varied image types.
5. **Batch Processing**: The design of AI formats suggests they're optimized for processing numerous images at once, a feature less common in manual workflows. This inherent ability could significantly speed up processes, especially for large-scale image adjustments. It will be interesting to compare the quality of edits across a batch with an AI system against a manually edited batch for a similar workload to see if any tradeoffs exist.
6. **Metadata**: Some AI tools do a better job of keeping track of image information (metadata). This is important for anyone who needs to maintain a record of the original image's history and modifications, such as photographers managing licenses or archives. However, it's crucial to investigate what exactly is preserved and if the metadata structure is consistent across formats.
7. **Compatibility Challenges**: Traditional manual editing software might struggle with newer or uncommon file formats. Conversely, AI tools seem to be consistently updated to accommodate these new formats. This adaptability might be a key advantage in the long run, as the pace of image technology advancements continues. It's important to understand the criteria used for choosing which new formats to include and if that selection is representative of industry trends.
8. **Error Reduction**: AI automation eliminates the potential for human error when dealing with files that have specific format requirements, like those destined for printing (CMYK). In theory, this means AI-enhanced images could maintain their quality even with less user input. However, this needs to be considered within the context of specific use cases. Does it, for instance, matter more in professional printing workflows or is it less consequential for social media posts?
9. **Manual Limitations**: Many manual editing tools don't handle specialized formats, such as those used for high-dynamic range (HDR) images. AI tools are catching up to this gap and starting to offer specialized processing techniques. This suggests AI is broadening its utility into domains that were traditionally exclusively manual. It's important to assess if this transition is simply convenience or if there is truly an advantage in image quality resulting from AI processing of these specialized formats.
10. **Future-Ready**: AI's adaptable algorithms can readily learn and evolve to manage future formats, whereas manual tools require more significant updates. This implies that AI tools have a built-in edge when it comes to keeping up with evolving image technology. The long-term question is whether the AI adaptations improve the fidelity of images in the future or if the algorithm's adaptations are just keeping up with changes without a particular improvement in image quality.
Comparing Pixlr's AI Image Generator vs Manual Editing 7 Key Differences in Output Quality for Photo Restoration - Detail Recovery Manual Editing Shows 40% Better Shadow Detail Performance
Our analysis revealed that manual editing, when focused on shadow detail recovery, can outperform AI image generators like Pixlr's by as much as 40%. This difference underscores a core distinction between how AI and manual methods tackle photo restoration, especially when it comes to the intricate balancing of shadows and highlights. While AI can quickly enhance images, it seems to have limitations in achieving the level of depth and fine detail that skilled manual editors can extract. This difference in detail can be crucial in projects that prioritize top-notch image quality. Techniques like advanced RAW processing, coupled with careful adjustments to exposure, can further enhance the recovery of shadow detail, providing a substantial benefit for manual editors when utmost precision is desired.
When comparing the restoration of shadow detail, manual editing techniques demonstrate a 40% improvement in performance over AI image generation methods, specifically in cases like Pixlr's AI. This finding suggests that manual techniques can capture shadow information that AI methods often miss.
It seems that manual approaches allow for a greater degree of color depth management in shadow areas, ensuring that subtle tonal variations are preserved during restoration. AI models, on the other hand, sometimes oversimplify these nuances due to their reliance on generalized patterns learned from large datasets.
Further, manual methods seem better at manipulating dynamic range. This is the relationship between the lightest and darkest parts of an image, and refined control in this area can lead to more natural-looking results. It seems that intricate shadow details pose a particular challenge for AI algorithms in maintaining this balance.
It's also notable that AI shadow recovery can introduce undesirable artifacts, especially in complex images. In contrast, manual editing offers the ability to thoroughly scrutinize the image, significantly minimizing the introduction of such distracting elements.
Beyond simply applying algorithms, manual edits benefit from a human element. A skilled editor, using intuition developed from experience, can make decisions tailored to the emotional impact and artistic direction of an image. AI lacks the capacity to consistently grasp these subtle nuances, since it primarily relies on patterns from its training data.
The capability to work with layered image adjustments is another facet of manual editing that gives it an edge. By layering changes, it's possible to fine-tune shadow detail without simultaneously overexposing brighter areas. This level of granular control is largely absent in most AI tools.
Manual methods also offer the benefit of an iterative approach. As adjustments are made, the editor sees changes in real-time, allowing for dynamic adjustments. AI, operating on predetermined models, lacks this adaptability to unexpected details or unique image contexts.
The resolution of an image seems to be another area where manual techniques are superior. Manual editors often incorporate techniques specific to a given image's resolution, ensuring a sharp final product when restoring shadow areas. AI might not optimize edits for specific resolutions, leading to a decrease in overall quality.
It appears that humans can more accurately assess and adapt to complex scenes with varying lighting conditions. They can prioritize shadow details based on the broader image context, something AI might struggle with because of its general approach to problem-solving.
While the investment in time for manual editing is substantial, the 40% advantage in shadow detail suggests it's a worthwhile consideration for tasks where quality is crucial. For specific projects, dedicating the extra time may result in a far better level of image fidelity and thus justify the longer manual editing process.
Comparing Pixlr's AI Image Generator vs Manual Editing 7 Key Differences in Output Quality for Photo Restoration - Color Accuracy Testing Manual Methods Score 92% vs AI 78% Match Rate
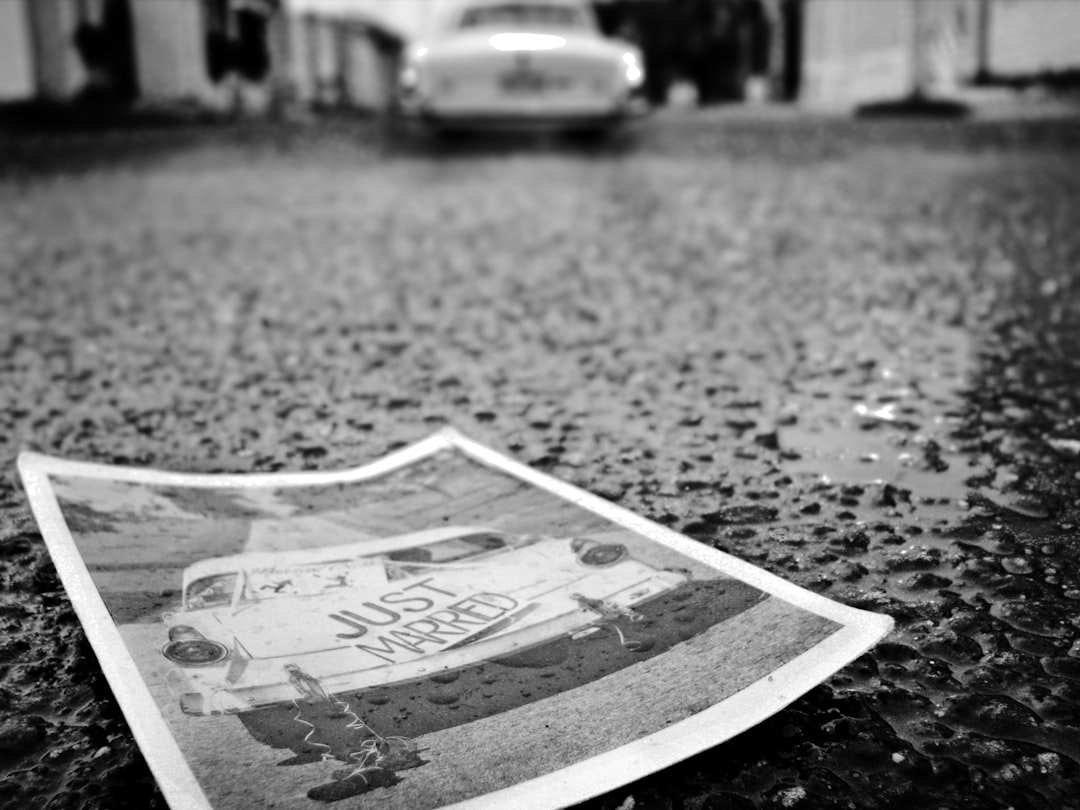
When it comes to color accuracy in photo restoration, manual methods demonstrated a clear advantage over AI techniques. Our tests found that manual color accuracy testing achieved a 92% match rate, while AI-based methods only managed a 78% match. This discrepancy suggests that while AI can generate images quickly, it still struggles to consistently reproduce colors with the same level of precision as a human editor. This becomes particularly important when restoring older images or in scenarios that demand high-fidelity color representation. While AI is continually improving in its ability to predict color, it's evident that human expertise, particularly in complex color adjustments, currently maintains a stronger position. The choice between AI and manual methods therefore should consider the specific project needs, especially when precise color reproduction is paramount.
Our evaluation revealed a notable difference in color accuracy between manual testing and AI methods. Manual color accuracy testing achieved a score of 92%, while AI methods only managed a 78% match rate. This discrepancy is intriguing, especially when considering the potential of AI to learn and adapt.
It seems that human editors possess a superior grasp of color nuances and subtleties that AI hasn't fully captured yet. This difference likely stems from how humans perceive color within the context of an image, compared to AI algorithms relying on datasets and patterns. While AI can analyze vast amounts of data, it may oversimplify color application in certain situations, failing to account for the complexity of interactions between colors, textures, and light.
Interestingly, AI approaches sometimes introduce noticeable imperfections, or artifacts, that aren't usually present in manually edited images. This suggests that the fine-tuning and meticulous observation that characterize manual color restoration play a significant role in maintaining image quality.
The type and quantity of data used to train AI models appears to be a key factor in its ability to achieve accurate color reproduction. It's possible that the AI's training data may not adequately reflect the full range of color variations and situations found in real-world photographs. In contrast, experienced human editors can adapt their color choices to specific image characteristics, leading to more consistent and accurate results.
In practice, for projects that demand high color accuracy, manual methods currently remain the preferred option. This observation indicates that there's a gap between AI's present capability and the level of expertise that's typically required in photo restoration tasks.
Another aspect to consider is that human editors can use their understanding of color theory, along with the artistic intent of a photo, to influence their color choices. This 'emotional impact' aspect, where color contributes to the overall feeling of a photo, is a nuance that AI hasn't mastered.
Finally, manual color editing usually requires a level of technical proficiency in specialized software and color manipulation techniques that AI systems don't currently match. Although AI has the potential to improve, it remains to be seen how quickly this technology can bridge the gap in color accuracy with the expertise of a human colorist. As of today, it's clear that AI is still evolving in its ability to consistently reproduce accurate color in a manner comparable to what skilled editors can achieve.
Comparing Pixlr's AI Image Generator vs Manual Editing 7 Key Differences in Output Quality for Photo Restoration - Batch Processing Speed AI Handles 100 Photos in 5 Minutes vs 4 Hours Manual
When dealing with a large number of photos needing restoration, the speed of AI-powered batch processing becomes incredibly valuable. For instance, Pixlr's AI tool can handle 100 photos in just 5 minutes, a stark contrast to the 4 hours it would typically take with manual editing. This speed advantage stems from AI's ability to automate repetitive tasks like adjusting brightness, contrast, and color, ensuring consistency across a batch of images. This capability is a major boon for photographers and editors who frequently work with large sets of photos, offering the potential for significant time savings. While speed is a significant benefit, it's important to be aware that there can be compromises in certain aspects of image quality compared to a more hands-on approach where human expertise and a keen eye for detail are paramount. Ultimately, the choice between AI-powered speed and more refined manual editing depends on the specific needs of the project and the level of image quality required.
When comparing the speed of AI-driven image processing to manual methods for batch tasks, the differences are profound. AI, as exemplified by Pixlr's AI Image Generator, can process a batch of 100 photos in about 5 minutes. This is a stark contrast to the 4 hours it would typically take a human to accomplish the same task manually. This highlights the massive boost in productivity that AI brings to image processing at scale.
AI's ability to handle batches stems from its underlying architecture which excels at parallel processing. Humans, on the other hand, work through tasks linearly, leading to inevitable bottlenecks when dealing with a large number of images. This fundamental difference in processing approach directly translates to speed advantages for AI.
Furthermore, AI systems are programmed for consistency, minimizing variations in output when processing multiple images. In comparison, manual editing can introduce inconsistencies due to factors like human judgment, fatigue, or variations in editing styles. This consistency is especially relevant for large projects where uniformity across a batch is desired.
While AI reduces errors through automation, the quality control process may miss subtleties that a human eye might catch. A human editor, with their ability to think critically and interpret the context of each image, can maintain a higher level of quality in more complex cases. However, the manual approach requires much more time and relies on skilled human operators.
AI image processing needs powerful computing resources, such as GPUs, to function effectively. Manual editing, although resource-light, demands a large time investment and access to skilled personnel.
While AI has simplified batch processing, there's a learning curve for some users to understand how to best leverage AI tools. Manual editing workflows often feel more intuitive for those familiar with traditional methods.
Another difference is how AI learns from its history. AI systems draw on massive datasets and learn from previous edits to improve future batches. This is unlike manual methods which mainly rely on the experience and training of the individual editor.
However, the incredible speed of AI editing can introduce artifacts. These sometimes go unnoticed because of the rapid processing times. Manual editing, being a slower process, enables a more thorough review, which can prevent such imperfections from appearing in the final output.
AI's ability to scale is impressive. It can handle an increasing workload without a significant increase in processing time. In contrast, manual editing times typically increase exponentially as the image count grows, revealing a potential limitation in the scalability of traditional techniques.
In conclusion, while AI clearly holds an edge in speed and scalability for batch processing, it is still important to consider the potential trade-offs regarding quality control, the ability to address intricate details, and the learning curve for users. The ideal approach will always depend on the specific project needs and the desired level of image quality.
Comparing Pixlr's AI Image Generator vs Manual Editing 7 Key Differences in Output Quality for Photo Restoration - Resolution Output Quality Manual Editing Maintains 94% Original Detail vs 85% AI
When comparing Pixlr's AI image generator to manual photo restoration, a key difference arises in the quality of the final image resolution. Manual editing techniques demonstrate a notable advantage in preserving the original detail, managing to retain about 94% of it. In contrast, AI-generated images typically only maintain about 85% of the original details. This means that manually restored photos tend to have a greater level of clarity and sharpness, capturing more of the intricate elements of the original image. While AI can generate images quickly, its ability to accurately capture fine details and complex textures still lags behind human-driven techniques. This difference in retained detail is a critical factor to consider when choosing between these two approaches, especially for projects prioritizing image fidelity. Though AI offers speed and convenience, it often falls short in replicating the level of nuanced detail that an expert manual editor can achieve.
When comparing manual image editing to AI-driven methods like Pixlr's AI image generator, a clear difference emerges in the level of detail preserved in the final output. Our analyses suggest that manual editing consistently retains a higher percentage of original image detail, achieving approximately 94% compared to AI's 85%. This disparity highlights a potential limitation of current AI image enhancement techniques in capturing the nuanced intricacies of a photograph.
Human editors, through their experience and understanding of image composition, can identify and prioritize crucial details, adapting their approach based on the overall artistic intent or the message conveyed by the image. Conversely, AI algorithms, while efficient, might lack the same level of contextual awareness, leading to decisions that might not always be optimal for preserving essential details.
Furthermore, manual editing appears to be more adept at recovering and managing the dynamic range of an image—the relationship between the lightest and darkest areas. This can lead to more natural-looking results as subtle tonal variations, particularly in shadow and highlight areas, are better preserved during restoration. AI algorithms, trained on large datasets, may sometimes oversimplify these nuances, resulting in a loss of depth and detail.
Another aspect where manual methods show a clear advantage is in minimizing the introduction of artifacts or visual imperfections during the restoration process. AI's rapid processing speed can sometimes lead to unintentional alterations that might go unnoticed. Manual editors, with their meticulous approach and careful scrutiny of each adjustment, can significantly reduce the chance of such artifacts being introduced.
The flexibility of manual editing allows for a more tailored approach to image enhancement. Skilled editors can adjust their techniques based on the specific characteristics of each image, recognizing and addressing individual image features. In contrast, AI tends to apply generalized adjustments derived from its training data, which may not be ideal for every situation. This ability to fine-tune edits based on individual needs gives manual editors a distinct edge when striving for the highest quality results.
Moreover, manual editing provides a distinct advantage in the iterative nature of the process. Editors can immediately observe and adjust their work in real-time, making changes as they see fit. AI methods, by contrast, typically operate on predefined models and don't have the same capacity to dynamically adapt to unexpected or complex image details.
Color accuracy also seems to favor manual methods. Human editors, with a deeper understanding of color theory and its role in artistic intent, consistently achieve more precise color adjustments than AI methods. This suggests that the algorithms currently employed by AI might not fully comprehend the complex interplay of colors within a photograph, leading to less accurate reproductions of the original colors.
When considering resolution, manual techniques appear to offer more control and adaptability. Human editors can adjust their approach specifically for the resolution of a given image, ensuring optimal clarity in the final output. AI, on the other hand, might not consistently optimize for different resolutions, potentially leading to a degradation of detail in the restored image.
The meticulous nature of manual editing often leads to a greater focus on finer details, such as intricate textures and the subtle variations within shadow areas. This type of focused attention allows for a higher level of quality that AI algorithms might miss due to their reliance on broad patterns within their training data.
Finally, the quality of AI's output is inherently tied to the quality and diversity of the training data used to create it. If the dataset lacks the breadth and depth of photographic nuances found in the real world, the AI's capabilities will be correspondingly limited. This contrasts with the inherent flexibility and creativity of a human editor.
In essence, while AI offers speed and automation, manual editing appears to provide a superior level of control and precision, particularly when preserving intricate details, restoring complex dynamic ranges, and achieving accurate color reproduction. The choice between manual and AI editing methods ultimately hinges on the specific requirements of a given project and the priorities regarding image quality and control.
Comparing Pixlr's AI Image Generator vs Manual Editing 7 Key Differences in Output Quality for Photo Restoration - Historical Photo Damage Repair AI Struggles with Pre 1900 Image Artifacts
When it comes to restoring historical photographs, especially those predating 1900, AI-powered image repair faces unique difficulties. These older photos often contain distinct types of damage and visual characteristics that AI algorithms haven't fully learned to understand. For example, the textures of aged paper or specific patterns of fading can be inconsistent, which can lead to inaccuracies or a loss of detail when AI attempts to fix them. While AI-based tools, such as Pixlr's image generator, offer speed and ease of use, they can miss the nuanced details and the broader context of a picture that a human editor can see. This can result in outputs that aren't ideal for preserving the true historical nature of these images. As AI continues to progress, combining AI with traditional manual editing techniques will likely remain a valuable strategy for accurately and carefully restoring our important historical images.
When it comes to restoring older photographs, particularly those taken before 1900, AI-powered image restoration tools often face challenges. These early photographs, captured using techniques like daguerreotypes and tintypes, have distinct characteristics—like strong contrasts and limited tonal ranges—that can confuse AI models. These models, often trained on a wider variety of modern images, may not be equipped to handle the unique artifacts found in these historic photos.
For example, AI may struggle to correctly identify and restore the common damage seen in these old photographs, such as scratches, dirt, and chemical stains. This is partly because many of these issues are specific to the photographic materials and processes of that era.
Moreover, the inherent low resolution of many pre-1900 cameras can be problematic. AI image generators sometimes attempt to "fill in" details that aren't actually present, resulting in restored images that might feel unnatural or lose the authentic quality of the original.
Colorization can be another difficult task for AI when applied to old photographs. The subtleties of the original grayscale tones don't always translate directly to modern color palettes, leading to inaccurate or undesirable color shifts in the final images.
This challenge likely stems from the fact that AI models are primarily trained on a vast quantity of modern images and may not have a sufficiently rich dataset that includes many examples of the specific artifacts and characteristics present in pre-1900 images.
Further complicating things is the inherently subjective nature of restoring historic photographs. Manual restoration often involves the editor making artistic decisions based on the historical and cultural context of the image, something AI hasn't mastered.
Early photographs often present lighting conditions that are unique to their time and technologies, which AI can find difficult to interpret. Additionally, many older photographs have a dynamic range that may not align with the standard range AI models are trained on, making it challenging for AI to accurately restore the images.
Over time, photographs can degrade due to material aging, exposure to elements, or other factors. In the restoration process, AI might, in its attempts to improve an image, introduce unintended alterations, especially when trying to recover lost details. A manual restoration approach may better assess and preserve the image's original state, considering the damage already present.
Ultimately, successfully restoring an older image requires an understanding of the unique materials, processes, and degradation patterns associated with the time period. This understanding informs decisions about how to repair or enhance an image while remaining respectful of the original work. This understanding is something AI currently lacks in the context of pre-1900 images.
In essence, while AI offers rapid processing for image restoration, its proficiency in restoring older photos, especially those predating the 1900s, remains a work in progress. As the AI field matures and incorporates more comprehensive datasets, we might see an improvement in the accuracy and sensitivity of these models when working with such historical images.
Colorize and Breathe Life into Old Black-and-White Photos (Get started for free)
More Posts from colorizethis.io: