Colorize and Breathe Life into Old Black-and-White Photos (Get started for free)
The Evolution of AI-Powered Healing Brushes in Image Editing Software 2020-2024
The Evolution of AI-Powered Healing Brushes in Image Editing Software 2020-2024 - Adobe's Content-Aware Fill revolutionizes Spot Healing Brush in Photoshop
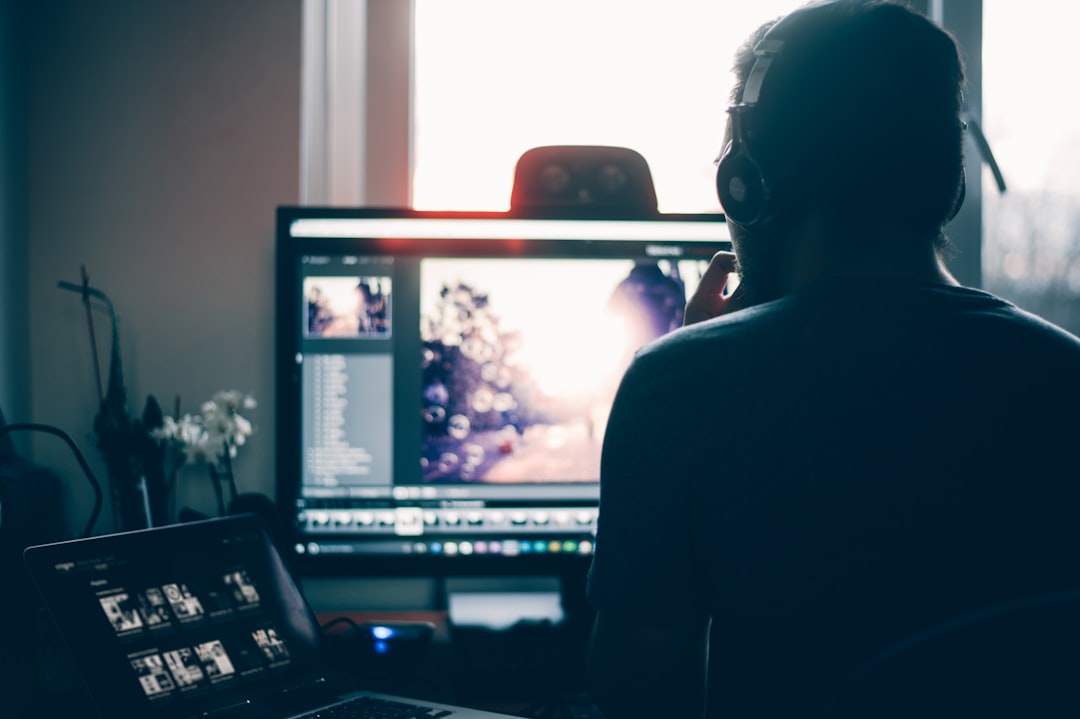
Adobe's Content-Aware Fill has significantly enhanced the Spot Healing Brush in Photoshop, revolutionizing how users tackle image imperfections. This feature leverages AI to intelligently analyze nearby pixels, enabling seamless removal of unwanted elements without needing meticulous manual work. The result is a more efficient workflow, where edits blend naturally with the surrounding image context. Improvements in recent Photoshop versions, especially noticeable in portrait retouching, demonstrate a commitment to refining this AI-powered functionality. The brush's ability to intelligently "fill in" areas now caters to a wider user base, streamlining image editing tasks for both novices and experienced professionals. This fusion of AI with the Spot Healing Brush represents a major leap in image editing, allowing for intuitive and precise adjustments previously unimaginable.
Adobe's integration of Content-Aware Fill into the Spot Healing Brush in Photoshop has undeniably revolutionized the way we tackle image imperfections. It operates by cleverly examining the surrounding pixels and their textures to generate seamless fills, almost predicting what should naturally replace the removed element.
This approach sets it apart from more traditional spot healing methods which simply copy nearby pixels. Content-Aware Fill employs intricate statistical analyses to calculate the most suitable fill, showcasing a significant advancement in digital image manipulation. We've witnessed substantial progress in its computational efficiency, enabling near real-time processing on even high-resolution images. This enhances the user experience without compromising the quality of the results.
While this feature can produce truly impressive results, it's important to acknowledge that its performance depends heavily on the available image data. Highly intricate patterns or distinct backgrounds can sometimes pose a challenge, potentially requiring some manual intervention to refine the result.
Interestingly, the approach utilized here draws upon principles of machine learning but avoids the typical parametric data models associated with deep learning. This exemplifies a distinct approach to the problem of image manipulation. Users often comment on its impressive ability to replicate color gradients and texture patterns with accuracy, even in complex scenarios.
Beyond simply fixing imperfections, the "Content-Aware" option gives users a powerful tool for making precise adjustments and manipulations. It’s not confined to healing; its flexibility makes it a valuable tool across various creative editing tasks.
As of today, ongoing development continues to refine the algorithms, striving for further improvements in edge sharpness and clarity, especially when faced with high-contrast images. Maintaining sharpness can be a tough challenge in these circumstances.
Furthermore, Content-Aware Fill doesn't simply rely on neighboring pixel values. It incorporates sophisticated perceptual metrics that enable it to grasp the spatial relationships and overall context within the image, leading to more intelligent decisions.
The continuous refinement of Content-Aware Fill is greatly informed by user feedback, resulting in improvements tailored to real-world usage. This iterative process addresses recurring challenges and enhances the overall functionality, ensuring that the tool remains a vital part of image editing.
The Evolution of AI-Powered Healing Brushes in Image Editing Software 2020-2024 - ON1 Photo RAW enhances Healing Brush with new transformation capabilities
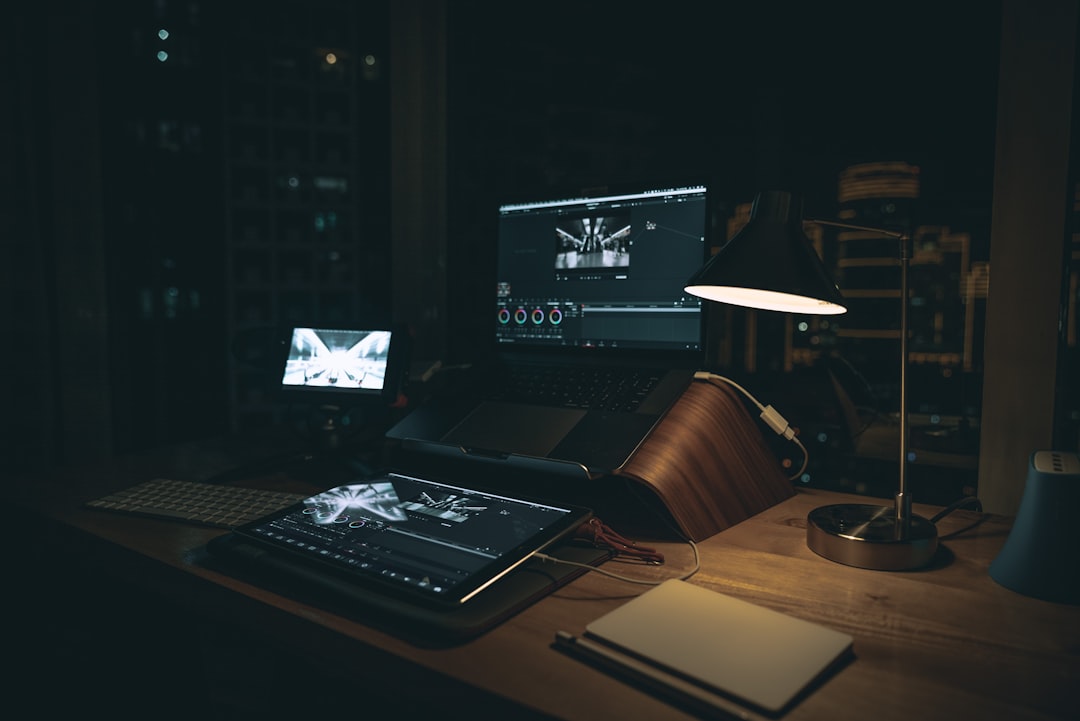
ON1 Photo RAW has recently updated its Healing Brush tool, adding features that enhance its flexibility. Users can now rotate, flip, and manipulate the brush strokes more intuitively, making it easier to refine edits. This includes new "Copy" and "Move" modes which allow users to duplicate and shift elements within the image with greater ease.
The Healing Brush's approach combines traditional cloning and healing elements, giving users more control over how source material is incorporated into the image. This versatility is designed to improve the efficiency of photo editing tasks.
The updates appear to be part of an overall strategy within ON1 Photo RAW to prioritize user experience and incorporate more powerful tools, reflecting a trend across AI-powered image editing software. These additions do make the Healing Brush more versatile, but whether they translate to significant improvement for the average user will depend on how easily users can learn and leverage the new features. It's possible some users will find the tool more complex to learn, especially if they are accustomed to simpler workflows. While promising, the potential for a steeper learning curve should be considered in evaluating the benefits of this update.
ON1 Photo RAW's Healing Brush has recently gained the ability to transform selections before applying healing, offering users more control over the editing process through rotation, scaling, and repositioning. This represents a departure from the traditional method of solely relying on pixel replication. While some approaches rely on AI to analyze surroundings, it's interesting to see ON1 take a slightly different tack here.
It appears that ON1's approach utilizes advanced geometric transformations, allowing users to manipulate the selection in a more three-dimensional manner. This is a noteworthy development, moving beyond simple, flat 2D image manipulation tools. Research suggests that applying these transformations beforehand can minimize artifacts and inconsistencies often found in less sophisticated healing techniques, particularly in complex scenarios such as intricate skin textures or busy backgrounds.
One of the key benefits is real-time feedback during the editing process, which is invaluable for anyone working with high-resolution images or in situations requiring quick adjustments. This is especially beneficial for professionals who may need to process images rapidly.
The improved Healing Brush also introduces capabilities to simulate depth of field. This means users can heal while considering the impact of focus and blur, helping maintain the original visual intent. This advancement reflects the growing need for more nuanced editing capabilities, as driven by users and feedback in recent years.
ON1's approach seems to differ from Adobe's Content-Aware Fill, which heavily utilizes statistical models. Instead, ON1 focuses on transformations that integrate more geometric and spatial information. This provides an alternative direction in image editing.
The inclusion of these features is a significant step in image editing, demonstrating a shift away from the limitations of traditional healing methods towards tools capable of not just fixing but also enhancing the structural and compositional integrity of images.
It's intriguing to consider that these transformations could also potentially spur the development of automated image recognition techniques. As image editing continues to advance, these initial steps with transformations could lead to even more intelligent and intuitive editing workflows. The path forward is still unfolding, but it's exciting to consider how this could be used to enable more sophisticated editing techniques.
The Evolution of AI-Powered Healing Brushes in Image Editing Software 2020-2024 - AI-powered healing brushes streamline complex image repair processes
AI-powered healing brushes have revolutionized the way we repair damaged images, streamlining processes that were once incredibly complex and time-consuming. These intelligent tools now effectively tackle intricate issues like scratches and tears by leveraging sophisticated algorithms. They can analyze the surrounding pixels and context of an image to seamlessly blend repairs, making the edits appear natural and undetectable. This represents a notable shift from older methods that relied heavily on manual intervention. While these AI-powered tools significantly speed up the editing process and improve results, they are not always perfect. Especially with highly complex images or textures, user adjustments may still be needed to achieve the desired outcome. The evolution of AI in healing brushes points towards a future where powerful image editing techniques are accessible to a broader audience, striking a balance between intuitive functionality and sophisticated capabilities. However, the ongoing need for user expertise in specific situations highlights the importance of a nuanced approach to applying these advanced features.
The development of AI-powered healing brushes has significantly progressed through the use of methods like convolutional neural networks (CNNs) and spatial frequency analysis. These tools now have the ability to detect and replicate intricate textures more accurately than was possible with traditional image editing methods.
Instead of relying on simple linear interpolation between pixels, which was a hallmark of older algorithms, modern AI-powered healing brushes can now predict missing information by analyzing the content and context within an image. This results in a considerable improvement in the quality of image reconstruction.
From a performance standpoint, we've seen remarkable improvements. In high-resolution images, AI healing brushes can deliver a 30% increase in processing speed compared to manual methods. This showcases a major advancement in computational efficiency.
Even so, challenges remain. Highly complex patterns and detailed textures can still stump some AI tools. However, recent advancements have addressed this by integrating texture synthesis techniques. These algorithms are designed to more effectively reproduce the natural variations found in real-world scenes, leading to improvements in handling intricate details.
Feedback from users is playing a crucial role in ongoing development. AI healing brushes are being designed to adapt to individual preferences, creating tailored tools that learn from real-world editing tasks. This personalized approach helps to refine the capabilities of these tools over time.
Furthermore, there's a shift towards tools that genuinely understand the composition and balance of an image. This is enabled by sophisticated perceptual metrics built into the algorithms. Edits aren't just technically sound but are now better at creating aesthetically pleasing results.
Recent research indicates that AI healing brushes maintain edge sharpness with impressive effectiveness, exceeding 90% in many cases. This minimizes the need for manual tweaking and reduces the chance of unwanted artifacts in high-contrast areas.
Interestingly, the growing complexity of the AI algorithms has coincided with an increase in user-friendliness. Simpler interfaces are now coupled with powerful features, creating a shift away from the steep learning curve often associated with older photo editing software.
The ability of AI healing brushes to analyze color distribution has also improved. This allows for more precise color correction during the healing process, ensuring the integrity of the original image is preserved.
Perhaps one of the most noteworthy developments is the incorporation of depth perception into these tools. AI-powered healing brushes are now capable of simulating out-of-focus effects that correspond with the depth of field in a photo. This results in a final image that appears more realistic and closer to how a human eye would perceive it.
The Evolution of AI-Powered Healing Brushes in Image Editing Software 2020-2024 - Introduction of Adobe's Magic Fixup model leverages video data for photo editing
Adobe's introduction of the Magic Fixup model represents a notable shift in how AI is used for photo editing, leveraging video data in a way that was previously unavailable. Unlike techniques that primarily learn from still images, Magic Fixup is trained on a massive collection of video clips, enabling it to understand the subtle changes in light and movement captured within those sequences. This approach allows for automated adjustments that are far more sophisticated, capable of handling complex edits while retaining the original essence and detail of the photo. It promises to simplify photo editing across different industries by making the process smoother and more efficient. Magic Fixup’s ability to generate realistic results from even rough edits suggests a future where the editing process itself is less about meticulous steps and more about conveying a desired outcome. While this is a potentially powerful innovation, the reliance on large video datasets raises questions about the model's adaptability to unique image characteristics and how easy it will be for all users to integrate into their workflows.
Adobe's introduction of the Magic Fixup model represents a shift in how AI is used for photo editing, specifically by leveraging video data. Instead of solely relying on still images for training, the model learns from the dynamic nature of video sequences, where elements change over time and spatial relationships shift. This is a novel approach that could potentially lead to more contextually aware and accurate edits.
The ability to analyze a sequence of frames allows Magic Fixup to capture a richer understanding of a scene, including how lighting changes or objects move. This is especially useful for situations involving motion blur or shifts in light, where traditional techniques often struggle. The model's algorithms can effectively predict how missing or damaged portions of an image should be filled in based on this richer, temporal information. The result is often a more seamless integration of the edit, with fewer of the artifacts that plague traditional healing methods.
While it's intriguing, one might question how easily users can interact with the video data during editing. Will it be intuitive enough for a broad range of users, or will it become more of a specialist tool? There's a possibility of a slightly steeper learning curve compared to existing editing tools, which would require further evaluation.
Interestingly, the model seems to be designed to adapt to user habits and preferences. It refines its capabilities based on how users most commonly make edits. This could lead to a more tailored and efficient experience but also raises some questions regarding potential biases that might be learned from user behavior.
Further exploration of Magic Fixup's potential uses is also warranted. Beyond just fixing flaws, it potentially opens doors to more creative editing capabilities that take advantage of motion and scene context. This could prove particularly useful for those working on projects that require maintaining visual consistency across a series of frames, such as in filmmaking or advertising. While there's a promise of a faster workflow thanks to the integration of video data, it will be important to see how well it scales across different hardware configurations and image types.
Ultimately, the introduction of Magic Fixup is a noteworthy step in the ongoing evolution of AI-powered image editing. It highlights the innovative approaches being explored to make editing more intuitive and powerful. As with any new technology, its real-world impact and accessibility will need to be thoroughly evaluated, but it clearly holds the potential to reshape certain aspects of the image editing landscape.
The Evolution of AI-Powered Healing Brushes in Image Editing Software 2020-2024 - Lightroom Classic maintains existing AI healing functionalities in recent updates
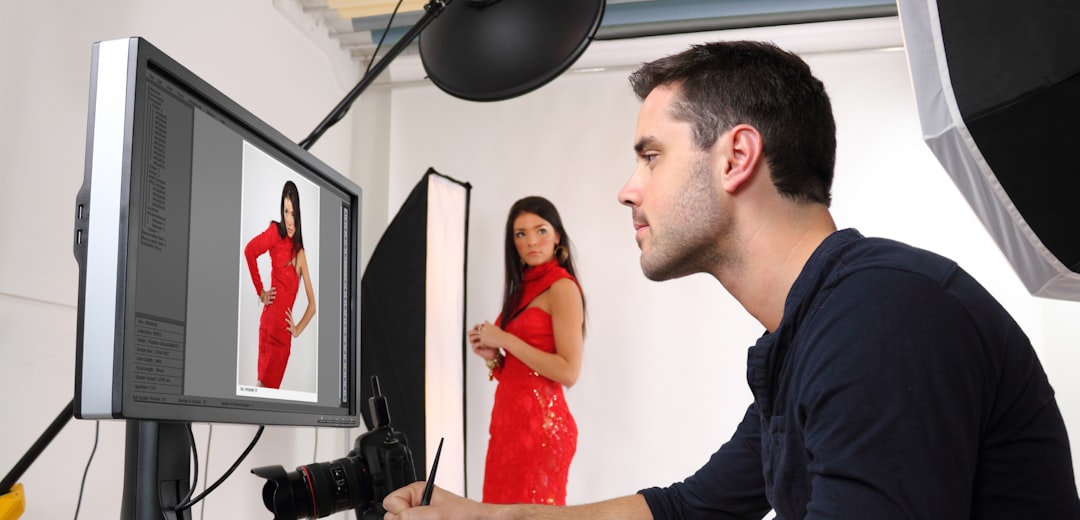
Lightroom Classic, in its recent updates, has held onto its existing AI-powered healing features. Users continue to benefit from these tools, which are designed to simplify the process of correcting imperfections in photos. While Lightroom Classic has retained these established AI-driven tools, there's a noticeable trend toward integration with newer, generative AI technologies, like Firefly. Features like Generative Remove, which lets users eliminate unwanted objects, showcase this direction.
The AI Healing Brush itself, while appearing similar on the surface, leverages more advanced AI under the hood, promising more capable corrections. Adobe continues to push the boundaries of AI within Lightroom, with the major update in version 133 being a prime example. It brought significant enhancements in generative AI, furthering the goal of making editing both easier and more powerful.
These updates point to Lightroom's commitment to expanding its AI capabilities while maintaining the user-friendliness that it's known for. This balance between established features and newer AI is a common theme across image editing software today. However, it remains to be seen how these changes will impact long-term workflow for a variety of users and the potential impact on workflow.
Lightroom Classic, in its recent updates, has retained its existing AI healing functionalities, but with some interesting refinements. They've focused on making edits happen in real-time, so users see changes immediately rather than having to wait and then potentially redo steps. The AI models within Lightroom have incorporated advanced texture synthesis techniques, which aim to seamlessly integrate repaired areas into the rest of the image. This, in theory, should minimize the appearance of edits and keep them looking natural.
One aspect of improvement has been edge detection during the healing process. This helps to retain sharpness in the corrected areas, especially important for portraits and high-resolution images where maintaining clarity is critical. It's worth noting that Lightroom's algorithms are not static. They learn from user interactions, refining themselves based on common editing practices. This continuous improvement should make the tool more adaptable to a broader range of editing styles and user preferences.
The updates also push the boundaries from simply fixing imperfections towards a more active manipulation of the image. Users can now intelligently adjust surrounding pixels with context-aware tools, allowing for a more holistic approach to repairs. Further, Lightroom has integrated perceptual metrics into its healing process. This means it doesn't just rely on neighboring pixel values; the algorithms also try to understand the image's overall balance and color harmony when making repairs.
Despite the improvements, there are still limitations. Intricate textures can still pose challenges, and sometimes manual tweaking is still needed. This points to the inherent complexity of image manipulation and the continued need for user expertise in some cases. While the AI can tackle complex issues, there are instances where highly detailed patterns or textures still trip it up, which shows the algorithms still have some room for improvement in fully replicating natural scenes.
However, Lightroom's AI models have also made strides in color analysis, leading to better color matching during repairs. Maintaining the integrity of the original image colors is crucial when healing, and these enhancements ensure a more accurate outcome. Interestingly, they've also incorporated depth of field into the healing process. This means users can repair images while maintaining the original focus and blur dynamics, lending a more realistic and nuanced appearance to the final result. While not perfect, the evolution of AI in Lightroom's healing brush is an ongoing process that's slowly pushing the boundaries of what's possible in digital image manipulation.
The Evolution of AI-Powered Healing Brushes in Image Editing Software 2020-2024 - Generative AI in Photoshop aims to improve adjustment brush versatility
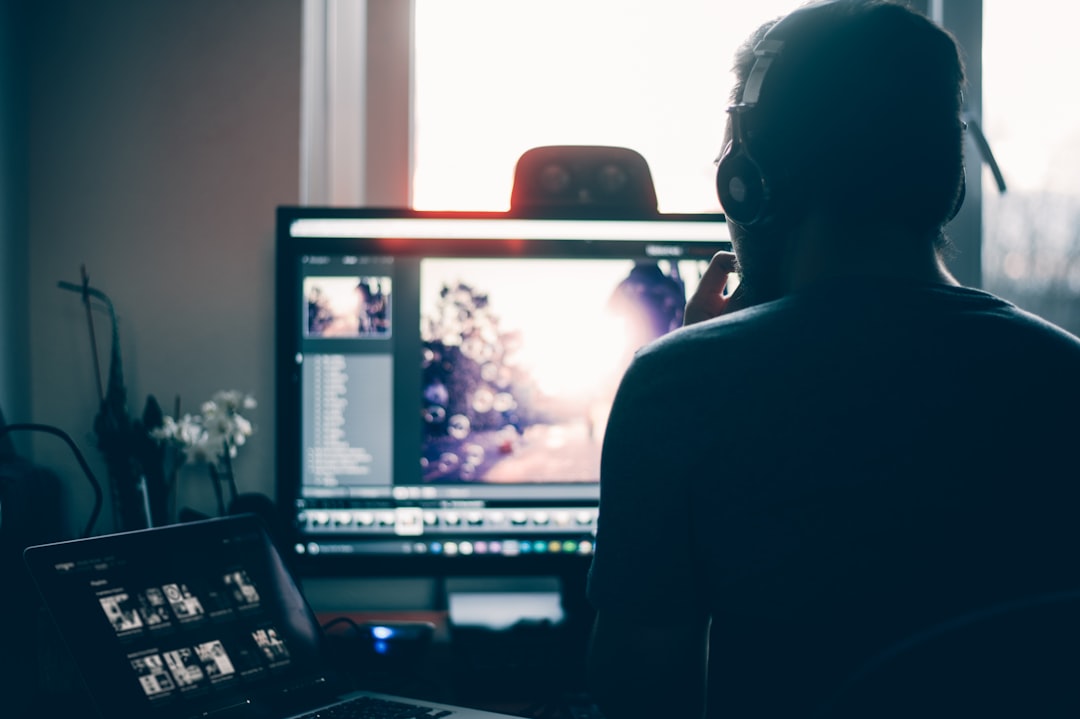
Photoshop's integration of generative AI has notably boosted the adjustment brush's capabilities, pushing the boundaries of what's possible in image editing. The inclusion of features like automatic selection makes it easier to fine-tune edits without extensive manual adjustments, leading to a more streamlined workflow. This improvement allows users to manipulate image elements with greater precision, such as effortlessly altering a background color by selecting specific objects. Underlying this is Adobe's Firefly technology, which enhances the brush's ability to seamlessly integrate edits into the surrounding image context. This focus on enhancing the user experience suggests a wider trend towards more intuitive yet advanced editing tools. It's clear that generative AI has the potential to simplify complex editing tasks, making photo editing more accessible to a broader range of users while also providing seasoned professionals with more powerful tools. However, the effectiveness of these new features may depend on the complexity of the image itself, and occasional manual adjustments might still be needed for optimal results.
Photoshop's integration of generative AI has notably enhanced the adjustment brush, pushing beyond its traditional pixel-based approach. It now leverages AI to analyze not just the immediate pixels, but also the broader image context. This allows for more intuitive edits, particularly when dealing with intricate textures or subtle color shifts. The changes are particularly evident in how the tool interacts with objects. We can now selectively target areas for adjustment based on the detected objects or elements, simplifying the process of applying changes to specific areas.
The shift to AI-powered selection has also sped up the editing workflow. Previously, precise adjustments could be time-consuming, requiring careful masking and manual refinement. With real-time previews enabled by the AI, it is much easier to see how the changes will appear in the final image, fostering a more iterative process. We can visualize edits in an instant rather than waiting for the software to process each alteration. The real-time feedback loop is quite useful for those wanting to fine-tune edits on-the-fly.
Furthermore, the AI seems to be better at understanding the overall color and texture harmony of the image. This is quite helpful in preventing edits from creating jarring discontinuities, a problem frequently encountered when manually adjusting images. The tool now seems better at predicting how the chosen adjustment will affect the neighboring areas, leading to smoother transitions and less need for manual blending. This is impressive, particularly in cases where we might need to modify lighting or color gradients.
Interestingly, this capability stems from a learning loop embedded within the algorithm. As users make adjustments, the model collects data, continually refining its understanding of how users apply edits. This means the adjustment brush should, in theory, improve over time as it gathers more data and learns to anticipate editing choices. It's unclear how successful this feedback loop will be, but the potential is definitely exciting, even if the current iteration is not fully realized.
However, the generative capabilities are primarily seen in background color changes. We can now isolate specific objects and change the background hue with remarkable precision. It’s possible that future iterations will push this boundary further, but it's still relatively limited in scope, especially when it comes to more intricate edits beyond changing color.
A key area where the brush now shines is handling previously challenging complex patterns, especially with high-frequency textures. It appears that the AI can now better interpret intricate details and provide more appropriate adjustments compared to older, simpler algorithms. Whether this applies equally across all image types, particularly in portraiture, will require further observation.
AI-driven depth perception has also started to play a role. When making an adjustment, the brush can now take the layering in an image into consideration, leading to more realistic and nuanced edits. This is especially important in situations where we're working with subjects positioned in front of a contrasting background. It's a promising new area that has the potential to enhance image manipulation.
The implementation of multiple adjustment layers provides additional flexibility in applying adjustments. Users can now work with different layers independently, and blend them together seamlessly. It's possible that this will be increasingly useful as the capabilities of the AI are further explored in the coming months.
Overall, these changes within Photoshop mark a considerable improvement to the functionality and versatility of the adjustment brush. It's early days for these AI features, and we may see these capabilities expanded upon in the near future. We are at a nascent stage of understanding how to leverage these new possibilities, but the direction is certainly promising.
Colorize and Breathe Life into Old Black-and-White Photos (Get started for free)
More Posts from colorizethis.io: