Colorize and Breathe Life into Old Black-and-White Photos (Get started for free)
The Evolution of AI-Powered Image Manipulation From Pixel Tweaks to Neural Network Transformations
The Evolution of AI-Powered Image Manipulation From Pixel Tweaks to Neural Network Transformations - Early Days Pixel-Level Adjustments in Digital Image Editing
The initial foray into digital image editing was characterized by manipulating images at the pixel level. These early days saw users wielding tools to perform fundamental adjustments like cropping unwanted areas, retouching imperfections, and fine-tuning color variations. While these early methods were relatively rudimentary compared to modern techniques, they laid the groundwork for the complex image editing capabilities we now have. This foundational era significantly impacted various fields, raising critical questions about responsible and ethical use of image manipulation. As software matured and became more user-friendly, the ability to manipulate images at the pixel level became increasingly accessible and vital for creative expression. This process spurred a fundamental shift that eventually paved the way for the current era of AI-driven image editing. The transition from basic pixel-level adjustments to sophisticated algorithms exemplifies the immense potential and inherent challenges that accompany the evolving landscape of image manipulation in our digitally saturated world.
The dawn of digital image editing was marked by a stark reality: the limitations of 8-bit per channel images. This meant a palette of just 256 shades for each color component, severely restricting the depth and subtlety of achievable visuals. The groundwork for these pixel-level manipulations was laid in early computer graphics research, with rudimentary systems experimenting with basic algorithms to alter contrast and brightness by directly modifying pixel values.
Adobe Photoshop's commercial debut in 1988 signaled the start of a new era, yet its early iterations were a far cry from the sophisticated tools we use today. The user experience was largely manual, demanding meticulous selection and painstaking adjustment procedures. Furthermore, a basic understanding of color theory, particularly the RGB color model, was essential for effective pixel manipulation. It wasn't always user-friendly, requiring a grasp of how colors interacted to achieve desired results.
Prior to widespread software, manipulating digital images involved a painstakingly tedious process: directly modifying raw pixel data through hex code. This approach, while offering the most granular control, was highly prone to errors. However, the invention of layers in image editing software marked a turning point. It enabled non-destructive adjustments, giving artists the freedom to experiment without jeopardizing the original image. This proved revolutionary for creative workflows.
Early digital image manipulation also had a significant performance constraint—it was computationally demanding. This meant that editing high-resolution or large images could be sluggish or impossible with the limited processing power available at the time. The interactive nature of adjusting pixel values through sliders, a concept borrowed from audio engineering, became a standard feature in the late 1990s, allowing for finer control.
Early image formats, like GIF and BMP, further highlighted the challenge of pixel-level manipulation, with their limited color depth. Artists needed to cleverly manipulate pixels to generate convincing color gradations and variations.
Despite remarkable advancements, the skill of manual pixel manipulation hasn't faded into obscurity. Professionals still often rely on these fundamental techniques, indicating that this traditional approach to control over image output retains its value in the modern digital environment. It highlights the enduring importance of understanding the foundational principles of image editing, even as AI-powered tools continue to develop.
The Evolution of AI-Powered Image Manipulation From Pixel Tweaks to Neural Network Transformations - Rise of Machine Learning Algorithms for Advanced Photo Manipulation
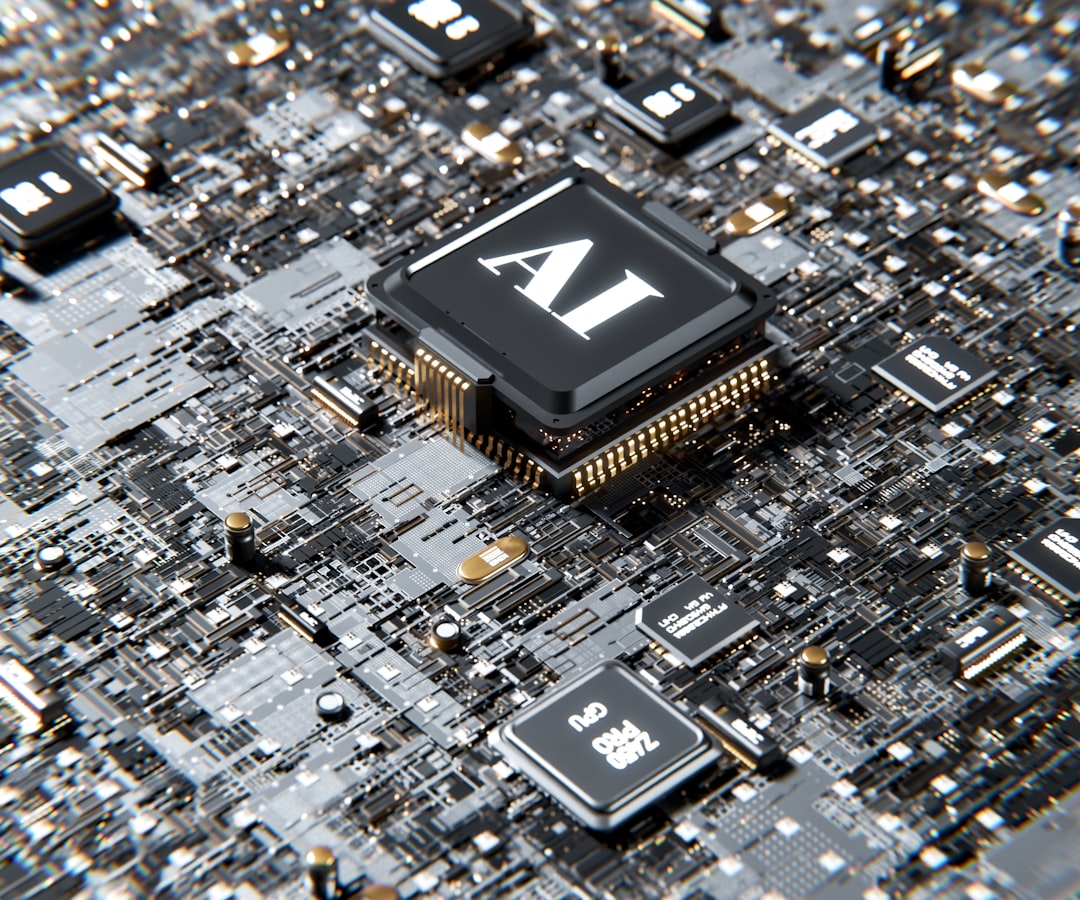
The integration of machine learning algorithms has ushered in a new era of advanced photo manipulation. Sophisticated models, such as generative adversarial networks (GANs), have empowered AI to perform highly complex image alterations exceeding the scope of traditional editing methods. This evolution allows AI to understand and interpret text-based descriptions, generating intricate visuals and empowering new creative possibilities. These developments, while offering incredible potential for artists and designers, also introduce challenges like discerning between authentic and manipulated images. Questions surrounding authenticity and ethical use of these powerful tools are critical in this evolving landscape. The advancements in AI-driven image manipulation not only expand the boundaries of what's achievable but also heighten the awareness of the responsibilities associated with these capabilities. It's a pivotal moment where the technology's potential clashes with the need for responsible implementation, forcing a critical examination of how we navigate this new reality of image creation and dissemination.
The field of machine learning has propelled photo manipulation to new heights. Early methods relied heavily on manual user input and specialized knowledge, but modern algorithms can now reconstruct images with impressive detail using minimal input. One significant breakthrough is Generative Adversarial Networks (GANs). These systems involve two neural networks that compete against each other, ultimately producing remarkably lifelike images that blend seamlessly with real photographs.
Deep learning's application to image editing has resulted in real-time enhancements, a feat unimaginable in the early days of pixel manipulation. This real-time capability has made image editing a significantly more fluid and responsive process. Intriguingly, researchers are discovering ways to use advanced algorithms to identify and even mitigate biases in image portrayal, presenting a new set of ethical considerations surrounding automated editing choices.
Neural style transfer, made possible by machine learning, allows users to infuse the style of one image onto another. This ability to seamlessly blend aesthetics and creative expression highlights a capability unattainable with traditional photo editing methods. Further, modern editing software can now anticipate user preferences based on previous editing history, suggesting edits that align with individual artistic styles, creating a personalized editing experience.
The power of machine learning extends beyond still images, revolutionizing video editing as well. Algorithms can now track and adjust objects across multiple frames with a level of precision and consistency that’s simply impossible to achieve with manual editing. Some advanced algorithms learn from user feedback, adapting their performance to foster a more intuitive and responsive editing experience – a major departure from the rigid tools of the past that didn't evolve with user interaction.
Furthermore, recent breakthroughs in contrast adjustment have demonstrated that dynamic algorithms can optimize image lighting based on real-time content analysis. This level of contextual awareness and adaptability is groundbreaking. However, while machine learning democratizes access to powerful image editing tools, it also sparks debates surrounding authenticity and copyright. Some algorithms can produce entirely original images without any human input, challenging conventional notions of artistic creation and ownership. This intriguing development underscores the evolving relationship between humans and artificial intelligence in creative endeavors.
The Evolution of AI-Powered Image Manipulation From Pixel Tweaks to Neural Network Transformations - Introduction of Generative Adversarial Networks in Image Creation
The introduction of Generative Adversarial Networks (GANs) in 2014 marked a pivotal moment in AI-powered image creation. GANs involve a unique approach where two neural networks—a generator and a discriminator—engage in a sort of ongoing competition. The generator attempts to produce realistic images, while the discriminator acts as a critic, evaluating the generated images for authenticity. This continuous feedback loop between the two networks drives the generator to refine its output, leading to increasingly realistic results.
Initially, GANs demonstrated their prowess in producing human faces with a degree of realism that was previously unattainable. This early success hinted at the vast potential of GANs for image generation and enhancement. Over time, GANs have expanded their capabilities, becoming capable of generating high-resolution, photorealistic images and even videos. This has been crucial in addressing persistent challenges in artificial intelligence, particularly in fields like computer vision, where the ability to create realistic synthetic data is highly valuable.
However, the development and deployment of GANs aren't without challenges. Their complex architecture and the intricacies of training them can make GANs difficult to implement in practice. Furthermore, as the line between original and synthetic images becomes increasingly blurred, critical conversations about the ethical considerations of using GANs have become necessary. In the artistic and creative communities, particularly, the use of GANs raises questions regarding the implications for originality, authorship, and the potential for misuse. As AI-generated imagery becomes increasingly sophisticated, navigating these ethical concerns is crucial for responsible technological advancement.
Generative Adversarial Networks (GANs), introduced in 2014 by Ian Goodfellow and his colleagues, represent a significant advancement in AI-driven image generation. GANs are built around a fascinating interplay between two neural networks: a generator, tasked with creating new images, and a discriminator, responsible for evaluating their authenticity. This dynamic duo engages in a sort of ongoing game, with the generator continuously trying to trick the discriminator into believing its outputs are real, while the discriminator refines its ability to distinguish between generated and genuine images. This adversarial process, effectively a constant back-and-forth, leads to increasingly refined image generation capabilities.
One of the remarkable achievements of GANs lies in their ability to create highly realistic images, often indistinguishable from real photographs. The quality of the output, reaching impressive resolutions like 1024x1024 pixels, showcases a significant improvement over prior image synthesis methods. The architecture of GANs has undergone constant refinement since its inception, with innovations such as Progressive Growing GANs and StyleGAN, which incorporated multi-scale synthesis and enhanced style transfer functionalities, continuing to push the boundaries of image generation.
While the artistic and entertainment industries have seen substantial benefits from GANs, their applicability extends beyond aesthetics. For example, in medicine, GANs are being used to generate high-quality images for training datasets, potentially revolutionizing diagnostic AI without the need for compromising patient data privacy. Another intriguing application involves addressing biases in datasets. GANs can generate synthetic images that include a more diverse range of demographic features, which is crucial for ensuring fair and equitable performance of machine learning models.
However, the journey with GANs hasn't been without its hurdles. Mode collapse, a tendency for the generator to produce only a limited set of output variations, remains a significant challenge. Researchers are actively working on methods to mitigate this issue, seeking to increase the diversity and novelty of images generated. Moreover, the powerful ability of GANs to generate images also necessitates careful consideration of ethical implications. Questions surrounding ownership, authorship, and the nature of consent, especially when GANs generate entirely novel content without direct human input, warrant ongoing debate.
GANs are not limited to static image generation, they can also generate animated images and even videos, delving into the temporal dimension of image creation. This capability expands their potential use across fields like film and gaming, where the possibility of real-time animation promises to enhance visual storytelling. While GANs' potential is vast, it's important to acknowledge their computational demands. Training these networks often requires significant resources, including powerful GPUs and substantial datasets. Researchers are persistently working to refine training techniques and make GANs accessible to a wider audience, including artists and researchers with limited computational resources.
The interplay between GANs and augmented reality (AR) is an exciting area of development. Imagine the potential for instantaneously generating realistic environments or objects that interact seamlessly with real-world elements. This convergence has the ability to drastically enhance spatial computing experiences and reshape the landscape of image manipulation. The rapid evolution of GANs in AI-powered image manipulation continues to reveal both remarkable potential and intriguing challenges. The capacity to create high-quality, novel images is transformative, but the need to address associated ethical dilemmas and computational limitations remains crucial to fostering a responsible and equitable landscape for this exciting technology.
The Evolution of AI-Powered Image Manipulation From Pixel Tweaks to Neural Network Transformations - Breakthrough of Neural Style Transfer Techniques
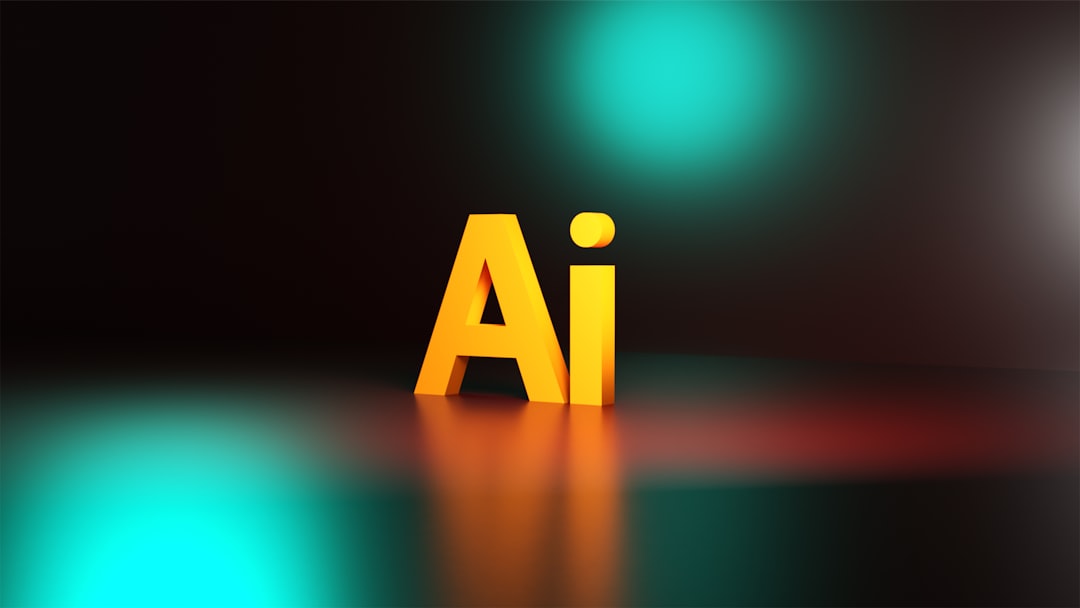
Neural style transfer (NST) emerged as a breakthrough in AI-powered image manipulation, leveraging convolutional neural networks (CNNs) to separate and recombine an image's content and style. Early research established the foundation for this technique, demonstrating its ability to transform images into various artistic styles using deep learning. Since then, NST has progressed significantly, allowing for the creation of exceptionally high-quality images and videos that blend photorealism with artistic elements. Moreover, researchers have developed approaches to optimize NST, resulting in faster and more precise transformations, expanding creative options for artists and users.
However, this surge in NST's capabilities also highlights ethical considerations regarding the nature of art produced by AI. Questions about authenticity, originality, and artistic ownership have become increasingly relevant as the line between human and machine-generated art blurs. The advancements in NST clearly mark a notable transition in how we view creativity and authorship in the context of evolving AI-powered image manipulation technologies. The ability to effortlessly blend artistic styles with photographic content pushes us to re-evaluate what constitutes authentic artistic expression in a world increasingly shaped by intelligent machines.
Neural Style Transfer (NST) utilizes convolutional neural networks (CNNs) to dissect and recombine the content and style of images, allowing for a fascinating artistic blending of two sources. This technique emerged from understanding that different layers within a CNN capture varying levels of image abstraction, providing a framework for this type of manipulation.
The initial impactful work on NST emerged in 2015, significantly influencing the fields of creative coding and digital art. This spurred a wave of adaptation within artistic communities, leading to experimental artworks that marry classical styles with modern imagery in intriguing ways.
Instead of manipulating individual pixel values like traditional editing software, NST works within a multi-dimensional space of features. This lets it capture stylistic elements at various levels of abstraction. This approach allows for a richer and more nuanced integration of texture and pattern than basic pixel methods could manage.
Improvements in computational efficiency have led to real-time NST, a huge advancement compared to its early stages that demanded significant processing time. This makes it feasible for artists to incorporate styles during live art or interactive installations, a captivating prospect.
Initially, NST primarily found application in still images, but it's expanded to different media such as videos and 3D rendering. This opens a new range of creative expression, allowing artists to explore dynamic styles that evolve over time.
NST has catalyzed collaborations between engineers, artists, and designers, ushering in innovative approaches that merge technology with artistic practice. These partnerships frequently lead to groundbreaking projects that redefine how we experience and interact with digital art.
The availability of user-friendly programs featuring NST has democratized advanced image manipulation. Tools like DeepArt and Prisma empower users without technical expertise to craft stylized images, expanding the audience for digital art.
Researchers are exploring how NST can support the creative process itself. By proposing styles that align with an artist's past work, these systems may transition from mere tools to collaborators, blurring the boundaries between human and artificial creative endeavors.
However, NST has also ignited discussions about authorship and originality in digital art. Its ability to replicate the style of prominent artists raises concerns about intellectual property and the ethics of replicating someone's artistic voice without consent.
Ongoing research on NST centers on enhancing style transfer fidelity while minimizing undesirable effects and inconsistencies in the output. These enhancements could unlock far more sophisticated applications, potentially altering the future of digital and interactive art.
The Evolution of AI-Powered Image Manipulation From Pixel Tweaks to Neural Network Transformations - Development of Text-to-Image AI Models like DALL-E and Midjourney
The emergence of text-to-image AI models like DALL-E and Midjourney signifies a remarkable advancement in AI's ability to understand and generate creative content. These systems leverage sophisticated deep learning techniques, notably GANs and diffusion models, to translate textual descriptions into visually compelling images. DALL-E, for example, has garnered significant recognition for its capability to translate abstract concepts and emotions into complex visual representations. Conversely, Midjourney focuses on artistic exploration, offering a wide range of stylistic options for users to experiment with. While these innovations democratize access to advanced image creation and hold vast potential for artistic endeavors, they concurrently spark critical conversations around ethical concerns. The blurring lines between human-generated and AI-created content raises complex questions regarding copyright, the nature of artistic originality, and the future of authorship. The continued advancement of text-to-image AI presents both exciting possibilities and a need for careful consideration of the societal implications of AI in creative fields.
The development of text-to-image AI models like DALL-E and Midjourney has been a fascinating journey, revealing both remarkable potential and unforeseen challenges. These systems rely on intricate language processing techniques, often utilizing transformer architectures, to go beyond simple keyword matching. They can understand context and create images that closely align with nuanced descriptions. The quality of their output hinges on the sheer volume and diversity of training data, with models like DALL-E benefiting from massive datasets of images paired with captions found on the internet.
Furthermore, many of these models use a multi-stage approach for generating images. They start with rough approximations and iteratively refine them based on user feedback or further computations, resulting in increasingly refined visuals. However, even with impressive advancements, these models have their limitations. They can still struggle to create scenes that require a deep understanding of spatial relationships between objects, sometimes leading to unrealistic or unexpected compositions.
Researchers are continuously striving to enhance these models by incorporating techniques like reinforcement learning that incorporate human feedback. This enables the models to learn from user preferences and further improve the quality of their outputs. Interestingly, the resolution of AI-generated images has dramatically increased. Earlier models often produced lower-resolution images, but now, they can create outputs exceeding 1024x1024 pixels, opening doors to diverse applications in areas like design and advertising where clarity and detail are paramount.
Yet, this incredible technology also brings about significant ethical concerns. The capacity to produce convincingly realistic images raises questions about authenticity and ownership. Instances of AI-generated art being confused with real photography highlight the evolving concept of authorship and the potential for misuse in the realm of disinformation.
Bias in training data remains a critical issue, potentially leading to cultural misrepresentations or the reinforcement of harmful stereotypes, particularly when AI is tasked with depicting diverse populations. Researchers need to be vigilant in curating training data to mitigate this risk. Excitingly, some newer text-to-image models are experimenting with multi-modal inputs, blending text and images to improve context awareness and generate more accurate visuals based on combined cues.
Ultimately, the influence of text-to-image AI extends far beyond the realms of art and creativity. Industries such as gaming, architecture, and education are finding innovative ways to leverage these technologies. They are being used for rapid prototyping, generating immersive training simulations, and even creating personalized content. This widespread potential across diverse sectors highlights the transformative capacity of this rapidly evolving technology.
The Evolution of AI-Powered Image Manipulation From Pixel Tweaks to Neural Network Transformations - Future Frontiers Real-Time Video Manipulation and 3D Scene Generation
The frontier of AI-powered video manipulation and 3D scene generation is rapidly expanding, fueled by breakthroughs in generative AI. We're seeing the emergence of real-time capabilities, where detailed 3D scenes can be generated on the fly, potentially revolutionizing fields like gaming, filmmaking, and virtual environments. This ability to instantly create intricate landscapes and objects from scratch is a significant development. Furthermore, the application of text-conditional diffusion models allows AI to generate increasingly realistic and diverse video content based on simple text prompts, pushing the boundaries of video synthesis.
However, as these technologies advance, ethical dilemmas arise. The ease with which realistic video content can be generated and manipulated raises questions about authenticity and the potential for misuse. Determining what constitutes original or genuine video becomes increasingly challenging in an environment where AI can convincingly fabricate scenes or alter existing footage. The potential for generating 'deepfakes' or manipulating video to deceive, further highlights the need for thoughtful consideration of the societal impact of these tools. As we move forward, we must grapple with how to responsibly navigate the intersection of advanced AI, creative expression, and potential misapplication of these transformative capabilities.
The future of AI-powered video manipulation and 3D scene generation is rapidly evolving, revealing exciting possibilities and presenting novel challenges. We're seeing a shift towards real-time processing, where changes to video can happen instantly, thanks to powerful GPUs and optimized algorithms. This real-time capability enables seamless integration of digital elements with live video, opening avenues for live video filters and augmented reality overlays.
Furthermore, AI's ability to reconstruct 3D scenes from 2D videos using depth estimation and motion tracking is astounding. This high-fidelity 3D reconstruction has wide-ranging implications for fields like gaming, architecture, and training simulations, promising more immersive and interactive environments. GANs, initially focused on still images, are being adapted for video generation, raising questions regarding originality and the potential for AI to construct narratives without human intervention.
AI is getting increasingly sophisticated in its ability to understand the content of videos. Advanced models now automatically segment scenes, recognize objects, actions, and environments, which is crucial for developing intelligent editing tools that can adapt to the video's context. We also see a fascinating convergence of traditional video editing tools and machine learning. Features like automated color grading and intelligent cutting are being integrated, allowing editors to focus on creative tasks rather than tedious, repetitive adjustments.
User interfaces are evolving too. Intuitive interfaces are being developed using natural gestures and voice commands to control 3D scene manipulation, making video editing accessible to a wider range of users. And these systems are getting remarkably good at maintaining temporal coherence—meaning that the changes they make in a video frame smoothly and seamlessly transition to the following frames, resulting in a more polished and visually appealing output.
However, we must be mindful that the power of AI video manipulation can also introduce biases. Similar to still image generation, training data issues can lead to skewed or inaccurate representations of certain demographics within generated videos, raising ethical concerns related to authenticity and representation.
Pushing the boundaries of creativity, researchers are finding ways to blend static images into moving video. Using image extrapolation, it's becoming possible to generate motion for still images, opening up new avenues for storytelling and advertisement. And in the future, we can anticipate increasingly personalized video editing experiences. Software could learn from an editor's past behavior, providing customized suggestions and tailoring the editing process to match their individual preferences. This user-centric approach would streamline workflows and further enhance the creative potential of AI in video manipulation.
The future frontiers of real-time video manipulation and 3D scene generation are both fascinating and complex. As the technology progresses, we must consider the ethical implications alongside the incredible opportunities it provides for creativity and innovation.
Colorize and Breathe Life into Old Black-and-White Photos (Get started for free)
More Posts from colorizethis.io: